オブジェクト指向Python-クイックガイド
プログラミング言語は絶えず出現しており、さまざまな方法論もあります。オブジェクト指向プログラミングは、過去数年間で非常に人気のある方法論の1つです。
この章では、Pythonプログラミング言語をオブジェクト指向プログラミング言語にする機能について説明します。
言語プログラミング分類スキーム
Pythonは、オブジェクト指向プログラミングの方法論の下で特徴付けることができます。次の画像は、さまざまなプログラミング言語の特性を示しています。Pythonをオブジェクト指向にする機能を観察してください。
言語クラス | カテゴリ | 言語 |
---|---|---|
プログラミングパラダイム | 手続き | C、C ++、C#、Objective-C、java、Go |
スクリプティング | CoffeeScript、JavaScript、Python、Perl、Php、Ruby | |
機能的 | Clojure、Eralang、Haskell、Scala | |
コンパイルクラス | 静的 | C、C ++、C#、Objective-C、java、Go、Haskell、Scala |
動的 | CoffeeScript、JavaScript、Python、Perl、Php、Ruby、Clojure、Erlang | |
タイプクラス | 強い | C#、java、Go、Python、Ruby、Clojure、Erlang、Haskell、Scala |
弱い | C、C ++、C#、Objective-C、CoffeeScript、JavaScript、Perl、Php | |
メモリクラス | 管理 | その他 |
管理されていない | C、C ++、C#、Objective-C |
オブジェクト指向プログラミングとは何ですか?
Object Orientedオブジェクトに向けられていることを意味します。言い換えれば、それはオブジェクトのモデリングに機能的に向けられていることを意味します。これは、データと動作を介して相互作用するオブジェクトのコレクションを記述することにより、複雑なシステムをモデル化するために使用される多くの手法の1つです。
オブジェクト指向プログラミング(OOP)であるPythonは、オブジェクトとクラスを使用してアプリケーションを設計および構築することに焦点を当てたプログラミング方法です。オブジェクト指向プログラミング(OOP)の主な柱は次のとおりです。 Inheritance, Polymorphism, Abstraction, 広告 Encapsulation。
オブジェクト指向分析(OOA)は、問題、システム、またはタスクを調査し、オブジェクトとそれらの間の相互作用を特定するプロセスです。
オブジェクト指向プログラミングを選ぶ理由
Pythonは、オブジェクト指向のアプローチで設計されました。OOPには次の利点があります-
明確なプログラム構造を提供し、現実世界の問題とその解決策を簡単にマッピングできるようにします。
既存のコードの簡単な保守と変更を容易にします。
各オブジェクトが独立して存在し、既存のオブジェクトを妨げることなく新しい機能を簡単に追加できるため、プログラムのモジュール性が向上します。
提供されたコンポーネントをプログラマーが簡単に適合および変更できるコードライブラリーの優れたフレームワークを提供します。
コードの再利用性を付与します
手続き型プログラミングとオブジェクト指向プログラミング
手続き型プログラミングは、の概念に基づく構造プログラミングから派生しています。 functions/procedure/routines。手続き型プログラミングでは、データに簡単にアクセスして変更できます。一方、オブジェクト指向プログラミング(OOP)では、問題を次のようないくつかの単位に分解できます。objects次に、これらのオブジェクトを中心にデータと関数を作成します。プロシージャや関数よりもデータに重点を置いています。また、OOPでは、データは非表示であり、外部プロシージャからアクセスすることはできません。
次の画像の表は、POPアプローチとOOPアプローチの主な違いを示しています。
手続き型プログラミング(POP)との違い。オブジェクト指向プログラミング(OOP)。
手続き型プログラミング | オブジェクト指向プログラミング | |
---|---|---|
に基づく | Popでは、データと関数に完全に焦点が当てられています | おっと、現実世界のシナリオに基づいています。プログラム全体は、オブジェクトと呼ばれる小さな部分に分割されています |
再利用性 | 制限されたコードの再利用 | コードの再利用 |
アプローチ | トップダウンアプローチ | オブジェクトに焦点を当てたデザイン |
アクセス指定子 | なにもない | パブリック、プライベート、保護 |
データの移動 | データはシステム内の機能間を自由に移動できます | Oopsでは、データはメンバー関数を介して相互に移動および通信できます |
データアクセス | ポップでは、ほとんどの関数は、システム内の関数間で自由にアクセスできる共有にグローバルデータを使用します | おっと、データはメソッド間を自由に移動することはできません。データのアクセスを制御できるように、パブリックまたはプライベートに保持できます。 |
データの隠蔽 | ポップでは、データを隠すための非常に具体的な方法なので、安全性が少し低下します | それはデータ隠蔽を提供し、はるかに安全です |
オーバーロード | ありえない | 関数と演算子のオーバーロード |
例-言語 | C、VB、Fortran、Pascal | C ++、Python、Java、C# |
抽象化 | プロシージャレベルで抽象化を使用します | クラスおよびオブジェクトレベルで抽象化を使用します |
オブジェクト指向プログラミングの原則
オブジェクト指向プログラミング(OOP)は、次の概念に基づいています。 objects アクションではなく、 data論理ではなく。プログラミング言語をオブジェクト指向にするためには、クラスとオブジェクトの操作、および基本的なオブジェクト指向の原則と概念、つまり継承、抽象化、カプセル化、ポリモーフィズムの実装と使用を可能にするメカニズムが必要です。

オブジェクト指向プログラミングの各柱を簡単に理解しましょう-
カプセル化
このプロパティは、不要な詳細を非表示にし、プログラム構造の管理を容易にします。各オブジェクトの実装と状態は、明確に定義された境界の背後に隠されており、オブジェクトを操作するためのクリーンでシンプルなインターフェイスを提供します。これを実現する1つの方法は、データをプライベートにすることです。
継承
一般化とも呼ばれる継承により、クラスとオブジェクトの間の階層関係をキャプチャできます。たとえば、「フルーツ」は「オレンジ」を一般化したものです。継承は、コードの再利用の観点から非常に役立ちます。
抽象化
このプロパティを使用すると、詳細を非表示にして、概念またはオブジェクトの重要な機能のみを公開できます。たとえば、スクーターを運転している人は、ホーンを押すと音が出るのを知っていますが、ホーンを押すと実際にどのように音が発生するのかわかりません。
ポリモーフィズム
ポリモーフィズムは多くの形態を意味します。つまり、物や行動はさまざまな形や方法で存在します。ポリモーフィズムの良い例の1つは、クラスでのコンストラクターのオーバーロードです。
オブジェクト指向Python
Pythonプログラミングの中心は object そして OOPただし、コードをクラスに編成することで、OOPの使用を制限する必要はありません。OOPは、Pythonの設計哲学全体に追加され、プログラミングのクリーンで実用的な方法を奨励します。OOPは、より大きく複雑なプログラムの作成も可能にします。
モジュールとクラスおよびオブジェクト
モジュールは「辞書」のようなものです
モジュールで作業するときは、次の点に注意してください-
Pythonモジュールは、再利用可能なコードをカプセル化するためのパッケージです。
モジュールは、 __init__.py その上にファイルします。
モジュールには関数とクラスが含まれています。
モジュールは、を使用してインポートされます import キーワード。
辞書は key-valueペア。つまり、キー付きの辞書がある場合EmployeID それを取得したい場合は、次のコード行を使用する必要があります-
employee = {“EmployeID”: “Employee Unique Identity!”}
print (employee [‘EmployeID])
次のプロセスでモジュールに取り組む必要があります-
モジュールは、いくつかの関数または変数を含むPythonファイルです。
必要なファイルをインポートします。
これで、そのモジュールの関数または変数に「。」を使用してアクセスできます。 (dot) オペレーター。
名前の付いたモジュールを考えてみましょう employee.py 呼び出された関数を含む employee。関数のコードを以下に示します-
# this goes in employee.py
def EmployeID():
print (“Employee Unique Identity!”)
モジュールをインポートしてから、関数にアクセスします EmployeID −
import employee
employee. EmployeID()
名前の付いた変数を挿入できます Age、示されているように-
def EmployeID():
print (“Employee Unique Identity!”)
# just a variable
Age = “Employee age is **”
ここで、次の方法でその変数にアクセスします-
import employee
employee.EmployeID()
print(employee.Age)
それでは、これを辞書と比較してみましょう-
Employee[‘EmployeID’] # get EmployeID from employee
Employee.employeID() # get employeID from the module
Employee.Age # get access to variable
Pythonには一般的なパターンがあることに注意してください-
取る key = value スタイルコンテナ
キーの名前でそれから何かを取得します
モジュールを辞書と比較する場合、次の点を除いて、両方とも類似しています。
の場合 dictionary、キーは文字列で、構文は[key]です。
の場合 module、キーは識別子であり、構文は.keyです。
クラスはモジュールのようなものです
モジュールは、Pythonコードを格納できる特殊な辞書であるため、「。」を使用してアクセスできます。オペレーター。クラスは、関数とデータのグループを取得してコンテナー内に配置し、「。」演算子を使用してそれらにアクセスできるようにする方法です。
従業員モジュールと同様のクラスを作成する必要がある場合は、次のコードを使用して作成できます-
class employee(object):
def __init__(self):
self. Age = “Employee Age is ##”
def EmployeID(self):
print (“This is just employee unique identity”)
Note−クラスは、モジュールをそのまま再利用でき、干渉をあまり受けないため、モジュールよりも優先されます。モジュールを使用している間は、プログラム全体で1つしかありません。
オブジェクトはミニインポートのようなものです
クラスは mini-module クラスの場合と同様の方法で、次の概念を使用してインポートできます。 instantiate。クラスをインスタンス化すると、object。
図のように、関数のようなクラスを呼び出すのと同じように、オブジェクトをインスタンス化できます。
this_obj = employee() # Instantiatethis_obj.EmployeID() # get EmployeId from the class
print(this_obj.Age) # get variable Age
これは、次の3つの方法のいずれかで実行できます-
# dictionary style
Employee[‘EmployeID’]
# module style
Employee.EmployeID()
Print(employee.Age)
# Class style
this_obj = employee()
this_obj.employeID()
Print(this_obj.Age)
この章では、ローカルコンピューターでのPython環境のセットアップについて詳しく説明します。
前提条件とツールキット
Pythonについてさらに学習する前に、次の前提条件が満たされているかどうかを確認することをお勧めします。
Pythonの最新バージョンがコンピューターにインストールされています
IDEまたはテキストエディタがインストールされている
Pythonでの記述とデバッグの基本的な知識があります。つまり、Pythonで次のことができます。
Pythonプログラムを作成して実行できる。
プログラムをデバッグし、エラーを診断します。
基本的なデータ型を操作します。
書く for ループ、 while ループ、および if ステートメント
コード functions
プログラミング言語の経験がない場合は、Pythonの初心者向けチュートリアルがたくさんあります。
https://www.tutorialpoints.com/Pythonのインストール
次の手順は、ローカルコンピュータにPythonをインストールする方法を詳しく示しています-
Step 1 −Pythonの公式Webサイトにアクセスします https://www.python.org/、 クリックしてください Downloads メニューを選択し、最新または安定したバージョンを選択します。

