Python orientato agli oggetti - Guida rapida
I linguaggi di programmazione stanno emergendo costantemente, così come diverse metodologie. La programmazione orientata agli oggetti è una di queste metodologie che è diventata piuttosto popolare negli ultimi anni.
Questo capitolo parla delle caratteristiche del linguaggio di programmazione Python che lo rende un linguaggio di programmazione orientato agli oggetti.
Schema di classificazione della programmazione del linguaggio
Python può essere caratterizzato con metodologie di programmazione orientate agli oggetti. L'immagine seguente mostra le caratteristiche di vari linguaggi di programmazione. Osserva le caratteristiche di Python che lo rendono orientato agli oggetti.
Corsi di lingua | Categorie | Langauages |
---|---|---|
Paradigma di programmazione | Procedurale | C, C ++, C #, Objective-C, java, Go |
Scripting | CoffeeScript, JavaScript, Python, Perl, Php, Ruby | |
Funzionale | Clojure, Eralang, Haskell, Scala | |
Classe di compilazione | Statico | C, C ++, C #, Objective-C, java, Go, Haskell, Scala |
Dinamico | CoffeeScript, JavaScript, Python, Perl, Php, Ruby, Clojure, Erlang | |
Tipo Classe | Forte | C #, java, Go, Python, Ruby, Clojure, Erlang, Haskell, Scala |
Debole | C, C ++, C #, Objective-C, CoffeeScript, JavaScript, Perl, Php | |
Classe di memoria | Gestito | Altri |
Non gestito | C, C ++, C #, Objective-C |
Che cos'è la programmazione orientata agli oggetti?
Object Orientedmezzi diretti verso gli oggetti. In altre parole, significa funzionalmente diretto verso oggetti di modellazione. Questa è una delle tante tecniche utilizzate per modellare sistemi complessi descrivendo una raccolta di oggetti interagenti tramite i loro dati e comportamento.
Python, una programmazione orientata agli oggetti (OOP), è un modo di programmare che si concentra sull'utilizzo di oggetti e classi per progettare e costruire applicazioni. Inheritance, Polymorphism, Abstraction, anno Domini Encapsulation.
L'analisi orientata agli oggetti (OOA) è il processo di esame di un problema, sistema o attività e di identificazione degli oggetti e delle interazioni tra di essi.
Perché scegliere la programmazione orientata agli oggetti?
Python è stato progettato con un approccio orientato agli oggetti. OOP offre i seguenti vantaggi:
Fornisce una chiara struttura del programma, che semplifica la mappatura dei problemi del mondo reale e delle relative soluzioni.
Facilita la facile manutenzione e modifica del codice esistente.
Migliora la modularità del programma perché ogni oggetto esiste indipendentemente e le nuove funzionalità possono essere aggiunte facilmente senza disturbare quelle esistenti.
Presenta una buona struttura per le librerie di codici in cui i componenti forniti possono essere facilmente adattati e modificati dal programmatore.
Fornisce la riusabilità del codice
Programmazione procedurale e orientata agli oggetti
La programmazione basata su procedure deriva dalla programmazione strutturale basata sui concetti di functions/procedure/routines. È facile accedere e modificare i dati nella programmazione procedurale orientata. D'altra parte, la programmazione orientata agli oggetti (OOP) consente la scomposizione di un problema in un numero di unità chiamateobjectse quindi creare i dati e le funzioni attorno a questi oggetti. Dà più importanza ai dati che alla procedura o alle funzioni. Anche in OOP, i dati sono nascosti e non è possibile accedervi da una procedura esterna.
La tabella nell'immagine seguente mostra le principali differenze tra l'approccio POP e OOP.
Differenza tra POP (Procedural Oriented Programming) vs. Programmazione orientata agli oggetti (OOP).
Programmazione procedurale orientata | Programmazione orientata agli oggetti | |
---|---|---|
Basato su | In Pop, tutta l'attenzione è rivolta ai dati e alle funzioni | Oops si basa su uno scenario del mondo reale. L'intero programma è diviso in piccole parti chiamate oggetto |
Riusabilità | Riutilizzo del codice limitato | Riutilizzo del codice |
Approccio | Approccio dall 'alto verso il basso | Design incentrato sugli oggetti |
Identificatori di accesso | Nemmeno uno | Pubblico, privato e protetto |
Spostamento dei dati | I dati possono spostarsi liberamente dalle funzioni alle funzioni nel sistema | In Oops, i dati possono spostarsi e comunicare tra loro tramite le funzioni membro |
Accesso ai dati | In pop, la maggior parte delle funzioni utilizza dati globali per la condivisione a cui è possibile accedere liberamente da una funzione all'altra nel sistema | In Oops, i dati non possono spostarsi liberamente da un metodo all'altro, possono essere mantenuti in pubblico o privato in modo da poter controllare l'accesso ai dati |
Dati nascosti | In pop, modo così specifico per nascondere i dati, quindi un po 'meno sicuro | Fornisce l'occultamento dei dati, molto più sicuro |
Sovraccarico | Non possibile | Funzioni e sovraccarico dell'operatore |
Linguaggi di esempio | C, VB, Fortran, Pascal | C ++, Python, Java, C # |
Astrazione | Utilizza l'astrazione a livello di procedura | Utilizza l'astrazione a livello di classe e oggetto |
Principi di programmazione orientata agli oggetti
La programmazione orientata agli oggetti (OOP) si basa sul concetto di objects piuttosto che azioni, e datapiuttosto che logica. Affinché un linguaggio di programmazione sia orientato agli oggetti, dovrebbe avere un meccanismo che consenta di lavorare con classi e oggetti, nonché l'implementazione e l'utilizzo dei principi e dei concetti fondamentali orientati agli oggetti, ovvero ereditarietà, astrazione, incapsulamento e polimorfismo.

Cerchiamo di capire in breve ciascuno dei pilastri della programmazione orientata agli oggetti:
Incapsulamento
Questa proprietà nasconde dettagli non necessari e semplifica la gestione della struttura del programma. L'implementazione e lo stato di ogni oggetto sono nascosti dietro confini ben definiti e ciò fornisce un'interfaccia pulita e semplice per lavorare con essi. Un modo per ottenere ciò è rendere privati i dati.
Eredità
L'ereditarietà, chiamata anche generalizzazione, ci consente di catturare una relazione gerarchica tra classi e oggetti. Ad esempio, un "frutto" è una generalizzazione di "arancia". L'ereditarietà è molto utile dal punto di vista del riutilizzo del codice.
Astrazione
Questa proprietà ci consente di nascondere i dettagli ed esporre solo le caratteristiche essenziali di un concetto o di un oggetto. Ad esempio, una persona alla guida di uno scooter sa che premendo un clacson viene emesso un suono, ma non ha idea di come il suono venga effettivamente generato premendo il clacson.
Polimorfismo
Poly-morfismo significa molte forme. Cioè, una cosa o un'azione è presente in diverse forme o modi. Un buon esempio di polimorfismo è il sovraccarico del costruttore nelle classi.
Python orientato agli oggetti
Il cuore della programmazione Python è object e OOP, tuttavia non è necessario limitarsi a utilizzare l'OOP organizzando il codice in classi. L'OOP si aggiunge all'intera filosofia di progettazione di Python e incoraggia un modo pulito e pragmatico di programmazione. OOP consente anche di scrivere programmi più grandi e complessi.
Moduli vs classi e oggetti
I moduli sono come i "dizionari"
Quando si lavora sui moduli, tenere presente i seguenti punti:
Un modulo Python è un pacchetto per incapsulare codice riutilizzabile.
I moduli risiedono in una cartella con estensione __init__.py file su di esso.
I moduli contengono funzioni e classi.
I moduli vengono importati utilizzando il file import parola chiave.
Ricorda che un dizionario è un file key-valuepaio. Ciò significa che se hai un dizionario con una chiaveEmployeID e vuoi recuperarlo, dovrai usare le seguenti righe di codice:
employee = {“EmployeID”: “Employee Unique Identity!”}
print (employee [‘EmployeID])
Dovrai lavorare sui moduli con il seguente processo:
Un modulo è un file Python con alcune funzioni o variabili al suo interno.
Importa il file che ti serve.
Ora puoi accedere alle funzioni o alle variabili in quel modulo con "." (dot) Operatore.
Considera un modulo denominato employee.py con una funzione in esso chiamata employee. Di seguito il codice della funzione:
# this goes in employee.py
def EmployeID():
print (“Employee Unique Identity!”)
Ora importa il modulo e quindi accedi alla funzione EmployeID -
import employee
employee. EmployeID()
Puoi inserire una variabile in essa denominata Age, come mostrato -
def EmployeID():
print (“Employee Unique Identity!”)
# just a variable
Age = “Employee age is **”
Ora accedi a quella variabile nel modo seguente:
import employee
employee.EmployeID()
print(employee.Age)
Ora confrontiamolo con il dizionario -
Employee[‘EmployeID’] # get EmployeID from employee
Employee.employeID() # get employeID from the module
Employee.Age # get access to variable
Si noti che esiste un modello comune in Python -
Prendi un key = value contenitore di stile
Prendi qualcosa dal nome della chiave
Quando si confronta il modulo con un dizionario, entrambi sono simili, tranne per quanto segue:
Nel caso di dictionary, la chiave è una stringa e la sintassi è [chiave].
Nel caso di module, la chiave è un identificatore e la sintassi è .key.
Le classi sono come i moduli
Il modulo è un dizionario specializzato che può memorizzare codice Python in modo che tu possa accedervi con il '.' Operatore. Una classe è un modo per prendere un raggruppamento di funzioni e dati e inserirli in un contenitore in modo da potervi accedere con l'operatore ".".
Se devi creare una classe simile al modulo dipendente, puoi farlo utilizzando il codice seguente:
class employee(object):
def __init__(self):
self. Age = “Employee Age is ##”
def EmployeID(self):
print (“This is just employee unique identity”)
Note- Le classi sono preferite rispetto ai moduli perché puoi riutilizzarle così come sono e senza troppe interferenze. Mentre con i moduli, ne hai solo uno con l'intero programma.
Gli oggetti sono come le mini-importazioni
Una classe è come un file mini-module e puoi importare in modo simile a come fai per le classi, usando il concetto chiamato instantiate. Tieni presente che quando crei un'istanza di una classe, ottieni un fileobject.
Puoi istanziare un oggetto, in modo simile alla chiamata di una classe come una funzione, come mostrato -
this_obj = employee() # Instantiatethis_obj.EmployeID() # get EmployeId from the class
print(this_obj.Age) # get variable Age
Puoi farlo in uno dei seguenti tre modi:
# dictionary style
Employee[‘EmployeID’]
# module style
Employee.EmployeID()
Print(employee.Age)
# Class style
this_obj = employee()
this_obj.employeID()
Print(this_obj.Age)
Questo capitolo spiegherà in dettaglio come configurare l'ambiente Python sul tuo computer locale.
Prerequisiti e toolkit
Prima di procedere con l'apprendimento ulteriore su Python, ti suggeriamo di verificare se i seguenti prerequisiti sono soddisfatti:
L'ultima versione di Python è installata sul tuo computer
È installato un IDE o un editor di testo
Hai familiarità di base per scrivere ed eseguire il debug in Python, ovvero puoi eseguire le seguenti operazioni in Python:
In grado di scrivere ed eseguire programmi Python.
Eseguire il debug dei programmi e diagnosticare gli errori.
Lavora con i tipi di dati di base.
Scrivi for loop, while loop e if dichiarazioni
Codice functions
Se non hai esperienza con i linguaggi di programmazione, puoi trovare molti tutorial per principianti in Python su
https://www.tutorialpoints.com/Installazione di Python
I seguenti passaggi mostrano in dettaglio come installare Python sul tuo computer locale -
Step 1 - Vai al sito web ufficiale di Python https://www.python.org/, clicca sul Downloads menu e scegli l'ultima o qualsiasi versione stabile di tua scelta.

Step 2- Salva il file exe del programma di installazione Python che stai scaricando e una volta scaricato, aprilo. Clicca suRun e scegli Next opzione predefinita e completare l'installazione.

Step 3- Dopo aver installato, dovresti ora vedere il menu Python come mostrato nell'immagine qui sotto. Avvia il programma scegliendo IDLE (Python GUI).

Questo avvierà la shell Python. Digita semplici comandi per controllare l'installazione.

Scegliere un IDE
Un ambiente di sviluppo integrato è un editor di testo orientato allo sviluppo del software. Dovrai installare un IDE per controllare il flusso della tua programmazione e per raggruppare i progetti quando lavori su Python. Ecco alcuni degli IDE disponibili online. Puoi sceglierne uno a tuo piacimento.
- Pycharm IDE
- Komodo IDE
- IDE Eric Python
Note - Eclipse IDE è utilizzato principalmente in Java, tuttavia ha un plugin Python.
Pycharm

Pycharm, l'IDE multipiattaforma è uno degli IDE più popolari attualmente disponibili. Fornisce assistenza e analisi per la codifica con completamento del codice, navigazione del progetto e del codice, test di unità integrati, integrazione del controllo della versione, debug e molto altro
Link per scaricare
https://www.jetbrains.com/pycharm/download/#section=windowsLanguages Supported - Python, HTML, CSS, JavaScript, Coffee Script, TypeScript, Cython, AngularJS, Node.js, linguaggi per modelli.
Immagine dello schermo

Perché scegliere?
PyCharm offre le seguenti caratteristiche e vantaggi per i suoi utenti:
- IDE multipiattaforma compatibile con Windows, Linux e Mac OS
- Include l'IDE Django, oltre al supporto per CSS e JavaScript
- Include migliaia di plugin, terminale integrato e controllo della versione
- Si integra con Git, SVN e Mercurial
- Offre strumenti di modifica intelligenti per Python
- Facile integrazione con Virtualenv, Docker e Vagrant
- Semplici funzioni di navigazione e ricerca
- Analisi del codice e refactoring
- Iniezioni configurabili
- Supporta tonnellate di librerie Python
- Contiene modelli e debugger JavaScript
- Include debugger Python / Django
- Funziona con Google App Engine, framework e librerie aggiuntivi.
- Ha un'interfaccia utente personalizzabile, emulazione VIM disponibile
Komodo IDE

È un IDE poliglotta che supporta oltre 100 lingue e fondamentalmente per linguaggi dinamici come Python, PHP e Ruby. È un IDE commerciale disponibile per 21 giorni di prova gratuita con funzionalità complete. ActiveState è la società di software che gestisce lo sviluppo dell'IDE di Komodo. Offre anche una versione ridotta di Komodo nota come Komodo Edit per semplici attività di programmazione.
Questo IDE contiene tutti i tipi di funzionalità dal livello più semplice a quello avanzato. Se sei uno studente o un libero professionista, puoi acquistarlo quasi la metà del prezzo effettivo. Tuttavia, è completamente gratuito per insegnanti e professori di istituzioni e università riconosciute.
Ha tutte le funzionalità necessarie per lo sviluppo web e mobile, incluso il supporto per tutti i tuoi linguaggi e framework.
Link per scaricare
I link per il download per Komodo Edit (versione gratuita) e Komodo IDE (versione a pagamento) sono come forniti qui -
Komodo Edit (free)
https://www.activestate.com/komodo-editKomodo IDE (paid)
https://www.activestate.com/komodo-ide/downloads/ideImmagine dello schermo

Perché scegliere?
- IDE potente con supporto per Perl, PHP, Python, Ruby e molti altri.
- IDE multipiattaforma.
Include funzionalità di base come supporto del debugger integrato, completamento automatico, visualizzatore DOM (Document Object Model), browser del codice, shell interattive, configurazione del punto di interruzione, profilazione del codice, unit test integrato. In breve, è un IDE professionale con una serie di funzioni per aumentare la produttività.
IDE Eric Python

È un IDE open source per Python e Ruby. Eric è un editor e un IDE completo, scritto in Python. È basato sul toolkit Qt GUI multipiattaforma, che integra il controllo dell'editor Scintilla altamente flessibile. L'IDE è molto configurabile e si può scegliere cosa usare e cosa no. Puoi scaricare Eric IDE dal link sottostante:
https://eric-ide.python-projects.org/eric-download.htmlPerché scegliere
- Grande rientro, evidenziazione degli errori.
- Assistenza sul codice
- Completamento del codice
- Pulizia del codice con PyLint
- Ricerca rapida
- Debugger Python integrato.
Immagine dello schermo

Scegliere un editor di testo
Potrebbe non essere sempre necessario un IDE. Per attività come l'apprendimento del codice con Python o Arduino, o quando si lavora su uno script veloce in script di shell per aiutarti ad automatizzare alcune attività, un editor di testo incentrato sul codice semplice e leggero farà. Inoltre, molti editor di testo offrono funzionalità come l'evidenziazione della sintassi e l'esecuzione di script nel programma, simili agli IDE. Alcuni degli editor di testo sono forniti qui:
- Atom
- Testo sublime
- Notepad++
Atom Text Editor

Atom è un editor di testo hackerabile creato dal team di GitHub. È un editor di testo e codice gratuito e open source, il che significa che tutto il codice è disponibile per essere letto, modificato per uso personale e persino per apportare miglioramenti. È un editor di testo multipiattaforma compatibile per macOS, Linux e Microsoft Windows con supporto per plug-in scritti in Node.js e Git Control incorporato.
Link per scaricare
https://atom.io/Immagine dello schermo
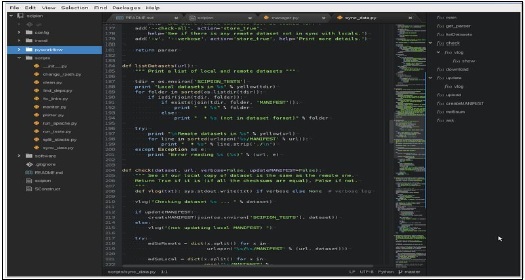
Lingue supportate
C / C ++, C #, CSS, CoffeeScript, HTML, JavaScript, Java, JSON, Julia, Objective-C, PHP, Perl, Python, Ruby on Rails, Ruby, Shell script, Scala, SQL, XML, YAML e molti altri.
Sublime Text Editor

Sublime text è un software proprietario e ti offre una versione di prova gratuita per provarlo prima di acquistarlo. Secondo stackoverflow.com , è il quarto ambiente di sviluppo più popolare.
Alcuni dei vantaggi che offre sono la sua incredibile velocità, facilità d'uso e supporto della comunità. Supporta anche molti linguaggi di programmazione e linguaggi di mark-up e le funzioni possono essere aggiunte dagli utenti con plug-in, in genere creati dalla comunità e mantenuti con licenze di software libero.
Immagine dello schermo

Lingua supportata
- Python, Ruby, JavaScript ecc.
Perché scegliere?
Personalizza associazioni di tasti, menu, snippet, macro, completamenti e altro ancora.
Funzione di completamento automatico
- Inserisci rapidamente testo e codice con frammenti di testo sublimi utilizzando frammenti, indicatori di campo e segnaposto
Si apre rapidamente
Supporto multipiattaforma per Mac, Linux e Windows.
Salta il cursore dove vuoi andare
Seleziona più righe, parole e colonne
Notepad ++

È un editor di codice sorgente gratuito e un sostituto del Blocco note che supporta diversi linguaggi da Assembly a XML e incluso Python. Funzionando nell'ambiente MS Windows, il suo utilizzo è regolato dalla licenza GPL. Oltre all'evidenziazione della sintassi, Notepad ++ ha alcune funzionalità particolarmente utili per i programmatori.
Immagine dello schermo