Step 2−ダウンロードしているPythonインストーラーexeファイルを保存し、ダウンロードしたら開きます。クリックRun と選択します Next デフォルトでオプションを選択し、インストールを終了します。

Step 3−インストールすると、下の画像に示すようなPythonメニューが表示されます。IDLE(Python GUI)を選択してプログラムを開始します。

これにより、Pythonシェルが起動します。簡単なコマンドを入力して、インストールを確認します。

IDEの選択
統合開発環境は、ソフトウェア開発を対象としたテキストエディタです。Pythonで作業する場合は、プログラミングのフローを制御し、プロジェクトをグループ化するためにIDEをインストールする必要があります。オンラインで利用できるIDEの一部を次に示します。ご都合の良いときにお選びいただけます。
- Pycharm IDE
- コモドIDE
- エリックPythonIDE
Note − Eclipse IDEは主にJavaで使用されますが、Pythonプラグインがあります。
Pycharm

クロスプラットフォームIDEであるPycharmは、現在利用可能な最も人気のあるIDEの1つです。コードの完成、プロジェクトとコードのナビゲーション、統合された単体テスト、バージョン管理の統合、デバッグなど、コーディングの支援と分析を提供します。
ダウンロードリンク
https://www.jetbrains.com/pycharm/download/#section=windowsLanguages Supported − Python、HTML、CSS、JavaScript、Coffee Script、TypeScript、Cython、AngularJS、Node.js、テンプレート言語。
スクリーンショット

なぜ選ぶのか?
PyCharmはユーザーに以下の機能と利点を提供します-
- Windows、Linux、およびMacOSと互換性のあるクロスプラットフォームIDE
- Django IDEに加えて、CSSとJavaScriptのサポートが含まれています
- 何千ものプラグイン、統合された端末およびバージョン管理が含まれています
- Git、SVN、Mercurialと統合
- Python用のインテリジェントな編集ツールを提供します
- Virtualenv、Docker、Vagrantとの簡単な統合
- シンプルなナビゲーションと検索機能
- コード分析とリファクタリング
- 構成可能な注入
- 大量のPythonライブラリをサポート
- テンプレートとJavaScriptデバッガーが含まれています
- Python / Djangoデバッガーが含まれています
- Google App Engine、追加のフレームワークおよびライブラリと連携します。
- カスタマイズ可能なUI、VIMエミュレーションが利用可能
コモドIDE

これは、100以上の言語をサポートし、基本的にPython、PHP、Rubyなどの動的言語をサポートするポリグロットIDEです。これは、全機能を備えた21日間の無料トライアルで利用できる商用IDEです。ActiveStateは、KomodoIDEの開発を管理するソフトウェア会社です。また、簡単なプログラミングタスク用にKomodoEditと呼ばれるKomodoのトリミングバージョンも提供しています。
このIDEには、最も基本的なレベルから高度なレベルまで、あらゆる種類の機能が含まれています。あなたが学生またはフリーランサーであるならば、あなたはそれを実際の価格のほぼ半分で買うことができます。ただし、公認の機関や大学の教師や教授は完全に無料です。
すべての言語とフレームワークのサポートを含む、Webおよびモバイル開発に必要なすべての機能を備えています。
ダウンロードリンク
Komodo Edit(無料版)とKomodo IDE(有料版)のダウンロードリンクは以下の通りです-
Komodo Edit (free)
https://www.activestate.com/komodo-editKomodo IDE (paid)
https://www.activestate.com/komodo-ide/downloads/ideスクリーンショット

なぜ選ぶのか?
- Perl、PHP、Python、Rubyなどをサポートする強力なIDE。
- クロスプラットフォームIDE。
これには、統合デバッガーサポート、オートコンプリート、ドキュメントオブジェクトモデル(DOM)ビューアー、コードブラウザー、インタラクティブシェル、ブレークポイント構成、コードプロファイリング、統合単体テストなどの基本機能が含まれています。要するに、それは生産性を高める多くの機能を備えたプロフェッショナルなIDEです。
エリックPythonIDE

PythonとRuby用のオープンソースIDEです。Ericは、Pythonで記述されたフル機能のエディターおよびIDEです。これは、クロスプラットフォームのQt GUIツールキットに基づいており、柔軟性の高いScintillaエディターコントロールを統合しています。IDEは非常に構成可能であり、使用するものと使用しないものを選択できます。以下のリンクからEricIDEをダウンロードできます。
https://eric-ide.python-projects.org/eric-download.html選ぶ理由
- 大きなインデント、エラーの強調表示。
- コード支援
- コード補完
- PyLintによるコードのクリーンアップ
- クイック検索
- 統合されたPythonデバッガー。
スクリーンショット

テキストエディタの選択
必ずしもIDEが必要なわけではありません。PythonやArduinoを使用したコーディングの学習などのタスクの場合、またはシェルスクリプトでクイックスクリプトを操作して一部のタスクを自動化する場合は、シンプルで軽量なコード中心のテキストエディターで実行できます。また、多くのテキストエディタは、IDEと同様に、構文の強調表示やプログラム内のスクリプト実行などの機能を提供します。テキストエディタの一部をここに示します-
- Atom
- 崇高なテキスト
- Notepad++
Atomテキストエディター

Atomは、GitHubのチームによって構築されたハッキング可能なテキストエディターです。これは無料のオープンソースのテキストおよびコードエディタです。つまり、すべてのコードを読んだり、自分で使用するために変更したり、改善に貢献したりすることができます。これは、macOS、Linux、およびMicrosoft Windowsと互換性のあるクロスプラットフォームのテキストエディターであり、Node.jsで記述されたプラグインと組み込みのGitControlをサポートしています。
ダウンロードリンク
https://atom.io/スクリーンショット
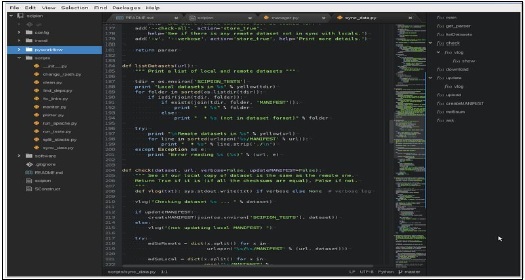
サポートされている言語
C / C ++、C#、CSS、CoffeeScript、HTML、JavaScript、Java、JSON、Julia、Objective-C、PHP、Perl、Python、Ruby on Rails、Ruby、Shellスクリプト、Scala、SQL、XML、YAMLなど。
崇高なテキストエディター