Caratteristiche principali
- Evidenziazione della sintassi e ripiegamento della sintassi
- PCRE (Espressione regolare compatibile con Perl) Cerca / sostituisci
- GUI completamente personalizzabile
- Completamento automatico
- Modifica a schede
- Multi-View
- Ambiente multilingue
- Lanciabile con argomenti diversi
Lingua supportata
- Quasi tutte le lingue (oltre 60 lingue) come Python, C, C ++, C #, Java ecc.
Le strutture dati Python sono molto intuitive dal punto di vista della sintassi e offrono un'ampia scelta di operazioni. È necessario scegliere la struttura dei dati Python a seconda di cosa comportano i dati, se devono essere modificati, o se si tratta di un dato fisso e di quale tipo di accesso è richiesto, come all'inizio / fine / casuale ecc.
Liste
Una lista rappresenta il tipo più versatile di struttura dati in Python. Un elenco è un contenitore che contiene valori separati da virgole (elementi o elementi) tra parentesi quadre. Gli elenchi sono utili quando vogliamo lavorare con più valori correlati. Poiché gli elenchi tengono insieme i dati, possiamo eseguire gli stessi metodi e operazioni su più valori contemporaneamente. Gli indici delle liste iniziano da zero e, a differenza delle stringhe, le liste sono mutabili.
Struttura dei dati - Elenco
>>>
>>> # Any Empty List
>>> empty_list = []
>>>
>>> # A list of String
>>> str_list = ['Life', 'Is', 'Beautiful']
>>> # A list of Integers
>>> int_list = [1, 4, 5, 9, 18]
>>>
>>> #Mixed items list
>>> mixed_list = ['This', 9, 'is', 18, 45.9, 'a', 54, 'mixed', 99, 'list']
>>> # To print the list
>>>
>>> print(empty_list)
[]
>>> print(str_list)
['Life', 'Is', 'Beautiful']
>>> print(type(str_list))
<class 'list'>
>>> print(int_list)
[1, 4, 5, 9, 18]
>>> print(mixed_list)
['This', 9, 'is', 18, 45.9, 'a', 54, 'mixed', 99, 'list']
Accesso agli elementi nell'elenco Python
Ad ogni elemento di una lista viene assegnato un numero, che è l'indice o la posizione di quel numero. L'indice inizia sempre da zero, il secondo indice è uno e così via. Per accedere agli elementi di un elenco, possiamo utilizzare questi numeri di indice all'interno di una parentesi quadra. Ad esempio, osservare il codice seguente:
>>> mixed_list = ['This', 9, 'is', 18, 45.9, 'a', 54, 'mixed', 99, 'list']
>>>
>>> # To access the First Item of the list
>>> mixed_list[0]
'This'
>>> # To access the 4th item
>>> mixed_list[3]
18
>>> # To access the last item of the list
>>> mixed_list[-1]
'list'
Oggetti vuoti
Gli oggetti vuoti sono i tipi integrati di Python più semplici e basilari. Li abbiamo usati più volte senza accorgercene e li abbiamo estesi a tutte le classi che abbiamo creato. Lo scopo principale di scrivere una classe vuota è bloccare qualcosa per il momento e successivamente estenderlo e aggiungervi un comportamento.
Aggiungere un comportamento a una classe significa sostituire una struttura dati con un oggetto e modificare tutti i riferimenti ad esso. Quindi è importante controllare i dati, se si tratta di un oggetto sotto mentite spoglie, prima di creare qualsiasi cosa. Osservare il codice seguente per una migliore comprensione:
>>> #Empty objects
>>>
>>> obj = object()
>>> obj.x = 9
Traceback (most recent call last):
File "<pyshell#3>", line 1, in <module>
obj.x = 9
AttributeError: 'object' object has no attribute 'x'
Quindi dall'alto, possiamo vedere che non è possibile impostare alcun attributo su un oggetto che è stato istanziato direttamente. Quando Python consente a un oggetto di avere attributi arbitrari, ci vuole una certa quantità di memoria di sistema per tenere traccia di quali attributi ha ogni oggetto, per memorizzare sia il nome dell'attributo che il suo valore. Anche se non vengono memorizzati attributi, una certa quantità di memoria viene allocata per potenziali nuovi attributi.
Quindi Python disabilita le proprietà arbitrarie sull'oggetto e molti altri integrati, per impostazione predefinita.
>>> # Empty Objects
>>>
>>> class EmpObject:
pass
>>> obj = EmpObject()
>>> obj.x = 'Hello, World!'
>>> obj.x
'Hello, World!'
Quindi, se vogliamo raggruppare le proprietà insieme, potremmo memorizzarle in un oggetto vuoto come mostrato nel codice sopra. Tuttavia, questo metodo non è sempre suggerito. Ricorda che le classi e gli oggetti dovrebbero essere usati solo quando vuoi specificare sia dati che comportamenti.
Tuple
Le tuple sono simili agli elenchi e possono memorizzare elementi. Tuttavia, sono immutabili, quindi non possiamo aggiungere, rimuovere o sostituire oggetti. Il vantaggio principale che la tupla fornisce a causa della sua immutabilità è che possiamo usarli come chiavi nei dizionari o in altre posizioni in cui un oggetto richiede un valore hash.
Le tuple vengono utilizzate per memorizzare i dati e non il comportamento. Nel caso in cui sia necessario un comportamento per manipolare una tupla, è necessario passare la tupla a una funzione (o metodo su un altro oggetto) che esegue l'azione.
Poiché la tupla può agire come una chiave del dizionario, i valori memorizzati sono diversi l'uno dall'altro. Possiamo creare una tupla separando i valori con una virgola. Le tuple sono racchiuse tra parentesi ma non obbligatorie. Il codice seguente mostra due assegnazioni identiche.
>>> stock1 = 'MSFT', 95.00, 97.45, 92.45
>>> stock2 = ('MSFT', 95.00, 97.45, 92.45)
>>> type (stock1)
<class 'tuple'>
>>> type(stock2)
<class 'tuple'>
>>> stock1 == stock2
True
>>>
Definizione di una tupla
Le tuple sono molto simili a list tranne per il fatto che l'intero insieme di elementi è racchiuso tra parentesi invece che tra parentesi quadre.
Proprio come quando si divide una lista, si ottiene una nuova lista e quando si divide una tupla, si ottiene una nuova tupla.
>>> tupl = ('Tuple','is', 'an','IMMUTABLE', 'list')
>>> tupl
('Tuple', 'is', 'an', 'IMMUTABLE', 'list')
>>> tupl[0]
'Tuple'
>>> tupl[-1]
'list'
>>> tupl[1:3]
('is', 'an')
Metodi di tupla Python
Il codice seguente mostra i metodi nelle tuple Python:
>>> tupl
('Tuple', 'is', 'an', 'IMMUTABLE', 'list')
>>> tupl.append('new')
Traceback (most recent call last):
File "<pyshell#148>", line 1, in <module>
tupl.append('new')
AttributeError: 'tuple' object has no attribute 'append'
>>> tupl.remove('is')
Traceback (most recent call last):
File "<pyshell#149>", line 1, in <module>
tupl.remove('is')
AttributeError: 'tuple' object has no attribute 'remove'
>>> tupl.index('list')
4
>>> tupl.index('new')
Traceback (most recent call last):
File "<pyshell#151>", line 1, in <module>
tupl.index('new')
ValueError: tuple.index(x): x not in tuple
>>> "is" in tupl
True
>>> tupl.count('is')
1
Dal codice mostrato sopra, possiamo capire che le tuple sono immutabili e quindi -
tu cannot aggiungere elementi a una tupla.
tu cannot aggiungere o estendere un metodo.
tu cannot rimuove gli elementi da una tupla.
Le tuple hanno no metodo di rimozione o pop.
Count e index sono i metodi disponibili in una tupla.
Dizionario
Dictionary è uno dei tipi di dati incorporati in Python e definisce le relazioni uno a uno tra chiavi e valori.
Definizione di dizionari
Osservare il codice seguente per comprendere come definire un dizionario:
>>> # empty dictionary
>>> my_dict = {}
>>>
>>> # dictionary with integer keys
>>> my_dict = { 1:'msft', 2: 'IT'}
>>>
>>> # dictionary with mixed keys
>>> my_dict = {'name': 'Aarav', 1: [ 2, 4, 10]}
>>>
>>> # using built-in function dict()
>>> my_dict = dict({1:'msft', 2:'IT'})
>>>
>>> # From sequence having each item as a pair
>>> my_dict = dict([(1,'msft'), (2,'IT')])
>>>
>>> # Accessing elements of a dictionary
>>> my_dict[1]
'msft'
>>> my_dict[2]
'IT'
>>> my_dict['IT']
Traceback (most recent call last):
File "<pyshell#177>", line 1, in <module>
my_dict['IT']
KeyError: 'IT'
>>>
Dal codice sopra possiamo osservare che:
Per prima cosa creiamo un dizionario con due elementi e lo assegniamo alla variabile my_dict. Ogni elemento è una coppia chiave-valore e l'intero set di elementi è racchiuso tra parentesi graffe.
Il numero 1 è la chiave e msftè il suo valore. Allo stesso modo,2 è la chiave e IT è il suo valore.
Puoi ottenere valori per chiave, ma non viceversa. Così quando ci proviamomy_dict[‘IT’] , solleva un'eccezione, perché IT non è una chiave.
Modifica dei dizionari
Osservare il codice seguente per comprendere come modificare un dizionario:
>>> # Modifying a Dictionary
>>>
>>> my_dict
{1: 'msft', 2: 'IT'}
>>> my_dict[2] = 'Software'
>>> my_dict
{1: 'msft', 2: 'Software'}
>>>
>>> my_dict[3] = 'Microsoft Technologies'
>>> my_dict
{1: 'msft', 2: 'Software', 3: 'Microsoft Technologies'}
Dal codice sopra possiamo osservare che -
Non puoi avere chiavi duplicate in un dizionario. La modifica del valore di una chiave esistente eliminerà il vecchio valore.
Puoi aggiungere nuove coppie chiave-valore in qualsiasi momento.
I dizionari non hanno il concetto di ordine tra gli elementi. Sono semplici raccolte non ordinate.
Combinazione di tipi di dati in un dizionario
Osservare il codice seguente per comprendere come combinare i tipi di dati in un dizionario:
>>> # Mixing Data Types in a Dictionary
>>>
>>> my_dict
{1: 'msft', 2: 'Software', 3: 'Microsoft Technologies'}
>>> my_dict[4] = 'Operating System'
>>> my_dict
{1: 'msft', 2: 'Software', 3: 'Microsoft Technologies', 4: 'Operating System'}
>>> my_dict['Bill Gates'] = 'Owner'
>>> my_dict
{1: 'msft', 2: 'Software', 3: 'Microsoft Technologies', 4: 'Operating System',
'Bill Gates': 'Owner'}
Dal codice sopra possiamo osservare che -
Non solo stringhe, ma il valore del dizionario può essere di qualsiasi tipo di dati, comprese stringhe, numeri interi, incluso il dizionario stesso.
A differenza dei valori del dizionario, le chiavi del dizionario sono più limitate, ma possono essere di qualsiasi tipo come stringhe, numeri interi o qualsiasi altro.
Eliminazione di elementi dai dizionari
Per informazioni sull'eliminazione di elementi da un dizionario, osservare il codice seguente:
>>> # Deleting Items from a Dictionary
>>>
>>> my_dict
{1: 'msft', 2: 'Software', 3: 'Microsoft Technologies', 4: 'Operating System',
'Bill Gates': 'Owner'}
>>>
>>> del my_dict['Bill Gates']
>>> my_dict
{1: 'msft', 2: 'Software', 3: 'Microsoft Technologies', 4: 'Operating System'}
>>>
>>> my_dict.clear()
>>> my_dict
{}
Dal codice sopra possiamo osservare che -
del - consente di eliminare singoli elementi da un dizionario tramite chiave.
clear - elimina tutti gli elementi da un dizionario.
Imposta
Set () è una raccolta non ordinata senza elementi duplicati. Sebbene i singoli elementi siano immutabili, l'insieme stesso è mutabile, ovvero possiamo aggiungere o rimuovere elementi / elementi dall'insieme. Possiamo eseguire operazioni matematiche come unione, intersezione ecc. Con set.
Sebbene i set in generale possano essere implementati usando gli alberi, i set in Python possono essere implementati usando una tabella hash. Ciò consente un metodo altamente ottimizzato per verificare se un elemento specifico è contenuto nel set
Creare un set
Un set viene creato inserendo tutti gli elementi (elementi) all'interno di parentesi graffe {}, separati da virgola o utilizzando la funzione incorporata set(). Rispettare le seguenti righe di codice:
>>> #set of integers
>>> my_set = {1,2,4,8}
>>> print(my_set)
{8, 1, 2, 4}
>>>
>>> #set of mixed datatypes
>>> my_set = {1.0, "Hello World!", (2, 4, 6)}
>>> print(my_set)
{1.0, (2, 4, 6), 'Hello World!'}
>>>
Metodi per insiemi
Osservare il codice seguente per comprendere i metodi per i set:
>>> >>> #METHODS FOR SETS
>>>
>>> #add(x) Method
>>> topics = {'Python', 'Java', 'C#'}
>>> topics.add('C++')
>>> topics
{'C#', 'C++', 'Java', 'Python'}
>>>
>>> #union(s) Method, returns a union of two set.
>>> topics
{'C#', 'C++', 'Java', 'Python'}
>>> team = {'Developer', 'Content Writer', 'Editor','Tester'}
>>> group = topics.union(team)
>>> group
{'Tester', 'C#', 'Python', 'Editor', 'Developer', 'C++', 'Java', 'Content
Writer'}
>>> # intersets(s) method, returns an intersection of two sets
>>> inters = topics.intersection(team)
>>> inters
set()
>>>
>>> # difference(s) Method, returns a set containing all the elements of
invoking set but not of the second set.
>>>
>>> safe = topics.difference(team)
>>> safe
{'Python', 'C++', 'Java', 'C#'}
>>>
>>> diff = topics.difference(group)
>>> diff
set()
>>> #clear() Method, Empties the whole set.
>>> group.clear()
>>> group
set()
>>>
Operatori per insiemi
Osservare il codice seguente per comprendere gli operatori per gli insiemi:
>>> # PYTHON SET OPERATIONS
>>>
>>> #Creating two sets
>>> set1 = set()
>>> set2 = set()
>>>
>>> # Adding elements to set
>>> for i in range(1,5):
set1.add(i)
>>> for j in range(4,9):
set2.add(j)
>>> set1
{1, 2, 3, 4}
>>> set2
{4, 5, 6, 7, 8}
>>>
>>> #Union of set1 and set2
>>> set3 = set1 | set2 # same as set1.union(set2)
>>> print('Union of set1 & set2: set3 = ', set3)
Union of set1 & set2: set3 = {1, 2, 3, 4, 5, 6, 7, 8}
>>>
>>> #Intersection of set1 & set2
>>> set4 = set1 & set2 # same as set1.intersection(set2)
>>> print('Intersection of set1 and set2: set4 = ', set4)
Intersection of set1 and set2: set4 = {4}
>>>
>>> # Checking relation between set3 and set4
>>> if set3 > set4: # set3.issuperset(set4)
print('Set3 is superset of set4')
elif set3 < set4: #set3.issubset(set4)
print('Set3 is subset of set4')
else: #set3 == set4
print('Set 3 is same as set4')
Set3 is superset of set4
>>>
>>> # Difference between set3 and set4
>>> set5 = set3 - set4
>>> print('Elements in set3 and not in set4: set5 = ', set5)
Elements in set3 and not in set4: set5 = {1, 2, 3, 5, 6, 7, 8}
>>>
>>> # Check if set4 and set5 are disjoint sets
>>> if set4.isdisjoint(set5):
print('Set4 and set5 have nothing in common\n')
Set4 and set5 have nothing in common
>>> # Removing all the values of set5
>>> set5.clear()
>>> set5 set()
In questo capitolo discuteremo in dettaglio i termini orientati agli oggetti e i concetti di programmazione. Class è solo una fabbrica per un'istanza. Questa fabbrica contiene il progetto che descrive come creare le istanze. Una istanza o un oggetto vengono costruiti dalla classe. Nella maggior parte dei casi, possiamo avere più di un'istanza di una classe. Ogni istanza ha un insieme di attributi e questi attributi sono definiti in una classe, quindi ogni istanza di una particolare classe dovrebbe avere gli stessi attributi.
Pacchetti di classi: comportamento e stato
Una classe ti permetterà di raggruppare insieme il comportamento e lo stato di un oggetto. Osservare il diagramma seguente per una migliore comprensione:
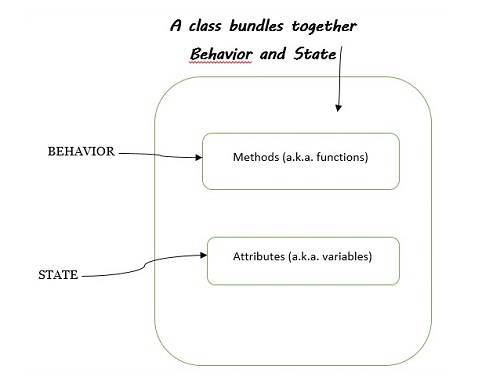
I seguenti punti sono degni di nota quando si discute di pacchetti di classi:
La parola behavior è identico a function - è un pezzo di codice che fa qualcosa (o implementa un comportamento)
La parola state è identico a variables - è un luogo in cui memorizzare i valori all'interno di una classe.
Quando affermiamo insieme un comportamento e uno stato di una classe, significa che una classe impacchetta funzioni e variabili.
Le classi hanno metodi e attributi
In Python, la creazione di un metodo definisce un comportamento di classe. La parola metodo è il nome OOP assegnato a una funzione definita all'interno di una classe. Per riassumere -
Class functions - è sinonimo di methods
Class variables - è sinonimo di name attributes.
Class - un progetto per un'istanza con un comportamento esatto.
Object - una delle istanze della classe, esegue la funzionalità definita nella classe.
Type - indica la classe a cui appartiene l'istanza
Attribute - Qualsiasi valore oggetto: object.attribute