Sublime textはプロプライエタリソフトウェアであり、購入前にテストするための無料の試用版を提供しています。stackoverflow.comによると、これは4番目に人気のある開発環境です。
それが提供する利点のいくつかは、その信じられないほどの速度、使いやすさ、そしてコミュニティのサポートです。また、多くのプログラミング言語とマークアップ言語をサポートしており、プラグインを使用してユーザーが機能を追加できます。プラグインは通常、コミュニティで構築され、フリーソフトウェアライセンスの下で維持されます。
スクリーンショット

サポートされている言語
- Python、Ruby、JavaScriptなど。
なぜ選ぶのか?
キーバインディング、メニュー、スニペット、マクロ、補完などをカスタマイズします。
オートコンプリート機能
- スニペット、フィールドマーカー、プレースホルダーを使用して、崇高なテキストスニペットを含むテキストとコードをすばやく挿入します
すばやく開きます
Mac、Linux、Windowsのクロスプラットフォームサポート。
行きたい場所にカーソルをジャンプします
複数の行、単語、列を選択する
メモ帳++

これは無料のソースコードエディタであり、メモ帳に代わるものであり、アセンブリからXMLまで、Pythonを含むいくつかの言語をサポートしています。MS Windows環境で実行され、その使用はGPLライセンスによって管理されます。構文の強調表示に加えて、Notepad ++にはコーダーにとって特に便利な機能がいくつかあります。
スクリーンショット

主な機能
- 構文の強調表示と構文の折りたたみ
- PCRE(Perl互換正規表現)検索/置換
- 完全にカスタマイズ可能なGUI
- S自動完了
- タブ付き編集
- Multi-View
- 多言語環境
- さまざまな引数で起動可能
サポートされる言語
- Python、C、C ++、C#、Javaなどのほぼすべての言語(60以上の言語)。
Pythonデータ構造は、構文の観点から非常に直感的であり、操作の選択肢が豊富です。Pythonデータ構造は、データの内容、変更が必要かどうか、固定データであり、開始/終了/ランダムなど、必要なアクセスタイプに応じて選択する必要があります。
リスト
リストは、Pythonで最も用途の広いタイプのデータ構造を表します。リストは、角かっこで囲まれたコンマ区切りの値(項目または要素)を保持するコンテナーです。リストは、複数の関連する値を処理する場合に役立ちます。リストはデータをまとめて保持するため、一度に複数の値に対して同じメソッドと操作を実行できます。リストのインデックスはゼロから始まり、文字列とは異なり、リストは変更可能です。
データ構造-リスト
>>>
>>> # Any Empty List
>>> empty_list = []
>>>
>>> # A list of String
>>> str_list = ['Life', 'Is', 'Beautiful']
>>> # A list of Integers
>>> int_list = [1, 4, 5, 9, 18]
>>>
>>> #Mixed items list
>>> mixed_list = ['This', 9, 'is', 18, 45.9, 'a', 54, 'mixed', 99, 'list']
>>> # To print the list
>>>
>>> print(empty_list)
[]
>>> print(str_list)
['Life', 'Is', 'Beautiful']
>>> print(type(str_list))
<class 'list'>
>>> print(int_list)
[1, 4, 5, 9, 18]
>>> print(mixed_list)
['This', 9, 'is', 18, 45.9, 'a', 54, 'mixed', 99, 'list']
Pythonリストのアイテムへのアクセス
リストの各項目には番号が割り当てられます。つまり、その番号のインデックスまたは位置です。インデックスは常に0から始まり、2番目のインデックスは1というように続きます。リスト内のアイテムにアクセスするには、角括弧内でこれらのインデックス番号を使用できます。たとえば、次のコードを確認してください。
>>> mixed_list = ['This', 9, 'is', 18, 45.9, 'a', 54, 'mixed', 99, 'list']
>>>
>>> # To access the First Item of the list
>>> mixed_list[0]
'This'
>>> # To access the 4th item
>>> mixed_list[3]
18
>>> # To access the last item of the list
>>> mixed_list[-1]
'list'
空のオブジェクト
空のオブジェクトは、最も単純で最も基本的なPython組み込み型です。気付かずに何度も使用し、作成したすべてのクラスに拡張しました。空のクラスを作成する主な目的は、当面は何かをブロックし、後で拡張して動作を追加することです。
クラスに動作を追加するということは、データ構造をオブジェクトに置き換え、そのオブジェクトへのすべての参照を変更することを意味します。したがって、何かを作成する前に、データが偽装されたオブジェクトであるかどうかを確認することが重要です。理解を深めるために、次のコードを確認してください。
>>> #Empty objects
>>>
>>> obj = object()
>>> obj.x = 9
Traceback (most recent call last):
File "<pyshell#3>", line 1, in <module>
obj.x = 9
AttributeError: 'object' object has no attribute 'x'
したがって、上から、直接インスタンス化されたオブジェクトに属性を設定することはできないことがわかります。Pythonがオブジェクトに任意の属性を持つことを許可する場合、属性名とその値の両方を格納するために、各オブジェクトが持つ属性を追跡するために一定量のシステムメモリが必要です。属性が格納されていない場合でも、潜在的な新しい属性に一定量のメモリが割り当てられます。
そのため、Pythonは、デフォルトで、オブジェクトおよびその他のいくつかの組み込みの任意のプロパティを無効にします。
>>> # Empty Objects
>>>
>>> class EmpObject:
pass
>>> obj = EmpObject()
>>> obj.x = 'Hello, World!'
>>> obj.x
'Hello, World!'
したがって、プロパティをグループ化する場合は、上記のコードに示すように、それらを空のオブジェクトに格納できます。ただし、この方法が常に推奨されるわけではありません。クラスとオブジェクトは、データと動作の両方を指定する場合にのみ使用する必要があることに注意してください。
タプル
タプルはリストに似ており、要素を格納できます。ただし、これらは不変であるため、オブジェクトを追加、削除、または置換することはできません。タプルがその不変性のために提供する主な利点は、辞書のキーとして、またはオブジェクトがハッシュ値を必要とする他の場所でそれらを使用できることです。
タプルは、動作ではなくデータを格納するために使用されます。タプルを操作するための動作が必要な場合は、アクションを実行する関数(または別のオブジェクトのメソッド)にタプルを渡す必要があります。
タプルは辞書キーとして機能できるため、格納される値は互いに異なります。値をコンマで区切ることでタプルを作成できます。タプルは括弧で囲まれていますが、必須ではありません。次のコードは、2つの同一の割り当てを示しています。
>>> stock1 = 'MSFT', 95.00, 97.45, 92.45
>>> stock2 = ('MSFT', 95.00, 97.45, 92.45)
>>> type (stock1)
<class 'tuple'>
>>> type(stock2)
<class 'tuple'>
>>> stock1 == stock2
True
>>>
タプルの定義
タプルはリストと非常によく似ていますが、要素のセット全体が角かっこではなく括弧で囲まれている点が異なります。
リストをスライスするときと同じように、新しいリストを取得し、タプルをスライスすると、新しいタプルを取得します。
>>> tupl = ('Tuple','is', 'an','IMMUTABLE', 'list')
>>> tupl
('Tuple', 'is', 'an', 'IMMUTABLE', 'list')
>>> tupl[0]
'Tuple'
>>> tupl[-1]
'list'
>>> tupl[1:3]
('is', 'an')
Pythonタプルメソッド
次のコードは、Pythonタプルのメソッドを示しています-
>>> tupl
('Tuple', 'is', 'an', 'IMMUTABLE', 'list')
>>> tupl.append('new')
Traceback (most recent call last):
File "<pyshell#148>", line 1, in <module>
tupl.append('new')
AttributeError: 'tuple' object has no attribute 'append'
>>> tupl.remove('is')
Traceback (most recent call last):
File "<pyshell#149>", line 1, in <module>
tupl.remove('is')
AttributeError: 'tuple' object has no attribute 'remove'
>>> tupl.index('list')
4
>>> tupl.index('new')
Traceback (most recent call last):
File "<pyshell#151>", line 1, in <module>
tupl.index('new')
ValueError: tuple.index(x): x not in tuple
>>> "is" in tupl
True
>>> tupl.count('is')
1
上記のコードから、タプルは不変であり、したがって−であることが理解できます。
君は cannot タプルに要素を追加します。
君は cannot メソッドを追加または拡張します。
君は cannot タプルから要素を削除します。
タプルは no removeまたはpopメソッド。
カウントとインデックスは、タプルで使用できるメソッドです。
辞書
ディクショナリはPythonの組み込みデータ型の1つであり、キーと値の間の1対1の関係を定義します。
辞書の定義
次のコードを観察して、辞書の定義について理解してください。
>>> # empty dictionary
>>> my_dict = {}
>>>
>>> # dictionary with integer keys
>>> my_dict = { 1:'msft', 2: 'IT'}
>>>
>>> # dictionary with mixed keys
>>> my_dict = {'name': 'Aarav', 1: [ 2, 4, 10]}
>>>
>>> # using built-in function dict()
>>> my_dict = dict({1:'msft', 2:'IT'})
>>>
>>> # From sequence having each item as a pair
>>> my_dict = dict([(1,'msft'), (2,'IT')])
>>>
>>> # Accessing elements of a dictionary
>>> my_dict[1]
'msft'
>>> my_dict[2]
'IT'
>>> my_dict['IT']
Traceback (most recent call last):
File "<pyshell#177>", line 1, in <module>
my_dict['IT']
KeyError: 'IT'
>>>
上記のコードから、次のことがわかります。
まず、2つの要素を持つ辞書を作成し、それを変数に割り当てます my_dict。各要素はキーと値のペアであり、要素のセット全体が中括弧で囲まれています。
番号 1 キーであり、 msftその値です。同様に、2 キーであり、 IT その値です。
キーで値を取得できますが、その逆はできません。したがって、私たちが試みるときmy_dict[‘IT’] 、例外が発生します。 IT キーではありません。
辞書の変更
次のコードを観察して、辞書の変更について理解してください。
>>> # Modifying a Dictionary
>>>
>>> my_dict
{1: 'msft', 2: 'IT'}
>>> my_dict[2] = 'Software'
>>> my_dict
{1: 'msft', 2: 'Software'}
>>>
>>> my_dict[3] = 'Microsoft Technologies'
>>> my_dict
{1: 'msft', 2: 'Software', 3: 'Microsoft Technologies'}
上記のコードから、次のことがわかります。
辞書に重複するキーを含めることはできません。既存のキーの値を変更すると、古い値が削除されます。
新しいキーと値のペアはいつでも追加できます。
辞書には、要素間の順序の概念がありません。それらは単純な順序付けられていないコレクションです。
辞書でのデータ型の混合
次のコードを観察して、辞書でのデータ型の混合について理解してください。
>>> # Mixing Data Types in a Dictionary
>>>
>>> my_dict
{1: 'msft', 2: 'Software', 3: 'Microsoft Technologies'}
>>> my_dict[4] = 'Operating System'
>>> my_dict
{1: 'msft', 2: 'Software', 3: 'Microsoft Technologies', 4: 'Operating System'}
>>> my_dict['Bill Gates'] = 'Owner'
>>> my_dict
{1: 'msft', 2: 'Software', 3: 'Microsoft Technologies', 4: 'Operating System',
'Bill Gates': 'Owner'}
上記のコードから、次のことがわかります。
文字列だけでなく、辞書の値は、辞書自体を含む、文字列、整数を含む任意のデータ型にすることができます。
ディクショナリ値とは異なり、ディクショナリキーはより制限されていますが、文字列、整数などの任意のタイプにすることができます。
辞書からのアイテムの削除
次のコードを観察して、辞書からのアイテムの削除について理解してください。
>>> # Deleting Items from a Dictionary
>>>
>>> my_dict
{1: 'msft', 2: 'Software', 3: 'Microsoft Technologies', 4: 'Operating System',
'Bill Gates': 'Owner'}
>>>
>>> del my_dict['Bill Gates']
>>> my_dict
{1: 'msft', 2: 'Software', 3: 'Microsoft Technologies', 4: 'Operating System'}
>>>
>>> my_dict.clear()
>>> my_dict
{}
上記のコードから、次のことがわかります。
del −キーを使用して辞書から個々のアイテムを削除できます。
clear −辞書からすべてのアイテムを削除します。
セット
Set()は、重複する要素のない順序付けられていないコレクションです。個々のアイテムは不変ですが、セット自体は変更可能です。つまり、要素/アイテムをセットに追加したり、セットから削除したりできます。セットを使用して、和集合、交差などの数学演算を実行できます。
セットは一般にツリーを使用して実装できますが、Pythonでのセットはハッシュテーブルを使用して実装できます。これにより、特定の要素がセットに含まれているかどうかを確認するための高度に最適化された方法が可能になります
セットの作成
セットは、すべてのアイテム(要素)を中括弧内に配置することによって作成されます {}、コンマで区切るか、組み込み関数を使用します set()。次のコード行を確認してください-
>>> #set of integers
>>> my_set = {1,2,4,8}
>>> print(my_set)
{8, 1, 2, 4}
>>>
>>> #set of mixed datatypes
>>> my_set = {1.0, "Hello World!", (2, 4, 6)}
>>> print(my_set)
{1.0, (2, 4, 6), 'Hello World!'}
>>>
セットのメソッド
セットのメソッドについて理解するには、次のコードを観察してください-
>>> >>> #METHODS FOR SETS
>>>
>>> #add(x) Method
>>> topics = {'Python', 'Java', 'C#'}
>>> topics.add('C++')
>>> topics
{'C#', 'C++', 'Java', 'Python'}
>>>
>>> #union(s) Method, returns a union of two set.
>>> topics
{'C#', 'C++', 'Java', 'Python'}
>>> team = {'Developer', 'Content Writer', 'Editor','Tester'}
>>> group = topics.union(team)
>>> group
{'Tester', 'C#', 'Python', 'Editor', 'Developer', 'C++', 'Java', 'Content
Writer'}
>>> # intersets(s) method, returns an intersection of two sets
>>> inters = topics.intersection(team)
>>> inters
set()
>>>
>>> # difference(s) Method, returns a set containing all the elements of
invoking set but not of the second set.
>>>
>>> safe = topics.difference(team)
>>> safe