Method - un “attributo richiamabile” definito nella classe
Ad esempio, osserva la seguente parte di codice:
var = “Hello, John”
print( type (var)) # < type ‘str’> or <class 'str'>
print(var.upper()) # upper() method is called, HELLO, JOHN
Creazione e istanziazione
Il codice seguente mostra come creare la nostra prima classe e poi la sua istanza.
class MyClass(object):
pass
# Create first instance of MyClass
this_obj = MyClass()
print(this_obj)
# Another instance of MyClass
that_obj = MyClass()
print (that_obj)
Qui abbiamo creato una classe chiamata MyClasse che non svolge alcun compito. L'argomentoobject in MyClass class implica l'ereditarietà delle classi e sarà discusso nei capitoli successivi. pass nel codice sopra indica che questo blocco è vuoto, cioè è una definizione di classe vuota.
Creiamo un'istanza this_obj di MyClass() class e stampalo come mostrato -
<__main__.MyClass object at 0x03B08E10>
<__main__.MyClass object at 0x0369D390>
Qui abbiamo creato un'istanza di MyClass.Il codice esadecimale si riferisce all'indirizzo in cui viene archiviato l'oggetto. Un'altra istanza punta a un altro indirizzo.
Ora definiamo una variabile all'interno della classe MyClass() e ottieni la variabile dall'istanza di quella classe come mostrato nel codice seguente:
class MyClass(object):
var = 9
# Create first instance of MyClass
this_obj = MyClass()
print(this_obj.var)
# Another instance of MyClass
that_obj = MyClass()
print (that_obj.var)
Produzione
È possibile osservare il seguente output quando si esegue il codice sopra riportato:
9
9
Poiché l'istanza sa da quale classe viene istanziata, quindi quando viene richiesto un attributo da un'istanza, l'istanza cerca l'attributo e la classe. Questo è chiamatoattribute lookup.
Metodi di istanza
Una funzione definita in una classe è chiamata a method.Un metodo di istanza richiede un'istanza per chiamarlo e non richiede un decoratore. Quando si crea un metodo di istanza, il primo parametro è sempreself. Sebbene possiamo chiamarlo (self) con qualsiasi altro nome, si consiglia di utilizzare self, poiché è una convenzione di denominazione.
class MyClass(object):
var = 9
def firstM(self):
print("hello, World")
obj = MyClass()
print(obj.var)
obj.firstM()
Produzione
È possibile osservare il seguente output quando si esegue il codice sopra riportato:
9
hello, World
Si noti che nel programma precedente, abbiamo definito un metodo con self come argomento. Ma non possiamo chiamare il metodo perché non abbiamo dichiarato alcun argomento.
class MyClass(object):
def firstM(self):
print("hello, World")
print(self)
obj = MyClass()
obj.firstM()
print(obj)
Produzione
È possibile osservare il seguente output quando si esegue il codice sopra riportato:
hello, World
<__main__.MyClass object at 0x036A8E10>
<__main__.MyClass object at 0x036A8E10>
Incapsulamento
L'incapsulamento è uno dei fondamenti dell'OOP. L'OOP ci consente di nascondere la complessità del funzionamento interno dell'oggetto che è vantaggioso per lo sviluppatore nei seguenti modi:
Semplifica e rende facile capire l'utilizzo di un oggetto senza conoscerne gli interni.
Qualsiasi modifica può essere facilmente gestibile.
La programmazione orientata agli oggetti si basa molto sull'incapsulamento. I termini incapsulamento e astrazione (chiamati anche dati nascosti) sono spesso usati come sinonimi. Sono quasi sinonimi, poiché l'astrazione si ottiene attraverso l'incapsulamento.
L'incapsulamento ci fornisce il meccanismo per limitare l'accesso ad alcuni dei componenti dell'oggetto, questo significa che la rappresentazione interna di un oggetto non può essere vista dall'esterno della definizione dell'oggetto. L'accesso a questi dati viene in genere ottenuto tramite metodi speciali:Getters e Setters.
Questi dati vengono archiviati negli attributi di istanza e possono essere manipolati da qualsiasi luogo al di fuori della classe. Per proteggerlo, è necessario accedere a tali dati solo utilizzando metodi di istanza. L'accesso diretto non dovrebbe essere consentito.
class MyClass(object):
def setAge(self, num):
self.age = num
def getAge(self):
return self.age
zack = MyClass()
zack.setAge(45)
print(zack.getAge())
zack.setAge("Fourty Five")
print(zack.getAge())
Produzione
È possibile osservare il seguente output quando si esegue il codice sopra riportato:
45
Fourty Five
I dati devono essere archiviati solo se sono corretti e validi, utilizzando i costrutti di gestione delle eccezioni. Come possiamo vedere sopra, non ci sono restrizioni sull'input dell'utente al metodo setAge (). Potrebbe essere una stringa, un numero o un elenco. Quindi dobbiamo controllare il codice sopra per garantire la correttezza dell'archiviazione.
class MyClass(object):
def setAge(self, num):
self.age = num
def getAge(self):
return self.age
zack = MyClass()
zack.setAge(45)
print(zack.getAge())
zack.setAge("Fourty Five")
print(zack.getAge())
Init Constructor
Il __initIl metodo __ viene chiamato implicitamente non appena viene istanziato un oggetto di una classe. Questo inizializzerà l'oggetto.
x = MyClass()
La riga di codice mostrata sopra creerà una nuova istanza e assegna questo oggetto alla variabile locale x.
L'operazione di istanziazione, cioè calling a class object, crea un oggetto vuoto. A molte classi piace creare oggetti con istanze personalizzate per uno stato iniziale specifico. Pertanto, una classe può definire un metodo speciale chiamato '__init __ ()' come mostrato -
def __init__(self):
self.data = []
Python chiama __init__ durante l'istanziazione per definire un attributo aggiuntivo che dovrebbe verificarsi quando viene istanziata una classe che potrebbe impostare alcuni valori iniziali per quell'oggetto o eseguire una routine richiesta sull'istanza. Quindi in questo esempio, una nuova istanza inizializzata può essere ottenuta da:
x = MyClass()
Il metodo __init __ () può avere argomenti singoli o multipli per una maggiore flessibilità. L'init sta per inizializzazione, poiché inizializza gli attributi dell'istanza. È chiamato il costruttore di una classe.
class myclass(object):
def __init__(self,aaa, bbb):
self.a = aaa
self.b = bbb
x = myclass(4.5, 3)
print(x.a, x.b)
Produzione
4.5 3
Attributi di classe
L'attributo definito nella classe è chiamato "attributi di classe" e gli attributi definiti nella funzione sono chiamati "attributi di istanza". Durante la definizione, questi attributi non sono prefissati da self, poiché sono proprietà della classe e non di un'istanza particolare.
È possibile accedere agli attributi della classe dalla classe stessa (className.attributeName) e dalle istanze della classe (inst.attributeName). Quindi, le istanze hanno accesso sia all'attributo di istanza che agli attributi di classe.
>>> class myclass():
age = 21
>>> myclass.age
21
>>> x = myclass()
>>> x.age
21
>>>
Un attributo di classe può essere sovrascritto in un'istanza, anche se non è un buon metodo per interrompere l'incapsulamento.
C'è un percorso di ricerca per gli attributi in Python. Il primo è il metodo definito all'interno della classe, quindi la classe sopra di essa.
>>> class myclass(object):
classy = 'class value'
>>> dd = myclass()
>>> print (dd.classy) # This should return the string 'class value'
class value
>>>
>>> dd.classy = "Instance Value"
>>> print(dd.classy) # Return the string "Instance Value"
Instance Value
>>>
>>> # This will delete the value set for 'dd.classy' in the instance.
>>> del dd.classy
>>> >>> # Since the overriding attribute was deleted, this will print 'class
value'.
>>> print(dd.classy)
class value
>>>
Stiamo sovrascrivendo l'attributo di classe "classy" nell'istanza dd. Quando è sovrascritto, l'interprete Python legge il valore sovrascritto. Ma una volta che il nuovo valore è stato cancellato con 'del', il valore sovrascritto non è più presente nell'istanza, e quindi la ricerca va di un livello superiore e lo ottiene dalla classe.
Lavorare con dati di classi e istanze
In questa sezione, comprendiamo come i dati della classe si relazionano ai dati dell'istanza. Possiamo memorizzare i dati in una classe o in un'istanza. Quando progettiamo una classe, decidiamo quali dati appartengono all'istanza e quali dati devono essere archiviati nella classe complessiva.
Un'istanza può accedere ai dati della classe. Se creiamo più istanze, queste istanze possono accedere ai valori degli attributi individuali e ai dati della classe complessiva.
Pertanto, i dati di una classe sono i dati condivisi tra tutte le istanze. Rispettare il codice riportato di seguito per una migliore comprensione -
class InstanceCounter(object):
count = 0 # class attribute, will be accessible to all instances
def __init__(self, val):
self.val = val
InstanceCounter.count +=1 # Increment the value of class attribute, accessible through class name
# In above line, class ('InstanceCounter') act as an object
def set_val(self, newval):
self.val = newval
def get_val(self):
return self.val
def get_count(self):
return InstanceCounter.count
a = InstanceCounter(9)
b = InstanceCounter(18)
c = InstanceCounter(27)
for obj in (a, b, c):
print ('val of obj: %s' %(obj.get_val())) # Initialized value ( 9, 18, 27)
print ('count: %s' %(obj.get_count())) # always 3
Produzione
val of obj: 9
count: 3
val of obj: 18
count: 3
val of obj: 27
count: 3
In breve, gli attributi di classe sono gli stessi per tutte le istanze di classe mentre gli attributi di istanza sono particolari per ogni istanza. Per due istanze diverse, avremo due attributi di istanza diversi.
class myClass:
class_attribute = 99
def class_method(self):
self.instance_attribute = 'I am instance attribute'
print (myClass.__dict__)
Produzione
È possibile osservare il seguente output quando si esegue il codice sopra riportato:
{'__module__': '__main__', 'class_attribute': 99, 'class_method': <function myClass.class_method at 0x04128D68>, '__dict__': <attribute '__dict__' of 'myClass' objects>, '__weakref__': <attribute '__weakref__' of 'myClass' objects>, '__doc__': None}
L'attributo dell'istanza myClass.__dict__ come mostrato -
>>> a = myClass()
>>> a.class_method()
>>> print(a.__dict__)
{'instance_attribute': 'I am instance attribute'}
Questo capitolo parla in dettaglio di varie funzioni integrate in Python, operazioni di I / O su file e concetti di sovraccarico.
Funzioni integrate di Python
L'interprete Python ha un numero di funzioni chiamate funzioni integrate che sono prontamente disponibili per l'uso. Nella sua ultima versione, Python contiene 68 funzioni integrate come elencato nella tabella riportata di seguito:
FUNZIONI INTEGRATE | ||||
---|---|---|---|---|
addominali() | dict () | Aiuto() | min () | setattr () |
tutti() | dir () | esadecimale() | Il prossimo() | fetta() |
qualunque() | divmod () | id () | oggetto() | smistato() |
ascii () | enumerare() | ingresso() | ott () | staticmethod () |
bidone() | eval () | int () | Aperto() | str () |
bool () | exec () | isinstance () | ord () | somma() |
bytearray () | filtro() | issubclass () | pow () | super() |
byte () | galleggiante() | iter () | Stampa() | tupla () |
richiamabile () | formato() | len () | proprietà() | genere() |
chr () | frozenset () | elenco() | gamma() | vars () |
classmethod () | getattr () | locali () | repr () | cerniera lampo() |
compilare() | globali () | carta geografica() | invertito () | __importare__() |
complesso() | hasattr () | max () | il giro() | |
delattr () | hash () | memoryview () | impostato() |
Questa sezione discute in breve alcune delle funzioni importanti:
funzione len ()
La funzione len () ottiene la lunghezza di stringhe, elenchi o raccolte. Restituisce la lunghezza o il numero di elementi di un oggetto, dove l'oggetto può essere una stringa, un elenco o una raccolta.
>>> len(['hello', 9 , 45.0, 24])
4
La funzione len () internamente funziona come list.__len__() o tuple.__len__(). Quindi, nota che len () funziona solo su oggetti che hanno un __len__() metodo.
>>> set1
{1, 2, 3, 4}
>>> set1.__len__()
4
Tuttavia, in pratica, preferiamo len() invece di __len__() funzione per i seguenti motivi:
È più efficiente. E non è necessario che un metodo particolare sia scritto per rifiutare l'accesso a metodi speciali come __len__.
È facile da mantenere.
Supporta la compatibilità con le versioni precedenti.
Invertito (seq)
Restituisce l'iteratore inverso. seq deve essere un oggetto che ha il metodo __reversed __ () o supporta il protocollo di sequenza (il metodo __len __ () e il metodo __getitem __ ()). Viene generalmente utilizzato infor loop quando vogliamo scorrere gli elementi da dietro a davanti.
>>> normal_list = [2, 4, 5, 7, 9]
>>>
>>> class CustomSequence():
def __len__(self):
return 5
def __getitem__(self,index):
return "x{0}".format(index)
>>> class funkyback():
def __reversed__(self):
return 'backwards!'
>>> for seq in normal_list, CustomSequence(), funkyback():
print('\n{}: '.format(seq.__class__.__name__), end="")
for item in reversed(seq):
print(item, end=", ")
Il ciclo for alla fine stampa l'elenco invertito di un elenco normale e le istanze delle due sequenze personalizzate. L'output lo mostrareversed() funziona su tutti e tre, ma ha risultati molto diversi quando definiamo __reversed__.
Produzione
È possibile osservare il seguente output quando si esegue il codice sopra riportato:
list: 9, 7, 5, 4, 2,
CustomSequence: x4, x3, x2, x1, x0,
funkyback: b, a, c, k, w, a, r, d, s, !,
Enumerare
Il enumerate () metodo aggiunge un contatore a un iterabile e restituisce l'oggetto enumerate.
La sintassi di enumerate () è -
enumerate(iterable, start = 0)
Ecco il secondo argomento start è facoltativo e per impostazione predefinita l'indice inizia con zero (0).
>>> # Enumerate
>>> names = ['Rajesh', 'Rahul', 'Aarav', 'Sahil', 'Trevor']
>>> enumerate(names)
<enumerate object at 0x031D9F80>
>>> list(enumerate(names))
[(0, 'Rajesh'), (1, 'Rahul'), (2, 'Aarav'), (3, 'Sahil'), (4, 'Trevor')]
>>>
Così enumerate()restituisce un iteratore che restituisce una tupla che tiene il conteggio degli elementi nella sequenza passata. Poiché il valore restituito è un iteratore, accedervi direttamente non è molto utile. Un approccio migliore per enumerate () è mantenere il conteggio all'interno di un ciclo for.
>>> for i, n in enumerate(names):
print('Names number: ' + str(i))
print(n)
Names number: 0
Rajesh
Names number: 1
Rahul
Names number: 2
Aarav
Names number: 3
Sahil
Names number: 4
Trevor
Ci sono molte altre funzioni nella libreria standard, ed ecco un altro elenco di alcune funzioni più ampiamente utilizzate:
hasattr, getattr, setattr e delattr, che consente agli attributi di un oggetto di essere manipolati dai loro nomi di stringa.
all e any, che accetta un oggetto iterabile e restituisce True se tutti o alcuni degli elementi vengono valutati come veri.
nzip, che accetta due o più sequenze e restituisce una nuova sequenza di tuple, dove ogni tupla contiene un singolo valore da ogni sequenza.
File I / O
Il concetto di file è associato al termine programmazione orientata agli oggetti. Python ha racchiuso in astrazione l'interfaccia fornita dai sistemi operativi che ci consente di lavorare con gli oggetti file.
Il open()La funzione incorporata viene utilizzata per aprire un file e restituire un oggetto file. È la funzione più comunemente usata con due argomenti:
open(filename, mode)
La funzione open () chiama due argomenti, il primo è il nome del file e il secondo è la modalità. Qui la modalità può essere "r" per la modalità di sola lettura, "w" per la sola scrittura (un file esistente con lo stesso nome verrà cancellato) e "a" apre il file per l'aggiunta, tutti i dati scritti nel file vengono aggiunti automaticamente all'estremità. 'r +' apre il file sia in lettura che in scrittura. La modalità predefinita è di sola lettura.