{'Python', 'C++', 'Java', 'C#'}
>>>
>>> diff = topics.difference(group)
>>> diff
set()
>>> #clear() Method, Empties the whole set.
>>> group.clear()
>>> group
set()
>>>
セットの演算子
セットの演算子について理解するには、次のコードを参照してください-
>>> # PYTHON SET OPERATIONS
>>>
>>> #Creating two sets
>>> set1 = set()
>>> set2 = set()
>>>
>>> # Adding elements to set
>>> for i in range(1,5):
set1.add(i)
>>> for j in range(4,9):
set2.add(j)
>>> set1
{1, 2, 3, 4}
>>> set2
{4, 5, 6, 7, 8}
>>>
>>> #Union of set1 and set2
>>> set3 = set1 | set2 # same as set1.union(set2)
>>> print('Union of set1 & set2: set3 = ', set3)
Union of set1 & set2: set3 = {1, 2, 3, 4, 5, 6, 7, 8}
>>>
>>> #Intersection of set1 & set2
>>> set4 = set1 & set2 # same as set1.intersection(set2)
>>> print('Intersection of set1 and set2: set4 = ', set4)
Intersection of set1 and set2: set4 = {4}
>>>
>>> # Checking relation between set3 and set4
>>> if set3 > set4: # set3.issuperset(set4)
print('Set3 is superset of set4')
elif set3 < set4: #set3.issubset(set4)
print('Set3 is subset of set4')
else: #set3 == set4
print('Set 3 is same as set4')
Set3 is superset of set4
>>>
>>> # Difference between set3 and set4
>>> set5 = set3 - set4
>>> print('Elements in set3 and not in set4: set5 = ', set5)
Elements in set3 and not in set4: set5 = {1, 2, 3, 5, 6, 7, 8}
>>>
>>> # Check if set4 and set5 are disjoint sets
>>> if set4.isdisjoint(set5):
print('Set4 and set5 have nothing in common\n')
Set4 and set5 have nothing in common
>>> # Removing all the values of set5
>>> set5.clear()
>>> set5 set()
この章では、オブジェクト指向の用語とプログラミングの概念について詳しく説明します。クラスは、インスタンスの単なるファクトリです。このファクトリには、インスタンスの作成方法を説明するブループリントが含まれています。インスタンスまたはオブジェクトは、クラスから構築されます。ほとんどの場合、クラスのインスタンスは複数持つことができます。すべてのインスタンスには一連の属性があり、これらの属性はクラスで定義されているため、特定のクラスのすべてのインスタンスは同じ属性を持つことが期待されます。
クラスバンドル:動作と状態
クラスを使用すると、オブジェクトの動作と状態をまとめることができます。理解を深めるために、次の図を参照してください。
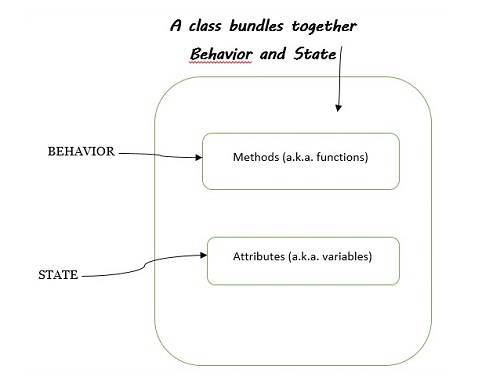
クラスバンドルについて説明する場合、次の点に注意してください。
言葉 behavior と同じです function –それは何かをする(または振る舞いを実装する)コードの一部です
言葉 state と同じです variables –クラス内に値を格納する場所です。
クラスの振る舞いと状態を一緒に主張するとき、それはクラスが関数と変数をパッケージ化することを意味します。
クラスにはメソッドと属性があります
Pythonでは、メソッドを作成するとクラスの動作が定義されます。メソッドという言葉は、クラス内で定義されている関数に付けられたOOP名です。まとめると-
Class functions −はの同義語です methods
Class variables −はの同義語です name attributes.
Class −正確な動作をするインスタンスの青写真。
Object −クラスのインスタンスの1つで、クラスで定義された機能を実行します。
Type −インスタンスが属するクラスを示します
Attribute −任意のオブジェクト値:object.attribute
Method −クラスで定義された「呼び出し可能な属性」
たとえば、次のコードを観察します。
var = “Hello, John”
print( type (var)) # < type ‘str’> or <class 'str'>
print(var.upper()) # upper() method is called, HELLO, JOHN
作成とインスタンス化
次のコードは、最初のクラスを作成し、次にそのインスタンスを作成する方法を示しています。
class MyClass(object):
pass
# Create first instance of MyClass
this_obj = MyClass()
print(this_obj)
# Another instance of MyClass
that_obj = MyClass()
print (that_obj)
ここで、というクラスを作成しました MyClassそしてそれは何の仕事もしません。議論object に MyClass クラスにはクラスの継承が含まれ、後の章で説明します。 pass 上記のコードでは、このブロックが空であること、つまり空のクラス定義であることを示しています。
インスタンスを作成しましょう this_obj の MyClass() クラスを作成し、図のように印刷します-
<__main__.MyClass object at 0x03B08E10>
<__main__.MyClass object at 0x0369D390>
ここでは、のインスタンスを作成しました MyClass.16進コードは、オブジェクトが格納されているアドレスを参照します。別のインスタンスが別のアドレスを指しています。
次に、クラス内で1つの変数を定義しましょう MyClass() 次のコードに示すように、そのクラスのインスタンスから変数を取得します-
class MyClass(object):
var = 9
# Create first instance of MyClass
this_obj = MyClass()
print(this_obj.var)
# Another instance of MyClass
that_obj = MyClass()
print (that_obj.var)
出力
上記のコードを実行すると、次の出力が表示されます。
9
9
インスタンスはインスタンス化されたクラスを認識しているため、インスタンスから属性を要求されると、インスタンスは属性とクラスを探します。これは、attribute lookup.
インスタンスメソッド
クラスで定義された関数は、 method.インスタンスメソッドは、それを呼び出すためにインスタンスを必要とし、デコレータを必要としません。インスタンスメソッドを作成する場合、最初のパラメータは常にself. 他の名前で(self)と呼ぶこともできますが、命名規則であるため、selfを使用することをお勧めします。
class MyClass(object):
var = 9
def firstM(self):
print("hello, World")
obj = MyClass()
print(obj.var)
obj.firstM()
出力
上記のコードを実行すると、次の出力が表示されます。
9
hello, World
上記のプログラムでは、selfを引数としてメソッドを定義していることに注意してください。ただし、引数を宣言していないため、メソッドを呼び出すことはできません。
class MyClass(object):
def firstM(self):
print("hello, World")
print(self)
obj = MyClass()
obj.firstM()
print(obj)
出力
上記のコードを実行すると、次の出力が表示されます。
hello, World
<__main__.MyClass object at 0x036A8E10>
<__main__.MyClass object at 0x036A8E10>
カプセル化
カプセル化は、OOPの基本の1つです。OOPを使用すると、オブジェクトの内部動作の複雑さを隠すことができます。これは、次の方法で開発者にとって有利です。
内部を知らなくてもオブジェクトを使用することを単純化し、理解しやすくします。
変更は簡単に管理できます。
オブジェクト指向プログラミングは、カプセル化に大きく依存しています。カプセル化と抽象化(データ隠蔽とも呼ばれます)という用語は、同義語としてよく使用されます。抽象化はカプセル化によって実現されるため、これらはほぼ同義です。
カプセル化は、オブジェクトのコンポーネントの一部へのアクセスを制限するメカニズムを提供します。これは、オブジェクトの内部表現がオブジェクト定義の外部からは見えないことを意味します。このデータへのアクセスは、通常、特別な方法で行われます。Getters そして Setters.
このデータはインスタンス属性に格納され、クラス外のどこからでも操作できます。それを保護するには、そのデータはインスタンスメソッドを使用してのみアクセスする必要があります。直接アクセスは許可しないでください。
class MyClass(object):
def setAge(self, num):
self.age = num
def getAge(self):
return self.age
zack = MyClass()
zack.setAge(45)
print(zack.getAge())
zack.setAge("Fourty Five")
print(zack.getAge())
出力
上記のコードを実行すると、次の出力が表示されます。
45
Fourty Five
データは、例外処理構造を使用して、正しく有効な場合にのみ保存する必要があります。上記のように、setAge()メソッドへのユーザー入力に制限はありません。文字列、数値、またはリストの場合があります。したがって、上記のコードをチェックして、保存の正確さを確認する必要があります。
class MyClass(object):
def setAge(self, num):
self.age = num
def getAge(self):
return self.age
zack = MyClass()
zack.setAge(45)
print(zack.getAge())
zack.setAge("Fourty Five")
print(zack.getAge())
Initコンストラクタ
__init__メソッドは、クラスのオブジェクトがインスタンス化されるとすぐに暗黙的に呼び出されます。これにより、オブジェクトが初期化されます。
x = MyClass()
上記のコード行は、新しいインスタンスを作成し、このオブジェクトをローカル変数xに割り当てます。
インスタンス化操作、つまり calling a class object、空のオブジェクトを作成します。多くのクラスは、特定の初期状態にカスタマイズされたインスタンスを使用してオブジェクトを作成することを好みます。したがって、クラスは、次のように「__init __()」という名前の特別なメソッドを定義できます。
def __init__(self):
self.data = []
Pythonは、インスタンス化中に__init__を呼び出して、クラスがインスタンス化されたときに発生する追加の属性を定義します。この属性は、そのオブジェクトの開始値を設定したり、インスタンス化に必要なルーチンを実行したりする場合があります。したがって、この例では、新しい初期化されたインスタンスを次のように取得できます。
x = MyClass()
__init __()メソッドは、柔軟性を高めるために単一または複数の引数を持つことができます。initは、インスタンスの属性を初期化するため、初期化を表します。これは、クラスのコンストラクターと呼ばれます。
class myclass(object):
def __init__(self,aaa, bbb):
self.a = aaa
self.b = bbb
x = myclass(4.5, 3)
print(x.a, x.b)
出力
4.5 3
クラス属性
クラスで定義された属性は「クラス属性」と呼ばれ、関数で定義された属性は「インスタンス属性」と呼ばれます。定義中、これらの属性はクラスのプロパティであり、特定のインスタンスのプロパティではないため、接頭辞はselfではありません。
クラス属性には、クラス自体(className.attributeName)と、クラスのインスタンス(inst.attributeName)からアクセスできます。したがって、インスタンスはインスタンス属性とクラス属性の両方にアクセスできます。
>>> class myclass():
age = 21
>>> myclass.age
21
>>> x = myclass()
>>> x.age
21
>>>
クラス属性は、カプセル化を解除するのに適した方法ではありませんが、インスタンスでオーバーライドできます。
Pythonには属性のルックアップパスがあります。最初はクラス内で定義されたメソッドであり、次にその上のクラスです。
>>> class myclass(object):
classy = 'class value'
>>> dd = myclass()
>>> print (dd.classy) # This should return the string 'class value'
class value
>>>
>>> dd.classy = "Instance Value"
>>> print(dd.classy) # Return the string "Instance Value"
Instance Value
>>>
>>> # This will delete the value set for 'dd.classy' in the instance.
>>> del dd.classy
>>> >>> # Since the overriding attribute was deleted, this will print 'class
value'.
>>> print(dd.classy)
class value
>>>
インスタンスddの「classy」クラス属性をオーバーライドしています。オーバーライドされると、Pythonインタープリターはオーバーライドされた値を読み取ります。ただし、新しい値が「del」で削除されると、オーバーライドされた値はインスタンスに存在しなくなるため、ルックアップは1レベル上に移動し、クラスから取得します。
クラスおよびインスタンスデータの操作
このセクションでは、クラスデータがインスタンスデータにどのように関連しているかを理解しましょう。データはクラスまたはインスタンスのいずれかに保存できます。クラスを設計するとき、どのデータがインスタンスに属し、どのデータをクラス全体に格納するかを決定します。
インスタンスはクラスデータにアクセスできます。複数のインスタンスを作成する場合、これらのインスタンスは、クラスデータ全体だけでなく個々の属性値にもアクセスできます。
したがって、クラスデータは、すべてのインスタンス間で共有されるデータです。より良いアンダーサンディングのために以下に与えられたコードを観察してください-
class InstanceCounter(object):
count = 0 # class attribute, will be accessible to all instances
def __init__(self, val):
self.val = val
InstanceCounter.count +=1 # Increment the value of class attribute, accessible through class name
# In above line, class ('InstanceCounter') act as an object
def set_val(self, newval):
self.val = newval
def get_val(self):
return self.val
def get_count(self):