Su Windows, "b" aggiunto alla modalità apre il file in modalità binaria, quindi ci sono anche modalità come "rb", "wb" e "r + b".
>>> text = 'This is the first line'
>>> file = open('datawork','w')
>>> file.write(text)
22
>>> file.close()
In alcuni casi, vogliamo semplicemente aggiungere al file esistente piuttosto che sovrascriverlo, per questo potremmo fornire il valore 'a' come argomento di modalità, da aggiungere alla fine del file, piuttosto che sovrascrivere completamente il file esistente Contenuti.
>>> f = open('datawork','a')
>>> text1 = ' This is second line'
>>> f.write(text1)
20
>>> f.close()
Una volta che un file è aperto per la lettura, possiamo chiamare il metodo read, readline o readlines per ottenere il contenuto del file. Il metodo read restituisce l'intero contenuto del file come un oggetto str o bytes, a seconda che il secondo argomento sia "b".
Per la leggibilità e per evitare di leggere un file di grandi dimensioni in una volta sola, è spesso meglio usare un ciclo for direttamente su un oggetto file. Per i file di testo, leggerà ogni riga, una alla volta, e possiamo elaborarla all'interno del corpo del ciclo. Per i file binari, tuttavia, è meglio leggere blocchi di dati di dimensioni fisse utilizzando il metodo read (), passando un parametro per il numero massimo di byte da leggere.
>>> f = open('fileone','r+')
>>> f.readline()
'This is the first line. \n'
>>> f.readline()
'This is the second line. \n'
Scrivendo su un file, tramite il metodo di scrittura sugli oggetti file, verrà scritto un oggetto stringa (byte per i dati binari) nel file. Il metodo writelines accetta una sequenza di stringhe e scrive ciascuno dei valori iterati nel file. Il metodo writelines non aggiunge una nuova riga dopo ogni elemento nella sequenza.
Infine il metodo close () dovrebbe essere chiamato quando abbiamo finito di leggere o scrivere il file, per assicurarci che tutte le scritture memorizzate nel buffer siano scritte sul disco, che il file sia stato adeguatamente ripulito e che tutte le risorse legate al file vengano rilasciate il sistema operativo. È un approccio migliore per chiamare il metodo close () ma tecnicamente ciò avverrà automaticamente quando lo script esiste.
Un'alternativa al sovraccarico del metodo
Il sovraccarico del metodo si riferisce alla presenza di più metodi con lo stesso nome che accettano diversi set di argomenti.
Dato un singolo metodo o funzione, possiamo specificare noi stessi il numero di parametri. A seconda della definizione della funzione, può essere chiamata con zero, uno, due o più parametri.
class Human:
def sayHello(self, name = None):
if name is not None:
print('Hello ' + name)
else:
print('Hello ')
#Create Instance
obj = Human()
#Call the method, else part will be executed
obj.sayHello()
#Call the method with a parameter, if part will be executed
obj.sayHello('Rahul')
Produzione
Hello
Hello Rahul
Argomenti predefiniti
Anche le funzioni sono oggetti
Un oggetto richiamabile è un oggetto che può accettare alcuni argomenti e possibilmente restituirà un oggetto. Una funzione è l'oggetto richiamabile più semplice in Python, ma ce ne sono anche altre come classi o determinate istanze di classe.
Ogni funzione in un Python è un oggetto. Gli oggetti possono contenere metodi o funzioni ma l'oggetto non è una funzione necessaria.
def my_func():
print('My function was called')
my_func.description = 'A silly function'
def second_func():
print('Second function was called')
second_func.description = 'One more sillier function'
def another_func(func):
print("The description:", end=" ")
print(func.description)
print('The name: ', end=' ')
print(func.__name__)
print('The class:', end=' ')
print(func.__class__)
print("Now I'll call the function passed in")
func()
another_func(my_func)
another_func(second_func)
Nel codice sopra, siamo in grado di passare due diverse funzioni come argomento nella nostra terza funzione e ottenere un output diverso per ciascuna -
The description: A silly function
The name: my_func
The class:
Now I'll call the function passed in My function was called The description: One more sillier function The name: second_func The class:
Now I'll call the function passed in Second function was called
callable objects
Just as functions are objects that can have attributes set on them, it is possible to create an object that can be called as though it were a function.
In Python any object with a __call__() method can be called using function-call syntax.
Inheritance and Polymorphism
Inheritance and polymorphism – this is a very important concept in Python. You must understand it better if you want to learn.
Inheritance
One of the major advantages of Object Oriented Programming is re-use. Inheritance is one of the mechanisms to achieve the same. Inheritance allows programmer to create a general or a base class first and then later extend it to more specialized class. It allows programmer to write better code.
Using inheritance you can use or inherit all the data fields and methods available in your base class. Later you can add you own methods and data fields, thus inheritance provides a way to organize code, rather than rewriting it from scratch.
In object-oriented terminology when class X extend class Y, then Y is called super/parent/base class and X is called subclass/child/derived class. One point to note here is that only data fields and method which are not private are accessible by child classes. Private data fields and methods are accessible only inside the class.
syntax to create a derived class is −
class BaseClass:
Body of base class
class DerivedClass(BaseClass):
Body of derived class
Inheriting Attributes
Now look at the below example −
Output
We first created a class called Date and pass the object as an argument, here-object is built-in class provided by Python. Later we created another class called time and called the Date class as an argument. Through this call we get access to all the data and attributes of Date class into the Time class. Because of that when we try to get the get_date method from the Time class object tm we created earlier possible.
Object.Attribute Lookup Hierarchy
- The instance
- The class
- Any class from which this class inherits
Inheritance Examples
Let’s take a closure look into the inheritance example −
Let’s create couple of classes to participate in examples −
- Animal − Class simulate an animal
- Cat − Subclass of Animal
- Dog − Subclass of Animal
In Python, constructor of class used to create an object (instance), and assign the value for the attributes.
Constructor of subclasses always called to a constructor of parent class to initialize value for the attributes in the parent class, then it start assign value for its attributes.
Output
In the above example, we see the command attributes or methods we put in the parent class so that all subclasses or child classes will inherits that property from the parent class.
If a subclass try to inherits methods or data from another subclass then it will through an error as we see when Dog class try to call swatstring() methods from that cat class, it throws an error(like AttributeError in our case).
Polymorphism (“MANY SHAPES”)
Polymorphism is an important feature of class definition in Python that is utilized when you have commonly named methods across classes or subclasses. This permits functions to use entities of different types at different times. So, it provides flexibility and loose coupling so that code can be extended and easily maintained over time.
This allows functions to use objects of any of these polymorphic classes without needing to be aware of distinctions across the classes.
Polymorphism can be carried out through inheritance, with subclasses making use of base class methods or overriding them.
Let understand the concept of polymorphism with our previous inheritance example and add one common method called show_affection in both subclasses −
From the example we can see, it refers to a design in which object of dissimilar type can be treated in the same manner or more specifically two or more classes with method of the same name or common interface because same method(show_affection in below example) is called with either type of objects.
Output
So, all animals show affections (show_affection), but they do differently. The “show_affection” behaviors is thus polymorphic in the sense that it acted differently depending on the animal. So, the abstract “animal” concept does not actually “show_affection”, but specific animals(like dogs and cats) have a concrete implementation of the action “show_affection”.
Python itself have classes that are polymorphic. Example, the len() function can be used with multiple objects and all return the correct output based on the input parameter.
Overriding
In Python, when a subclass contains a method that overrides a method of the superclass, you can also call the superclass method by calling
Super(Subclass, self).method instead of self.method.
Example
class Thought(object):
def __init__(self):
pass
def message(self):
print("Thought, always come and go")
class Advice(Thought):
def __init__(self):
super(Advice, self).__init__()
def message(self):
print('Warning: Risk is always involved when you are dealing with market!')
Inheriting the Constructor
If we see from our previous inheritance example, __init__ was located in the parent class in the up ‘cause the child class dog or cat didn’t‘ve __init__ method in it. Python used the inheritance attribute lookup to find __init__ in animal class. When we created the child class, first it will look the __init__ method in the dog class, then it didn’t find it then looked into parent class Animal and found there and called that there. So as our class design became complex we may wish to initialize a instance firstly processing it through parent class constructor and then through child class constructor.
Output
In above example- all animals have a name and all dogs a particular breed. We called parent class constructor with super. So dog has its own __init__ but the first thing that happen is we call super. Super is built in function and it is designed to relate a class to its super class or its parent class.
In this case we saying that get the super class of dog and pass the dog instance to whatever method we say here the constructor __init__. So in another words we are calling parent class Animal __init__ with the dog object. You may ask why we won’t just say Animal __init__ with the dog instance, we could do this but if the name of animal class were to change, sometime in the future. What if we wanna rearrange the class hierarchy,so the dog inherited from another class. Using super in this case allows us to keep things modular and easy to change and maintain.
So in this example we are able to combine general __init__ functionality with more specific functionality. This gives us opportunity to separate common functionality from the specific functionality which can eliminate code duplication and relate class to one another in a way that reflects the system overall design.
Conclusion
__init__ is like any other method; it can be inherited
If a class does not have a __init__ constructor, Python will check its parent class to see if it can find one.
As soon as it finds one, Python calls it and stops looking
We can use the super () function to call methods in the parent class.
We may want to initialize in the parent as well as our own class.
Multiple Inheritance and the Lookup Tree
As its name indicates, multiple inheritance is Python is when a class inherits from multiple classes.
For example, a child inherits personality traits from both parents (Mother and Father).
Python Multiple Inheritance Syntax
To make a class inherits from multiple parents classes, we write the the names of these classes inside the parentheses to the derived class while defining it. We separate these names with comma.
Below is an example of that −
>>> class Mother:
pass
>>> class Father:
pass
>>> class Child(Mother, Father):
pass
>>> issubclass(Child, Mother) and issubclass(Child, Father)
True
Multiple inheritance refers to the ability of inheriting from two or more than two class. The complexity arises as child inherits from parent and parents inherits from the grandparent class. Python climbs an inheriting tree looking for attributes that is being requested to be read from an object. It will check the in the instance, within class then parent class and lastly from the grandparent class. Now the question arises in what order the classes will be searched - breath-first or depth-first. By default, Python goes with the depth-first.
That’s is why in the below diagram the Python searches the dothis() method first in class A. So the method resolution order in the below example will be
Mro- D→B→A→C
Look at the below multiple inheritance diagram −
Let’s go through an example to understand the “mro” feature of an Python.
Output
Example 3
Let’s take another example of “diamond shape” multiple inheritance.
Above diagram will be considered ambiguous. From our previous example understanding “method resolution order” .i.e. mro will be D→B→A→C→A but it’s not. On getting the second A from the C, Python will ignore the previous A. so the mro will be in this case will be D→B→C→A.
Let’s create an example based on above diagram −
Output
Simple rule to understand the above output is- if the same class appear in the method resolution order, the earlier appearances of this class will be remove from the method resolution order.
In conclusion −
Any class can inherit from multiple classes
Python normally uses a “depth-first” order when searching inheriting classes.
But when two classes inherit from the same class, Python eliminates the first appearances of that class from the mro.
Decorators, Static and Class Methods
Functions(or methods) are created by def statement.
Though methods works in exactly the same way as a function except one point where method first argument is instance object.
We can classify methods based on how they behave, like
Simple method − defined outside of a class. This function can access class attributes by feeding instance argument:
def outside_func(():
Instance method −
def func(self,)
Class method − if we need to use class attributes
@classmethod
def cfunc(cls,)
Static method − do not have any info about the class
@staticmethod
def sfoo()
Till now we have seen the instance method, now is the time to get some insight into the other two methods,
Class Method
The @classmethod decorator, is a builtin function decorator that gets passed the class it was called on or the class of the instance it was called on as first argument. The result of that evaluation shadows your function definition.
syntax
class C(object):
@classmethod
def fun(cls, arg1, arg2, ...):
....
fun: function that needs to be converted into a class method
returns: a class method for function
They have the access to this cls argument, it can’t modify object instance state. That would require access to self.
It is bound to the class and not the object of the class.
Class methods can still modify class state that applies across all instances of the class.
Static Method
A static method takes neither a self nor a cls(class) parameter but it’s free to accept an arbitrary number of other parameters.
syntax
class C(object):
@staticmethod
def fun(arg1, arg2, ...):
...
returns: a static method for function funself.
- A static method can neither modify object state nor class state.
- They are restricted in what data they can access.
When to use what
We generally use class method to create factory methods. Factory methods return class object (similar to a constructor) for different use cases.
We generally use static methods to create utility functions.
Python Design Pattern
Overview
Modern software development needs to address complex business requirements. It also needs to take into account factors such as future extensibility and maintainability. A good design of a software system is vital to accomplish these goals. Design patterns play an important role in such systems.
To understand design pattern, let’s consider below example −
Every car’s design follows a basic design pattern, four wheels, steering wheel, the core drive system like accelerator-break-clutch, etc.
So, all things repeatedly built/ produced, shall inevitably follow a pattern in its design.. it cars, bicycle, pizza, atm machines, whatever…even your sofa bed.
Designs that have almost become standard way of coding some logic/mechanism/technique in software, hence come to be known as or studied as, Software Design Patterns.
Why is Design Pattern Important?
Benefits of using Design Patterns are −
Helps you to solve common design problems through a proven approach.
No ambiguity in the understanding as they are well documented.
Reduce the overall development time.
Helps you deal with future extensions and modifications with more ease than otherwise.
May reduce errors in the system since they are proven solutions to common problems.
Classification of Design Patterns
The GoF (Gang of Four) design patterns are classified into three categories namely creational, structural and behavioral.
Creational Patterns
Creational design patterns separate the object creation logic from the rest of the system. Instead of you creating objects, creational patterns creates them for you. The creational patterns include Abstract Factory, Builder, Factory Method, Prototype and Singleton.
Creational Patterns are not commonly used in Python because of the dynamic nature of the language. Also language itself provide us with all the flexibility we need to create in a sufficient elegant fashion, we rarely need to implement anything on top, like singleton or Factory.
Also these patterns provide a way to create objects while hiding the creation logic, rather than instantiating objects directly using a new operator.
Structural Patterns
Sometimes instead of starting from scratch, you need to build larger structures by using an existing set of classes. That’s where structural class patterns use inheritance to build a new structure. Structural object patterns use composition/ aggregation to obtain a new functionality. Adapter, Bridge, Composite, Decorator, Façade, Flyweight and Proxy are Structural Patterns. They offers best ways to organize class hierarchy.
Behavioral Patterns
Behavioral patterns offers best ways of handling communication between objects. Patterns comes under this categories are: Visitor, Chain of responsibility, Command, Interpreter, Iterator, Mediator, Memento, Observer, State, Strategy and Template method are Behavioral Patterns.
Because they represent the behavior of a system, they are used generally to describe the functionality of software systems.
Commonly used Design Patterns
Singleton
It is one of the most controversial and famous of all design patterns. It is used in overly object-oriented languages, and is a vital part of traditional object-oriented programming.
The Singleton pattern is used for,
When logging needs to be implemented. The logger instance is shared by all the components of the system.
The configuration files is using this because cache of information needs to be maintained and shared by all the various components in the system.
Managing a connection to a database.
Here is the UML diagram,
class Logger(object):
def __new__(cls, *args, **kwargs):
if not hasattr(cls, '_logger'):
cls._logger = super(Logger, cls).__new__(cls, *args, **kwargs)
return cls._logger
In this example, Logger is a Singleton.
When __new__ is called, it normally constructs a new instance of that class. When we override it, we first check if our singleton instance has been created or not. If not, we create it using a super call. Thus, whenever we call the constructor on Logger, we always get the exact same instance.
>>>
>>> obj1 = Logger()
>>> obj2 = Logger()
>>> obj1 == obj2
True
>>>
>>> obj1
<__main__.Logger object at 0x03224090>
>>> obj2
<__main__.Logger object at 0x03224090>
Object Oriented Python - Advanced Features
In this we will look into some of the advanced features which Python provide
Core Syntax in our Class design
In this we will look onto, how Python allows us to take advantage of operators in our classes. Python is largely objects and methods call on objects and this even goes on even when its hidden by some convenient syntax.
>>> var1 = 'Hello'
>>> var2 = ' World!'
>>> var1 + var2
'Hello World!'
>>>
>>> var1.__add__(var2)
'Hello World!'
>>> num1 = 45
>>> num2 = 60
>>> num1.__add__(num2)
105
>>> var3 = ['a', 'b']
>>> var4 = ['hello', ' John']
>>> var3.__add__(var4)
['a', 'b', 'hello', ' John']
So if we have to add magic method __add__ to our own classes, could we do that too. Let’s try to do that.
We have a class called Sumlist which has a contructor __init__ which takes list as an argument called my_list.
class SumList(object):
def __init__(self, my_list):
self.mylist = my_list
def __add__(self, other):
new_list = [ x + y for x, y in zip(self.mylist, other.mylist)]
return SumList(new_list)
def __repr__(self):
return str(self.mylist)
aa = SumList([3,6, 9, 12, 15])
bb = SumList([100, 200, 300, 400, 500])
cc = aa + bb # aa.__add__(bb)
print(cc) # should gives us a list ([103, 206, 309, 412, 515])
Output
[103, 206, 309, 412, 515]
But there are many methods which are internally managed by others magic methods. Below are some of them,
'abc' in var # var.__contains__('abc')
var == 'abc' # var.__eq__('abc')
var[1] # var.__getitem__(1)
var[1:3] # var.__getslice__(1, 3)
len(var) # var.__len__()
print(var) # var.__repr__()
Inheriting From built-in types
Classes can also inherit from built-in types this means inherits from any built-in and take advantage of all the functionality found there.
In below example we are inheriting from dictionary but then we are implementing one of its method __setitem__. This (setitem) is invoked when we set key and value in the dictionary. As this is a magic method, this will be called implicitly.
class MyDict(dict):
def __setitem__(self, key, val):
print('setting a key and value!')
dict.__setitem__(self, key, val)
dd = MyDict()
dd['a'] = 10
dd['b'] = 20
for key in dd.keys():
print('{0} = {1}'.format(key, dd[key]))
Output
setting a key and value!
setting a key and value!
a = 10
b = 20
Let’s extend our previous example, below we have called two magic methods called __getitem__ and __setitem__ better invoked when we deal with list index.
# Mylist inherits from 'list' object but indexes from 1 instead for 0!
class Mylist(list): # inherits from list
def __getitem__(self, index):
if index == 0:
raise IndexError
if index > 0:
index = index - 1
return list.__getitem__(self, index) # this method is called when
# we access a value with subscript like x[1]
def __setitem__(self, index, value):
if index == 0:
raise IndexError
if index > 0:
index = index - 1
list.__setitem__(self, index, value)
x = Mylist(['a', 'b', 'c']) # __init__() inherited from builtin list
print(x) # __repr__() inherited from builtin list
x.append('HELLO'); # append() inherited from builtin list
print(x[1]) # 'a' (Mylist.__getitem__ cutomizes list superclass
# method. index is 1, but reflects 0!
print (x[4]) # 'HELLO' (index is 4 but reflects 3!
Output
['a', 'b', 'c']
a
HELLO
In above example, we set a three item list in Mylist and implicitly __init__ method is called and when we print the element x, we get the three item list ([‘a’,’b’,’c’]). Then we append another element to this list. Later we ask for index 1 and index 4. But if you see the output, we are getting element from the (index-1) what we have asked for. As we know list indexing start from 0 but here the indexing start from 1 (that’s why we are getting the first item of the list).
Naming Conventions
In this we will look into names we’ll used for variables especially private variables and conventions used by Python programmers worldwide. Although variables are designated as private but there is not privacy in Python and this by design. Like any other well documented languages, Python has naming and style conventions that it promote although it doesn’t enforce them. There is a style guide written by “Guido van Rossum” the originator of Python, that describe the best practices and use of name and is called PEP8. Here is the link for this, https://www.python.org/dev/peps/pep-0008/
PEP stands for Python enhancement proposal and is a series of documentation that distributed among the Python community to discuss proposed changes. For example it is recommended all,
- Module names − all_lower_case
- Class names and exception names − CamelCase
- Global and local names − all_lower_case
- Functions and method names − all_lower_case
- Constants − ALL_UPPER_CASE
These are just the recommendation, you can vary if you like. But as most of the developers follows these recommendation so might me your code is less readable.
Why conform to convention?
We can follow the PEP recommendation we it allows us to get,
- More familiar to the vast majority of developers
- Clearer to most readers of your code.
- Will match style of other contributers who work on same code base.
- Mark of a professional software developers
- Everyone will accept you.
Variable Naming − ‘Public’ and ‘Private’
In Python, when we are dealing with modules and classes, we designate some variables or attribute as private. In Python, there is no existence of “Private” instance variable which cannot be accessed except inside an object. Private simply means they are simply not intended to be used by the users of the code instead they are intended to be used internally. In general, a convention is being followed by most Python developers i.e. a name prefixed with an underscore for example. _attrval (example below) should be treated as a non-public part of the API or any Python code, whether it is a function, a method or a data member. Below is the naming convention we follow,
Public attributes or variables (intended to be used by the importer of this module or user of this class) −regular_lower_case
Private attributes or variables (internal use by the module or class) −_single_leading_underscore
Private attributes that shouldn’t be subclassed −__double_leading_underscore
Magic attributes −__double_underscores__(use them, don’t create them)
class GetSet(object):
instance_count = 0 # public
__mangled_name = 'no privacy!' # special variable
def __init__(self, value):
self._attrval = value # _attrval is for internal use only
GetSet.instance_count += 1
@property
def var(self):
print('Getting the "var" attribute')
return self._attrval
@var.setter
def var(self, value):
print('setting the "var" attribute')
self._attrval = value
@var.deleter
def var(self):
print('deleting the "var" attribute')
self._attrval = None
cc = GetSet(5)
cc.var = 10 # public name
print(cc._attrval)
print(cc._GetSet__mangled_name)
Output
setting the "var" attribute
10
no privacy!
Object Oriented Python - Files and Strings
Strings
Strings are the most popular data types used in every programming language. Why? Because we, understand text better than numbers, so in writing and talking we use text and words, similarly in programming too we use strings. In string we parse text, analyse text semantics, and do data mining – and all this data is human consumed text.The string in Python is immutable.
String Manipulation
In Python, string can be marked in multiple ways, using single quote ( ‘ ), double quote( “ ) or even triple quote ( ‘’’ ) in case of multiline strings.
>>> # String Examples
>>> a = "hello"
>>> b = ''' A Multi line string,
Simple!'''
>>> e = ('Multiple' 'strings' 'togethers')
String manipulation is very useful and very widely used in every language. Often, programmers are required to break down strings and examine them closely.
Strings can be iterated over (character by character), sliced, or concatenated. The syntax is the same as for lists.
The str class has numerous methods on it to make manipulating strings easier. The dir and help commands provides guidance in the Python interpreter how to use them.
Below are some of the commonly used string methods we use.
Sr.No.
Method & Description
1
isalpha()
Checks if all characters are Alphabets
2
isdigit()
Checks Digit Characters
3
isdecimal()
Checks decimal Characters
4
isnumeric()
checks Numeric Characters
5
find()
Returns the Highest Index of substrings
6
istitle()
Checks for Titlecased strings
7
join()
Returns a concatenated string
8
lower()
returns lower cased string
9
upper()
returns upper cased string
10
partion()
Returns a tuple
11
bytearray()
Returns array of given byte size
12
enumerate()
Returns an enumerate object
13
isprintable()
Checks printable character
Let’s try to run couple of string methods,
>>> str1 = 'Hello World!'
>>> str1.startswith('h')
False
>>> str1.startswith('H')
True
>>> str1.endswith('d')
False
>>> str1.endswith('d!')
True
>>> str1.find('o')
4
>>> #Above returns the index of the first occurence of the character/substring.
>>> str1.find('lo')
3
>>> str1.upper()
'HELLO WORLD!'
>>> str1.lower()
'hello world!'
>>> str1.index('b')
Traceback (most recent call last):
File "<pyshell#19>", line 1, in <module>
str1.index('b')
ValueError: substring not found
>>> s = ('hello How Are You')
>>> s.split(' ')
['hello', 'How', 'Are', 'You']
>>> s1 = s.split(' ')
>>> '*'.join(s1)
'hello*How*Are*You'
>>> s.partition(' ')
('hello', ' ', 'How Are You')
>>>
String Formatting
In Python 3.x formatting of strings has changed, now it more logical and is more flexible. Formatting can be done using the format() method or the % sign(old style) in format string.
The string can contain literal text or replacement fields delimited by braces {} and each replacement field may contains either the numeric index of a positional argument or the name of a keyword argument.
syntax
str.format(*args, **kwargs)
Basic Formatting
>>> '{} {}'.format('Example', 'One')
'Example One'
>>> '{} {}'.format('pie', '3.1415926')
'pie 3.1415926'
Below example allows re-arrange the order of display without changing the arguments.
>>> '{1} {0}'.format('pie', '3.1415926')
'3.1415926 pie'
Padding and aligning strings
A value can be padded to a specific length.
>>> #Padding Character, can be space or special character
>>> '{:12}'.format('PYTHON')
'PYTHON '
>>> '{:>12}'.format('PYTHON')
' PYTHON'
>>> '{:<{}s}'.format('PYTHON',12)
'PYTHON '
>>> '{:*<12}'.format('PYTHON')
'PYTHON******'
>>> '{:*^12}'.format('PYTHON')
'***PYTHON***'
>>> '{:.15}'.format('PYTHON OBJECT ORIENTED PROGRAMMING')
'PYTHON OBJECT O'
>>> #Above, truncated 15 characters from the left side of a specified string
>>> '{:.{}}'.format('PYTHON OBJECT ORIENTED',15)
'PYTHON OBJECT O'
>>> #Named Placeholders
>>> data = {'Name':'Raghu', 'Place':'Bangalore'}
>>> '{Name} {Place}'.format(**data)
'Raghu Bangalore'