return InstanceCounter.count
a = InstanceCounter(9)
b = InstanceCounter(18)
c = InstanceCounter(27)
for obj in (a, b, c):
print ('val of obj: %s' %(obj.get_val())) # Initialized value ( 9, 18, 27)
print ('count: %s' %(obj.get_count())) # always 3
出力
val of obj: 9
count: 3
val of obj: 18
count: 3
val of obj: 27
count: 3
つまり、クラス属性はクラスのすべてのインスタンスで同じですが、インスタンス属性は各インスタンスに固有です。2つの異なるインスタンスの場合、2つの異なるインスタンス属性があります。
class myClass:
class_attribute = 99
def class_method(self):
self.instance_attribute = 'I am instance attribute'
print (myClass.__dict__)
出力
上記のコードを実行すると、次の出力が表示されます。
{'__module__': '__main__', 'class_attribute': 99, 'class_method': <function myClass.class_method at 0x04128D68>, '__dict__': <attribute '__dict__' of 'myClass' objects>, '__weakref__': <attribute '__weakref__' of 'myClass' objects>, '__doc__': None}
インスタンス属性 myClass.__dict__ 示されているように-
>>> a = myClass()
>>> a.class_method()
>>> print(a.__dict__)
{'instance_attribute': 'I am instance attribute'}
この章では、Pythonのさまざまな組み込み関数、ファイルI / O操作、およびオーバーロードの概念について詳しく説明します。
Python組み込み関数
Pythonインタープリターには、すぐに使用できる組み込み関数と呼ばれる関数がいくつかあります。最新バージョンでは、Pythonには以下の表にリストされている68の組み込み関数が含まれています-
組み込み関数 | ||||
---|---|---|---|---|
abs() | dict() | 助けて() | min() | setattr() |
すべて() | dir() | hex() | 次() | スライス() |
どれか() | divmod() | id() | object() | ソート済み() |
ascii() | enumerate() | 入力() | oct() | staticmethod() |
置き場() | eval() | int() | 開いた() | str() |
bool() | exec() | isinstance() | ord() | 和() |
bytearray() | フィルタ() | issubclass() | 捕虜() | 素晴らしい() |
bytes() | 浮く() | iter() | print() | tuple() |
callable() | フォーマット() | len() | property() | タイプ() |
chr() | 凍結セット() | リスト() | 範囲() | vars() |
classmethod() | getattr() | locals() | repr() | zip() |
コンパイル() | globals() | 地図() | reverse() | __インポート__() |
繁雑() | hasattr() | max() | 円形() | |
delattr() | ハッシュ() | memoryview() | セットする() |
このセクションでは、いくつかの重要な機能について簡単に説明します。
len()関数
len()関数は、文字列、リスト、またはコレクションの長さを取得します。オブジェクトのアイテムの長さまたは数を返します。オブジェクトは文字列、リスト、またはコレクションです。
>>> len(['hello', 9 , 45.0, 24])
4
len()関数は内部的に次のように機能します list.__len__() または tuple.__len__()。したがって、len()は__を持つオブジェクトでのみ機能することに注意してくださいlen__() 方法。
>>> set1
{1, 2, 3, 4}
>>> set1.__len__()
4
ただし、実際には、 len() の代わりに __len__() 以下の理由で機能する−
それはより効率的です。また、__ len__などの特別なメソッドへのアクセスを拒否するために特定のメソッドを作成する必要はありません。
メンテナンスが簡単です。
下位互換性をサポートします。
反転(seq)
逆イテレータを返します。seqは、__ reverse __()メソッドを持つオブジェクト、またはシーケンスプロトコル(__len __()メソッドと__getitem __()メソッド)をサポートするオブジェクトである必要があります。それは一般的に使用されますfor アイテムを後ろから前にループしたいときにループします。
>>> normal_list = [2, 4, 5, 7, 9]
>>>
>>> class CustomSequence():
def __len__(self):
return 5
def __getitem__(self,index):
return "x{0}".format(index)
>>> class funkyback():
def __reversed__(self):
return 'backwards!'
>>> for seq in normal_list, CustomSequence(), funkyback():
print('\n{}: '.format(seq.__class__.__name__), end="")
for item in reversed(seq):
print(item, end=", ")
最後のforループは、通常のリストの反転リストと、2つのカスタムシーケンスのインスタンスを出力します。出力は次のことを示していますreversed() 3つすべてで機能しますが、定義すると結果が大きく異なります。 __reversed__。
出力
上記のコードを実行すると、次の出力が表示されます。
list: 9, 7, 5, 4, 2,
CustomSequence: x4, x3, x2, x1, x0,
funkyback: b, a, c, k, w, a, r, d, s, !,
列挙する
ザ・ enumerate () メソッドは、反復可能オブジェクトにカウンターを追加し、列挙オブジェクトを返します。
enumerate()の構文は次のとおりです。
enumerate(iterable, start = 0)
ここで2番目の引数 start オプションであり、デフォルトではインデックスはゼロ(0)で始まります。
>>> # Enumerate
>>> names = ['Rajesh', 'Rahul', 'Aarav', 'Sahil', 'Trevor']
>>> enumerate(names)
<enumerate object at 0x031D9F80>
>>> list(enumerate(names))
[(0, 'Rajesh'), (1, 'Rahul'), (2, 'Aarav'), (3, 'Sahil'), (4, 'Trevor')]
>>>
そう enumerate()渡されたシーケンス内の要素のカウントを保持するタプルを生成するイテレータを返します。戻り値はイテレータであるため、直接アクセスすることはあまり役に立ちません。enumerate()のより良いアプローチは、forループ内でカウントを維持することです。
>>> for i, n in enumerate(names):
print('Names number: ' + str(i))
print(n)
Names number: 0
Rajesh
Names number: 1
Rahul
Names number: 2
Aarav
Names number: 3
Sahil
Names number: 4
Trevor
標準ライブラリには他にも多くの関数があります。これは、より広く使用されている関数の別のリストです。
hasattr, getattr, setattr そして delattr, これにより、オブジェクトの属性を文字列名で操作できます。
all そして any, 反復可能なオブジェクトを受け入れて返す True すべてまたはいずれかの項目が真であると評価された場合。
nzip, これは、2つ以上のシーケンスを取り、タプルの新しいシーケンスを返します。各タプルには、各シーケンスからの単一の値が含まれます。
ファイルI / O
ファイルの概念は、オブジェクト指向プログラミングという用語に関連しています。Pythonは、オペレーティングシステムが抽象化して提供するインターフェイスをラップし、ファイルオブジェクトを操作できるようにしました。
ザ・ open()組み込み関数は、ファイルを開いてファイルオブジェクトを返すために使用されます。これは、2つの引数を持つ最も一般的に使用される関数です-
open(filename, mode)
open()関数は2つの引数を呼び出します。最初はファイル名で、2番目はモードです。ここで、モードは読み取り専用モードの場合は「r」、書き込み専用の場合は「w」(同じ名前の既存のファイルは消去されます)、「a」は追加用にファイルを開き、ファイルに書き込まれたデータはすべて自動的に追加されます。最後まで。'r +'は、読み取りと書き込みの両方のためにファイルを開きます。デフォルトモードは読み取り専用です。
Windowsでは、モードに「b」を追加するとファイルがバイナリモードで開くため、「rb」、「wb」、「r + b」などのモードもあります。
>>> text = 'This is the first line'
>>> file = open('datawork','w')
>>> file.write(text)
22
>>> file.close()
場合によっては、既存のファイルを完全に上書きするのではなく、ファイルの最後に追加するために、モード引数として値 'a'を指定できるため、既存のファイルを上書きするのではなく、単に追加する必要があります。内容。
>>> f = open('datawork','a')
>>> text1 = ' This is second line'
>>> f.write(text1)
20
>>> f.close()
ファイルが読み取り用に開かれると、read、readline、またはreadlinesメソッドを呼び出してファイルの内容を取得できます。readメソッドは、2番目の引数が「b」であるかどうかに応じて、ファイルの内容全体をstrまたはbytesオブジェクトとして返します。
読みやすくするため、また大きなファイルを一度に読み取らないようにするために、ファイルオブジェクトで直接forループを使用する方がよい場合がよくあります。テキストファイルの場合、各行を一度に1つずつ読み取り、ループ本体内で処理できます。ただし、バイナリファイルの場合は、read()メソッドを使用して固定サイズのデータチャンクを読み取り、読み取る最大バイト数のパラメータを渡すことをお勧めします。
>>> f = open('fileone','r+')
>>> f.readline()
'This is the first line. \n'
>>> f.readline()
'This is the second line. \n'
ファイルオブジェクトのwriteメソッドを介してファイルに書き込むと、文字列(バイナリデータの場合はバイト)オブジェクトがファイルに書き込まれます。writelinesメソッドは一連の文字列を受け入れ、繰り返された各値をファイルに書き込みます。writelinesメソッドは、シーケンスの各項目の後に改行を追加しません。
最後に、ファイルの読み取りまたは書き込みが終了したら、close()メソッドを呼び出して、バッファリングされた書き込みがディスクに書き込まれ、ファイルが適切にクリーンアップされ、ファイルに関連付けられているすべてのリソースが解放されていることを確認する必要があります。オペレーティングシステム。close()メソッドを呼び出す方が良いアプローチですが、技術的には、スクリプトが存在するときにこれが自動的に行われます。
メソッドのオーバーロードの代替
メソッドのオーバーロードとは、異なる引数のセットを受け入れる同じ名前の複数のメソッドを持つことを指します。
単一のメソッドまたは関数が与えられた場合、パラメーターの数を自分で指定できます。関数の定義に応じて、0、1、2、またはそれ以上のパラメーターを使用して呼び出すことができます。
class Human:
def sayHello(self, name = None):
if name is not None:
print('Hello ' + name)
else:
print('Hello ')
#Create Instance
obj = Human()
#Call the method, else part will be executed
obj.sayHello()
#Call the method with a parameter, if part will be executed
obj.sayHello('Rahul')
出力
Hello
Hello Rahul
デフォルトの引数
関数もオブジェクトです
呼び出し可能オブジェクトは、オブジェクトがいくつかの引数を受け入れることができ、場合によってはオブジェクトを返すことです。関数はPythonで最も単純な呼び出し可能なオブジェクトですが、クラスや特定のクラスインスタンスのようなものもあります。
Pythonのすべての関数はオブジェクトです。オブジェクトにはメソッドまたは関数を含めることができますが、オブジェクトは関数である必要はありません。
def my_func():
print('My function was called')
my_func.description = 'A silly function'
def second_func():
print('Second function was called')
second_func.description = 'One more sillier function'
def another_func(func):
print("The description:", end=" ")
print(func.description)
print('The name: ', end=' ')
print(func.__name__)
print('The class:', end=' ')
print(func.__class__)
print("Now I'll call the function passed in")
func()
another_func(my_func)
another_func(second_func)
上記のコードでは、2つの異なる関数を引数として3番目の関数に渡し、それぞれに対して異なる出力を取得できます。
The description: A silly function
The name: my_func
The class:
Now I'll call the function passed in My function was called The description: One more sillier function The name: second_func The class:
Now I'll call the function passed in Second function was called
callable objects
Just as functions are objects that can have attributes set on them, it is possible to create an object that can be called as though it were a function.
In Python any object with a __call__() method can be called using function-call syntax.
Inheritance and Polymorphism
Inheritance and polymorphism – this is a very important concept in Python. You must understand it better if you want to learn.
Inheritance