>>> #Datetime
>>> from datetime import datetime
>>> '{:%Y/%m/%d.%H:%M}'.format(datetime(2018,3,26,9,57))
'2018/03/26.09:57'
Strings are Unicode
Strings as collections of immutable Unicode characters. Unicode strings provide an opportunity to create software or programs that works everywhere because the Unicode strings can represent any possible character not just the ASCII characters.
Many IO operations only know how to deal with bytes, even if the bytes object refers to textual data. It is therefore very important to know how to interchange between bytes and Unicode.
Converting text to bytes
Converting a strings to byte object is termed as encoding. There are numerous forms of encoding, most common ones are: PNG; JPEG, MP3, WAV, ASCII, UTF-8 etc. Also this(encoding) is a format to represent audio, images, text, etc. in bytes.
This conversion is possible through encode(). It take encoding technique as argument. By default, we use ‘UTF-8’ technique.
>>> # Python Code to demonstrate string encoding
>>>
>>> # Initialising a String
>>> x = 'TutorialsPoint'
>>>
>>> #Initialising a byte object
>>> y = b'TutorialsPoint'
>>>
>>> # Using encode() to encode the String >>> # encoded version of x is stored in z using ASCII mapping
>>> z = x.encode('ASCII')
>>>
>>> # Check if x is converted to bytes or not
>>>
>>> if(z==y):
print('Encoding Successful!')
else:
print('Encoding Unsuccessful!')
Encoding Successful!
Converting bytes to text
Converting bytes to text is called the decoding. This is implemented through decode(). We can convert a byte string to a character string if we know which encoding is used to encode it.
So Encoding and decoding are inverse processes.
>>>
>>> # Python code to demonstrate Byte Decoding
>>>
>>> #Initialise a String
>>> x = 'TutorialsPoint'
>>>
>>> #Initialising a byte object
>>> y = b'TutorialsPoint'
>>>
>>> #using decode() to decode the Byte object
>>> # decoded version of y is stored in z using ASCII mapping
>>> z = y.decode('ASCII')
>>> #Check if y is converted to String or not
>>> if (z == x):
print('Decoding Successful!')
else:
print('Decoding Unsuccessful!') Decoding Successful!
>>>
File I/O
Operating systems represents files as a sequence of bytes, not text.
A file is a named location on disk to store related information. It is used to permanently store data in your disk.
In Python, a file operation takes place in the following order.
- Open a file
- Read or write onto a file (operation).Open a file
- Close the file.
Python wraps the incoming (or outgoing) stream of bytes with appropriate decode (or encode) calls so we can deal directly with str objects.
Opening a file
Python has a built-in function open() to open a file. This will generate a file object, also called a handle as it is used to read or modify the file accordingly.
>>> f = open(r'c:\users\rajesh\Desktop\index.webm','rb')
>>> f
<_io.BufferedReader name='c:\\users\\rajesh\\Desktop\\index.webm'>
>>> f.mode
'rb'
>>> f.name
'c:\\users\\rajesh\\Desktop\\index.webm'
For reading text from a file, we only need to pass the filename into the function. The file will be opened for reading, and the bytes will be converted to text using the platform default encoding.
Exception and Exception Classes
In general, an exception is any unusual condition. Exception usually indicates errors but sometimes they intentionally puts in the program, in cases like terminating a procedure early or recovering from a resource shortage. There are number of built-in exceptions, which indicate conditions like reading past the end of a file, or dividing by zero. We can define our own exceptions called custom exception.
Exception handling enables you handle errors gracefully and do something meaningful about it. Exception handling has two components: “throwing” and ‘catching’.
Identifying Exception (Errors)
Every error occurs in Python result an exception which will an error condition identified by its error type.
>>> #Exception
>>> 1/0
Traceback (most recent call last):
File "<pyshell#2>", line 1, in <module>
1/0
ZeroDivisionError: division by zero
>>>
>>> var = 20
>>> print(ver)
Traceback (most recent call last):
File "<pyshell#5>", line 1, in <module>
print(ver)
NameError: name 'ver' is not defined
>>> #Above as we have misspelled a variable name so we get an NameError.
>>>
>>> print('hello)
SyntaxError: EOL while scanning string literal
>>> #Above we have not closed the quote in a string, so we get SyntaxError.
>>>
>>> #Below we are asking for a key, that doen't exists.
>>> mydict = {}
>>> mydict['x']
Traceback (most recent call last):
File "<pyshell#15>", line 1, in <module>
mydict['x']
KeyError: 'x'
>>> #Above keyError
>>>
>>> #Below asking for a index that didn't exist in a list.
>>> mylist = [1,2,3,4]
>>> mylist[5]
Traceback (most recent call last):
File "<pyshell#20>", line 1, in <module>
mylist[5]
IndexError: list index out of range
>>> #Above, index out of range, raised IndexError.
Catching/Trapping Exception
When something unusual occurs in your program and you wish to handle it using the exception mechanism, you ‘throw an exception’. The keywords try and except are used to catch exceptions. Whenever an error occurs within a try block, Python looks for a matching except block to handle it. If there is one, execution jumps there.
syntax
try:
#write some code
#that might throw some exception
except <ExceptionType>:
# Exception handler, alert the user
The code within the try clause will be executed statement by statement.
If an exception occurs, the rest of the try block will be skipped and the except clause will be executed.
try:
some statement here
except:
exception handling
Let’s write some code to see what happens when you not use any error handling mechanism in your program.
number = int(input('Please enter the number between 1 & 10: '))
print('You have entered number',number)
Above programme will work correctly as long as the user enters a number, but what happens if the users try to puts some other data type(like a string or a list).
Please enter the number between 1 > 10: 'Hi'
Traceback (most recent call last):
File "C:/Python/Python361/exception2.py", line 1, in <module>
number = int(input('Please enter the number between 1 & 10: '))
ValueError: invalid literal for int() with base 10: "'Hi'"
Now ValueError is an exception type. Let’s try to rewrite the above code with exception handling.
import sys
print('Previous code with exception handling')
try:
number = int(input('Enter number between 1 > 10: '))
except(ValueError):
print('Error..numbers only')
sys.exit()
print('You have entered number: ',number)
If we run the program, and enter a string (instead of a number), we can see that we get a different result.
Previous code with exception handling
Enter number between 1 > 10: 'Hi'
Error..numbers only
Raising Exceptions
To raise your exceptions from your own methods you need to use raise keyword like this
raise ExceptionClass(‘Some Text Here’)
Let’s take an example
def enterAge(age):
if age<0:
raise ValueError('Only positive integers are allowed')
if age % 2 ==0:
print('Entered Age is even')
else:
print('Entered Age is odd')
try:
num = int(input('Enter your age: '))
enterAge(num)
except ValueError:
print('Only positive integers are allowed')
Run the program and enter positive integer.
Expected Output
Enter your age: 12
Entered Age is even
But when we try to enter a negative number we get,
Expected Output
Enter your age: -2
Only positive integers are allowed
Creating Custom exception class
You can create a custom exception class by Extending BaseException class or subclass of BaseException.
From above diagram we can see most of the exception classes in Python extends from the BaseException class. You can derive your own exception class from BaseException class or from its subclass.
Create a new file called NegativeNumberException.py and write the following code.
class NegativeNumberException(RuntimeError):
def __init__(self, age):
super().__init__()
self.age = age
Above code creates a new exception class named NegativeNumberException, which consists of only constructor which call parent class constructor using super()__init__() and sets the age.
Now to create your own custom exception class, will write some code and import the new exception class.
from NegativeNumberException import NegativeNumberException
def enterage(age):
if age < 0:
raise NegativeNumberException('Only positive integers are allowed')
if age % 2 == 0:
print('Age is Even')
else:
print('Age is Odd')
try:
num = int(input('Enter your age: '))
enterage(num)
except NegativeNumberException:
print('Only positive integers are allowed')
except:
print('Something is wrong')
Output
Enter your age: -2
Only positive integers are allowed
Another way to create a custom Exception class.
class customException(Exception):
def __init__(self, value):
self.parameter = value
def __str__(self):
return repr(self.parameter)
try:
raise customException('My Useful Error Message!')
except customException as instance:
print('Caught: ' + instance.parameter)
Output
Caught: My Useful Error Message!
Exception hierarchy
The class hierarchy for built-in exceptions is −
+-- SystemExit
+-- KeyboardInterrupt
+-- GeneratorExit
+-- Exception
+-- StopIteration
+-- StopAsyncIteration
+-- ArithmeticError
| +-- FloatingPointError
| +-- OverflowError
| +-- ZeroDivisionError
+-- AssertionError
+-- AttributeError
+-- BufferError
+-- EOFError
+-- ImportError
+-- LookupError
| +-- IndexError
| +-- KeyError
+-- MemoryError
+-- NameError
| +-- UnboundLocalError
+-- OSError
| +-- BlockingIOError
| +-- ChildProcessError
| +-- ConnectionError
| | +-- BrokenPipeError
| | +-- ConnectionAbortedError
| | +-- ConnectionRefusedError
| | +-- ConnectionResetError
| +-- FileExistsError
| +-- FileNotFoundError
| +-- InterruptedError
| +-- IsADirectoryError
| +-- NotADirectoryError
| +-- PermissionError
| +-- ProcessLookupError
| +-- TimeoutError
+-- ReferenceError
+-- RuntimeError
| +-- NotImplementedError
| +-- RecursionError
+-- SyntaxError
| +-- IndentationError
| +-- TabError
+-- SystemError
+-- TypeError
+-- ValueError
| +-- UnicodeError
| +-- UnicodeDecodeError
| +-- UnicodeEncodeError
| +-- UnicodeTranslateError
+-- Warning
+-- DeprecationWarning
+-- PendingDeprecationWarning
+-- RuntimeWarning
+-- SyntaxWarning
+-- UserWarning
+-- FutureWarning
+-- ImportWarning
+-- UnicodeWarning
+-- BytesWarning
+-- ResourceWarning
Object Oriented Python - Object Serialization
In the context of data storage, serialization is the process of translating data structures or object state into a format that can be stored (for example, in a file or memory buffer) or transmitted and reconstructed later.
In serialization, an object is transformed into a format that can be stored, so as to be able to deserialize it later and recreate the original object from the serialized format.
Pickle
Pickling is the process whereby a Python object hierarchy is converted into a byte stream (usually not human readable) to be written to a file, this is also known as Serialization. Unpickling is the reverse operation, whereby a byte stream is converted back into a working Python object hierarchy.
Pickle is operationally simplest way to store the object. The Python Pickle module is an object-oriented way to store objects directly in a special storage format.
What can it do?
- Pickle can store and reproduce dictionaries and lists very easily.
- Stores object attributes and restores them back to the same State.
What pickle can’t do?
- It does not save an objects code. Only it’s attributes values.
- It cannot store file handles or connection sockets.
In short we can say, pickling is a way to store and retrieve data variables into and out from files where variables can be lists, classes, etc.
To Pickle something you must −
- import pickle
- Write a variable to file, something like
pickle.dump(mystring, outfile, protocol),
where 3rd argument protocol is optional To unpickling something you must −
Import pickle
Write a variable to a file, something like
myString = pickle.load(inputfile)
Methods
The pickle interface provides four different methods.
dump() − The dump() method serializes to an open file (file-like object).
dumps() − Serializes to a string
load() − Deserializes from an open-like object.
loads() − Deserializes from a string.
Based on above procedure, below is an example of “pickling”.
Output
My Cat pussy is White and has 4 legs
Would you like to see her pickled? Here she is!
b'\x80\x03c__main__\nCat\nq\x00)\x81q\x01}q\x02(X\x0e\x00\x00\x00number_of_legsq\x03K\x04X\x05\x00\x00\x00colorq\x04X\x05\x00\x00\x00Whiteq\x05ub.'
So, in the example above, we have created an instance of a Cat class and then we’ve pickled it, transforming our “Cat” instance into a simple array of bytes.
This way we can easily store the bytes array on a binary file or in a database field and restore it back to its original form from our storage support in a later time.
Also if you want to create a file with a pickled object, you can use the dump() method ( instead of the dumps*()* one) passing also an opened binary file and the pickling result will be stored in the file automatically.
[….]
binary_file = open(my_pickled_Pussy.bin', mode='wb')
my_pickled_Pussy = pickle.dump(Pussy, binary_file)
binary_file.close()
Unpickling
The process that takes a binary array and converts it to an object hierarchy is called unpickling.
The unpickling process is done by using the load() function of the pickle module and returns a complete object hierarchy from a simple bytes array.
Let’s use the load function in our previous example.
Output
MeOw is black
Pussy is white
JSON
JSON(JavaScript Object Notation) has been part of the Python standard library is a lightweight data-interchange format. It is easy for humans to read and write. It is easy to parse and generate.
Because of its simplicity, JSON is a way by which we store and exchange data, which is accomplished through its JSON syntax, and is used in many web applications. As it is in human readable format, and this may be one of the reasons for using it in data transmission, in addition to its effectiveness when working with APIs.
An example of JSON-formatted data is as follow −
{"EmployID": 40203, "Name": "Zack", "Age":54, "isEmployed": True}
Python makes it simple to work with Json files. The module sused for this purpose is the JSON module. This module should be included (built-in) within your Python installation.
So let’s see how can we convert Python dictionary to JSON and write it to a text file.
JSON to Python
Reading JSON means converting JSON into a Python value (object). The json library parses JSON into a dictionary or list in Python. In order to do that, we use the loads() function (load from a string), as follow −
Output
Below is one sample json file,
data1.json
{"menu": {
"id": "file",
"value": "File",
"popup": {
"menuitem": [
{"value": "New", "onclick": "CreateNewDoc()"},
{"value": "Open", "onclick": "OpenDoc()"},
{"value": "Close", "onclick": "CloseDoc()"}
]
}
}}
Above content (Data1.json) looks like a conventional dictionary. We can use pickle to store this file but the output of it is not human readable form.
JSON(Java Script Object Notification) is a very simple format and that’s one of the reason for its popularity. Now let’s look into json output through below program.
Output