One of the major advantages of Object Oriented Programming is re-use. Inheritance is one of the mechanisms to achieve the same. Inheritance allows programmer to create a general or a base class first and then later extend it to more specialized class. It allows programmer to write better code.
Using inheritance you can use or inherit all the data fields and methods available in your base class. Later you can add you own methods and data fields, thus inheritance provides a way to organize code, rather than rewriting it from scratch.
In object-oriented terminology when class X extend class Y, then Y is called super/parent/base class and X is called subclass/child/derived class. One point to note here is that only data fields and method which are not private are accessible by child classes. Private data fields and methods are accessible only inside the class.
syntax to create a derived class is −
class BaseClass:
Body of base class
class DerivedClass(BaseClass):
Body of derived class
Inheriting Attributes
Now look at the below example −
Output
We first created a class called Date and pass the object as an argument, here-object is built-in class provided by Python. Later we created another class called time and called the Date class as an argument. Through this call we get access to all the data and attributes of Date class into the Time class. Because of that when we try to get the get_date method from the Time class object tm we created earlier possible.
Object.Attribute Lookup Hierarchy
- The instance
- The class
- Any class from which this class inherits
Inheritance Examples
Let’s take a closure look into the inheritance example −
Let’s create couple of classes to participate in examples −
- Animal − Class simulate an animal
- Cat − Subclass of Animal
- Dog − Subclass of Animal
In Python, constructor of class used to create an object (instance), and assign the value for the attributes.
Constructor of subclasses always called to a constructor of parent class to initialize value for the attributes in the parent class, then it start assign value for its attributes.
Output
In the above example, we see the command attributes or methods we put in the parent class so that all subclasses or child classes will inherits that property from the parent class.
If a subclass try to inherits methods or data from another subclass then it will through an error as we see when Dog class try to call swatstring() methods from that cat class, it throws an error(like AttributeError in our case).
Polymorphism (“MANY SHAPES”)
Polymorphism is an important feature of class definition in Python that is utilized when you have commonly named methods across classes or subclasses. This permits functions to use entities of different types at different times. So, it provides flexibility and loose coupling so that code can be extended and easily maintained over time.
This allows functions to use objects of any of these polymorphic classes without needing to be aware of distinctions across the classes.
Polymorphism can be carried out through inheritance, with subclasses making use of base class methods or overriding them.
Let understand the concept of polymorphism with our previous inheritance example and add one common method called show_affection in both subclasses −
From the example we can see, it refers to a design in which object of dissimilar type can be treated in the same manner or more specifically two or more classes with method of the same name or common interface because same method(show_affection in below example) is called with either type of objects.
Output
So, all animals show affections (show_affection), but they do differently. The “show_affection” behaviors is thus polymorphic in the sense that it acted differently depending on the animal. So, the abstract “animal” concept does not actually “show_affection”, but specific animals(like dogs and cats) have a concrete implementation of the action “show_affection”.
Python itself have classes that are polymorphic. Example, the len() function can be used with multiple objects and all return the correct output based on the input parameter.
Overriding
In Python, when a subclass contains a method that overrides a method of the superclass, you can also call the superclass method by calling
Super(Subclass, self).method instead of self.method.
Example
class Thought(object):
def __init__(self):
pass
def message(self):
print("Thought, always come and go")
class Advice(Thought):
def __init__(self):
super(Advice, self).__init__()
def message(self):
print('Warning: Risk is always involved when you are dealing with market!')
Inheriting the Constructor
If we see from our previous inheritance example, __init__ was located in the parent class in the up ‘cause the child class dog or cat didn’t‘ve __init__ method in it. Python used the inheritance attribute lookup to find __init__ in animal class. When we created the child class, first it will look the __init__ method in the dog class, then it didn’t find it then looked into parent class Animal and found there and called that there. So as our class design became complex we may wish to initialize a instance firstly processing it through parent class constructor and then through child class constructor.
Output
In above example- all animals have a name and all dogs a particular breed. We called parent class constructor with super. So dog has its own __init__ but the first thing that happen is we call super. Super is built in function and it is designed to relate a class to its super class or its parent class.
In this case we saying that get the super class of dog and pass the dog instance to whatever method we say here the constructor __init__. So in another words we are calling parent class Animal __init__ with the dog object. You may ask why we won’t just say Animal __init__ with the dog instance, we could do this but if the name of animal class were to change, sometime in the future. What if we wanna rearrange the class hierarchy,so the dog inherited from another class. Using super in this case allows us to keep things modular and easy to change and maintain.
So in this example we are able to combine general __init__ functionality with more specific functionality. This gives us opportunity to separate common functionality from the specific functionality which can eliminate code duplication and relate class to one another in a way that reflects the system overall design.
Conclusion
__init__ is like any other method; it can be inherited
If a class does not have a __init__ constructor, Python will check its parent class to see if it can find one.
As soon as it finds one, Python calls it and stops looking
We can use the super () function to call methods in the parent class.
We may want to initialize in the parent as well as our own class.
Multiple Inheritance and the Lookup Tree
As its name indicates, multiple inheritance is Python is when a class inherits from multiple classes.
For example, a child inherits personality traits from both parents (Mother and Father).
Python Multiple Inheritance Syntax
To make a class inherits from multiple parents classes, we write the the names of these classes inside the parentheses to the derived class while defining it. We separate these names with comma.
Below is an example of that −
>>> class Mother:
pass
>>> class Father:
pass
>>> class Child(Mother, Father):
pass
>>> issubclass(Child, Mother) and issubclass(Child, Father)
True
Multiple inheritance refers to the ability of inheriting from two or more than two class. The complexity arises as child inherits from parent and parents inherits from the grandparent class. Python climbs an inheriting tree looking for attributes that is being requested to be read from an object. It will check the in the instance, within class then parent class and lastly from the grandparent class. Now the question arises in what order the classes will be searched - breath-first or depth-first. By default, Python goes with the depth-first.