Above we open the json file (data1.json) for reading, obtain the file handler and pass on to json.load and getting back the object. When we try to print the output of the object, its same as the json file. Although the type of the object is dictionary, it comes out as a Python object. Writing to the json is simple as we saw this pickle. Above we load the json file, add another key value pair and writing it back to the same json file. Now if we see out data1.json, it looks different .i.e. not in the same format as we see previously.
To make our Output looks same (human readable format), add the couple of arguments into our last line of the program,
json.dump(conf, fh, indent = 4, separators = (‘,’, ‘: ‘))
Similarly like pickle, we can print the string with dumps and load with loads. Below is an example of that,
YAML
YAML may be the most human friendly data serialization standard for all programming languages.
Python yaml module is called pyaml
YAML is an alternative to JSON −
Human readable code − YAML is the most human readable format so much so that even its front-page content is displayed in YAML to make this point.
Compact code − In YAML we use whitespace indentation to denote structure not brackets.
Syntax for relational data − For internal references we use anchors (&) and aliases (*).
One of the area where it is used widely is for viewing/editing of data structures − for example configuration files, dumping during debugging and document headers.
Installing YAML
As yaml is not a built-in module, we need to install it manually. Best way to install yaml on windows machine is through pip. Run below command on your windows terminal to install yaml,
pip install pyaml (Windows machine)
sudo pip install pyaml (*nix and Mac)
On running above command, screen will display something like below based on what’s the current latest version.
Collecting pyaml
Using cached pyaml-17.12.1-py2.py3-none-any.whl
Collecting PyYAML (from pyaml)
Using cached PyYAML-3.12.tar.gz
Installing collected packages: PyYAML, pyaml
Running setup.py install for PyYAML ... done
Successfully installed PyYAML-3.12 pyaml-17.12.1
To test it, go to the Python shell and import the yaml module, import yaml, if no error is found, then we can say installation is successful.
After installing pyaml, let’s look at below code,
script_yaml1.py
Above we created three different data structure, dictionary, list and tuple. On each of the structure, we do yaml.dump. Important point is how the output is displayed on the screen.
Output
Dictionary output looks clean .ie. key: value.
White space to separate different objects.
List is notated with dash (-)
Tuple is indicated first with !!Python/tuple and then in the same format as lists.
Loading a yaml file
So let’s say I have one yaml file, which contains,
---
# An employee record
name: Raagvendra Joshi
job: Developer
skill: Oracle
employed: True
foods:
- Apple
- Orange
- Strawberry
- Mango
languages:
Oracle: Elite
power_builder: Elite
Full Stack Developer: Lame
education:
4 GCSEs
3 A-Levels
MCA in something called com
Now let’s write a code to load this yaml file through yaml.load function. Below is code for the same.
As the output doesn’t looks that much readable, I prettify it by using json in the end. Compare the output we got and the actual yaml file we have.
Output
One of the most important aspect of software development is debugging. In this section we’ll see different ways of Python debugging either with built-in debugger or third party debuggers.
PDB – The Python Debugger
The module PDB supports setting breakpoints. A breakpoint is an intentional pause of the program, where you can get more information about the programs state.
To set a breakpoint, insert the line
pdb.set_trace()
Example
pdb_example1.py
import pdb
x = 9
y = 7
pdb.set_trace()
total = x + y
pdb.set_trace()
We have inserted a few breakpoints in this program. The program will pause at each breakpoint (pdb.set_trace()). To view a variables contents simply type the variable name.
c:\Python\Python361>Python pdb_example1.py
> c:\Python\Python361\pdb_example1.py(8)<module>()
-> total = x + y
(Pdb) x
9
(Pdb) y
7
(Pdb) total
*** NameError: name 'total' is not defined
(Pdb)
Press c or continue to go on with the programs execution until the next breakpoint.
(Pdb) c
--Return--
> c:\Python\Python361\pdb_example1.py(8)<module>()->None
-> total = x + y
(Pdb) total
16
Eventually, you will need to debug much bigger programs – programs that use subroutines. And sometimes, the problem that you’re trying to find will lie inside a subroutine. Consider the following program.
import pdb
def squar(x, y):
out_squared = x^2 + y^2
return out_squared
if __name__ == "__main__":
#pdb.set_trace()
print (squar(4, 5))
Now on running the above program,
c:\Python\Python361>Python pdb_example2.py
> c:\Python\Python361\pdb_example2.py(10)<module>()
-> print (squar(4, 5))
(Pdb)
We can use ? to get help, but the arrow indicates the line that’s about to be executed. At this point it’s helpful to hit s to s to step into that line.
(Pdb) s
--Call--
>c:\Python\Python361\pdb_example2.py(3)squar()
-> def squar(x, y):
This is a call to a function. If you want an overview of where you are in your code, try l −
(Pdb) l
1 import pdb
2
3 def squar(x, y):
4 -> out_squared = x^2 + y^2
5
6 return out_squared
7
8 if __name__ == "__main__":
9 pdb.set_trace()
10 print (squar(4, 5))
[EOF]
(Pdb)
You can hit n to advance to the next line. At this point you are inside the out_squared method and you have access to the variable declared inside the function .i.e. x and y.
(Pdb) x
4
(Pdb) y
5
(Pdb) x^2
6
(Pdb) y^2
7
(Pdb) x**2
16
(Pdb) y**2
25
(Pdb)
So we can see the ^ operator is not what we wanted instead we need to use ** operator to do squares.
This way we can debug our program inside the functions/methods.
Logging
The logging module has been a part of Python’s Standard Library since Python version 2.3. As it’s a built-in module all Python module can participate in logging, so that our application log can include your own message integrated with messages from third party module. It provides a lot of flexibility and functionality.
Benefits of Logging
Diagnostic logging − It records events related to the application’s operation.
Audit logging − It records events for business analysis.
Messages are written and logged at levels of “severity” &minu
DEBUG (debug()) − diagnostic messages for development.
INFO (info()) − standard “progress” messages.
WARNING (warning()) − detected a non-serious issue.
ERROR (error()) − encountered an error, possibly serious.
CRITICAL (critical()) − usually a fatal error (program stops).
Let’s looks into below simple program,
import logging
logging.basicConfig(level=logging.INFO)
logging.debug('this message will be ignored') # This will not print
logging.info('This should be logged') # it'll print
logging.warning('And this, too') # It'll print
Above we are logging messages on severity level. First we import the module, call basicConfig and set the logging level. Level we set above is INFO. Then we have three different statement: debug statement, info statement and a warning statement.
Output of logging1.py
INFO:root:This should be logged
WARNING:root:And this, too
As the info statement is below debug statement, we are not able to see the debug message. To get the debug statement too in the Output terminal, all we need to change is the basicConfig level.
logging.basicConfig(level = logging.DEBUG)
And in the Output we can see,
DEBUG:root:this message will be ignored
INFO:root:This should be logged
WARNING:root:And this, too
Also the default behavior means if we don’t set any logging level is warning. Just comment out the second line from the above program and run the code.
#logging.basicConfig(level = logging.DEBUG)
Output
WARNING:root:And this, too
Python built in logging level are actually integers.
>>> import logging
>>>
>>> logging.DEBUG
10
>>> logging.CRITICAL
50
>>> logging.WARNING
30
>>> logging.INFO
20
>>> logging.ERROR
40
>>>
We can also save the log messages into the file.
logging.basicConfig(level = logging.DEBUG, filename = 'logging.log')
Now all log messages will go the file (logging.log) in your current working directory instead of the screen. This is a much better approach as it lets us to do post analysis of the messages we got.
We can also set the date stamp with our log message.
logging.basicConfig(level=logging.DEBUG, format = '%(asctime)s %(levelname)s:%(message)s')
Output will get something like,
2018-03-08 19:30:00,066 DEBUG:this message will be ignored
2018-03-08 19:30:00,176 INFO:This should be logged
2018-03-08 19:30:00,201 WARNING:And this, too
Benchmarking
Benchmarking or profiling is basically to test how fast is your code executes and where the bottlenecks are? The main reason to do this is for optimization.
timeit
Python comes with a in-built module called timeit. You can use it to time small code snippets. The timeit module uses platform-specific time functions so that you will get the most accurate timings possible.
So, it allows us to compare two shipment of code taken by each and then optimize the scripts to given better performance.
The timeit module has a command line interface, but it can also be imported.
There are two ways to call a script. Let’s use the script first, for that run the below code and see the Output.
import timeit
print ( 'by index: ', timeit.timeit(stmt = "mydict['c']", setup = "mydict = {'a':5, 'b':10, 'c':15}", number = 1000000))
print ( 'by get: ', timeit.timeit(stmt = 'mydict.get("c")', setup = 'mydict = {"a":5, "b":10, "c":15}', number = 1000000))
Output
by index: 0.1809192126703489
by get: 0.6088525265034692
Above we use two different method .i.e. by subscript and get to access the dictionary key value. We execute statement 1 million times as it executes too fast for a very small data. Now we can see the index access much faster as compared to the get. We can run the code multiply times and there will be slight variation in the time execution to get the better understanding.
Another way is to run the above test in the command line. Let’s do it,
c:\Python\Python361>Python -m timeit -n 1000000 -s "mydict = {'a': 5, 'b':10, 'c':15}" "mydict['c']"
1000000 loops, best of 3: 0.187 usec per loop
c:\Python\Python361>Python -m timeit -n 1000000 -s "mydict = {'a': 5, 'b':10, 'c':15}" "mydict.get('c')"
1000000 loops, best of 3: 0.659 usec per loop
Above output may vary based on your system hardware and what all applications are running currently in your system.
Below we can use the timeit module, if we want to call to a function. As we can add multiple statement inside the function to test.
import timeit
def testme(this_dict, key):
return this_dict[key]
print (timeit.timeit("testme(mydict, key)", setup = "from __main__ import testme; mydict = {'a':9, 'b':18, 'c':27}; key = 'c'", number = 1000000))
Output
0.7713474590139164
Object Oriented Python - Libraries
Requests − Python Requests Module
Requests is a Python module which is an elegant and simple HTTP library for Python. With this you can send all kinds of HTTP requests. With this library we can add headers, form data, multipart files and parameters and access the response data.
As Requests is not a built-in module, so we need to install it first.
You can install it by running the following command in the terminal −
pip install requests
Once you have installed the module, you can verify if the installation is successful by typing below command in the Python shell.
import requests
If the installation has been successful, you won’t see any error message.
Making a GET Request
As a means of example we’ll be using the “pokeapi”
Output −
Making POST Requests
The requests library methods for all of the HTTP verbs currently in use. If you wanted to make a simple POST request to an API endpoint then you can do that like so −
req = requests.post(‘http://api/user’, data = None, json = None)
This would work in exactly the same fashion as our previous GET request, however it features two additional keyword parameters −
data which can be populated with say a dictionary, a file or bytes that will be passed in the HTTP body of our POST request.
json which can be populated with a json object that will be passed in the body of our HTTP request also.
Pandas: Python Library Pandas
Pandas is an open-source Python Library providing high-performance data manipulation and analysis tool using its powerful data structures. Pandas is one of the most widely used Python libraries in data science. It is mainly used for data munging, and with good reason: Powerful and flexible group of functionality.
Built on Numpy package and the key data structure is called the DataFrame. These dataframes allows us to store and manipulate tabular data in rows of observations and columns of variables.
There are several ways to create a DataFrame. One way is to use a dictionary. For example −
Output
From the output we can see new brics DataFrame, Pandas has assigned a key for each country as the numerical values 0 through 4.
If instead of giving indexing values from 0 to 4, we would like to have different index values, say the two letter country code, you can do that easily as well −
Adding below one lines in the above code, gives
brics.index = ['BR', 'RU', 'IN', 'CH', 'SA']
Output
Indexing DataFrames
Output
Pygame
Pygame is the open source and cross-platform library that is for making multimedia applications including games. It includes computer graphics and sound libraries designed to be used with the Python programming language. You can develop many cool games with Pygame.’
Overview
Pygame is composed of various modules, each dealing with a specific set of tasks. For example, the display module deals with the display window and screen, the draw module provides functions to draw shapes and the key module works with the keyboard. These are just some of the modules of the library.
The home of the Pygame library is at https://www.pygame.org/news
To make a Pygame application, you follow these steps −
Import the Pygame library
import pygame
Initialize the Pygame library
pygame.init()
Create a window.
screen = Pygame.display.set_mode((560,480))
Pygame.display.set_caption(‘First Pygame Game’)
Initialize game objects
In this step we load images, load sounds, do object positioning, set up some state variables, etc.
Start the game loop.
It is just a loop where we continuously handle events, checks for input, move objects, and draw them. Each iteration of the loop is called a frame.
Let’s put all the above logic into one below program,
Pygame_script.py
Output
Beautiful Soup: Web Scraping with Beautiful Soup
The general idea behind web scraping is to get the data that exists on a website, and convert it into some format that is usable for analysis.
It’s a Python library for pulling data out of HTML or XML files. With your favourite parser it provide idiomatic ways of navigating, searching and modifying the parse tree.
As BeautifulSoup is not a built-in library, we need to install it before we try to use it. To install BeautifulSoup, run the below command
$ apt-get install Python-bs4 # For Linux and Python2
$ apt-get install Python3-bs4 # for Linux based system and Python3.
$ easy_install beautifulsoup4 # For windows machine,
Or
$ pip instal beatifulsoup4 # For window machine
Once the installation is done, we are ready to run few examples and explores Beautifulsoup in details,
Output
Below are some simple ways to navigate that data structure −
One common task is extracting all the URLs found within a page’s <a> tags −
Another common task is extracting all the text from a page −