That’s is why in the below diagram the Python searches the dothis() method first in class A. So the method resolution order in the below example will be
Mro- D→B→A→C
Look at the below multiple inheritance diagram −
Let’s go through an example to understand the “mro” feature of an Python.
Output
Example 3
Let’s take another example of “diamond shape” multiple inheritance.
Above diagram will be considered ambiguous. From our previous example understanding “method resolution order” .i.e. mro will be D→B→A→C→A but it’s not. On getting the second A from the C, Python will ignore the previous A. so the mro will be in this case will be D→B→C→A.
Let’s create an example based on above diagram −
Output
Simple rule to understand the above output is- if the same class appear in the method resolution order, the earlier appearances of this class will be remove from the method resolution order.
In conclusion −
Any class can inherit from multiple classes
Python normally uses a “depth-first” order when searching inheriting classes.
But when two classes inherit from the same class, Python eliminates the first appearances of that class from the mro.
Decorators, Static and Class Methods
Functions(or methods) are created by def statement.
Though methods works in exactly the same way as a function except one point where method first argument is instance object.
We can classify methods based on how they behave, like
Simple method − defined outside of a class. This function can access class attributes by feeding instance argument:
def outside_func(():
Instance method −
def func(self,)
Class method − if we need to use class attributes
@classmethod
def cfunc(cls,)
Static method − do not have any info about the class
@staticmethod
def sfoo()
Till now we have seen the instance method, now is the time to get some insight into the other two methods,
Class Method
The @classmethod decorator, is a builtin function decorator that gets passed the class it was called on or the class of the instance it was called on as first argument. The result of that evaluation shadows your function definition.
syntax
class C(object):
@classmethod
def fun(cls, arg1, arg2, ...):
....
fun: function that needs to be converted into a class method
returns: a class method for function
They have the access to this cls argument, it can’t modify object instance state. That would require access to self.
It is bound to the class and not the object of the class.
Class methods can still modify class state that applies across all instances of the class.
Static Method
A static method takes neither a self nor a cls(class) parameter but it’s free to accept an arbitrary number of other parameters.
syntax
class C(object):
@staticmethod
def fun(arg1, arg2, ...):
...
returns: a static method for function funself.
- A static method can neither modify object state nor class state.
- They are restricted in what data they can access.
When to use what
We generally use class method to create factory methods. Factory methods return class object (similar to a constructor) for different use cases.
We generally use static methods to create utility functions.
Python Design Pattern
Overview
Modern software development needs to address complex business requirements. It also needs to take into account factors such as future extensibility and maintainability. A good design of a software system is vital to accomplish these goals. Design patterns play an important role in such systems.
To understand design pattern, let’s consider below example −
Every car’s design follows a basic design pattern, four wheels, steering wheel, the core drive system like accelerator-break-clutch, etc.
So, all things repeatedly built/ produced, shall inevitably follow a pattern in its design.. it cars, bicycle, pizza, atm machines, whatever…even your sofa bed.
Designs that have almost become standard way of coding some logic/mechanism/technique in software, hence come to be known as or studied as, Software Design Patterns.
Why is Design Pattern Important?
Benefits of using Design Patterns are −
Helps you to solve common design problems through a proven approach.
No ambiguity in the understanding as they are well documented.
Reduce the overall development time.
Helps you deal with future extensions and modifications with more ease than otherwise.
May reduce errors in the system since they are proven solutions to common problems.
Classification of Design Patterns
The GoF (Gang of Four) design patterns are classified into three categories namely creational, structural and behavioral.
Creational Patterns
Creational design patterns separate the object creation logic from the rest of the system. Instead of you creating objects, creational patterns creates them for you. The creational patterns include Abstract Factory, Builder, Factory Method, Prototype and Singleton.
Creational Patterns are not commonly used in Python because of the dynamic nature of the language. Also language itself provide us with all the flexibility we need to create in a sufficient elegant fashion, we rarely need to implement anything on top, like singleton or Factory.
Also these patterns provide a way to create objects while hiding the creation logic, rather than instantiating objects directly using a new operator.
Structural Patterns
Sometimes instead of starting from scratch, you need to build larger structures by using an existing set of classes. That’s where structural class patterns use inheritance to build a new structure. Structural object patterns use composition/ aggregation to obtain a new functionality. Adapter, Bridge, Composite, Decorator, Façade, Flyweight and Proxy are Structural Patterns. They offers best ways to organize class hierarchy.
Behavioral Patterns
Behavioral patterns offers best ways of handling communication between objects. Patterns comes under this categories are: Visitor, Chain of responsibility, Command, Interpreter, Iterator, Mediator, Memento, Observer, State, Strategy and Template method are Behavioral Patterns.
Because they represent the behavior of a system, they are used generally to describe the functionality of software systems.
Commonly used Design Patterns
Singleton
It is one of the most controversial and famous of all design patterns. It is used in overly object-oriented languages, and is a vital part of traditional object-oriented programming.
The Singleton pattern is used for,
When logging needs to be implemented. The logger instance is shared by all the components of the system.
The configuration files is using this because cache of information needs to be maintained and shared by all the various components in the system.
Managing a connection to a database.
Here is the UML diagram,
class Logger(object):
def __new__(cls, *args, **kwargs):
if not hasattr(cls, '_logger'):
cls._logger = super(Logger, cls).__new__(cls, *args, **kwargs)
return cls._logger
In this example, Logger is a Singleton.
When __new__ is called, it normally constructs a new instance of that class. When we override it, we first check if our singleton instance has been created or not. If not, we create it using a super call. Thus, whenever we call the constructor on Logger, we always get the exact same instance.
>>>
>>> obj1 = Logger()
>>> obj2 = Logger()
>>> obj1 == obj2
True
>>>
>>> obj1
<__main__.Logger object at 0x03224090>
>>> obj2
<__main__.Logger object at 0x03224090>
Object Oriented Python - Advanced Features
In this we will look into some of the advanced features which Python provide
Core Syntax in our Class design
In this we will look onto, how Python allows us to take advantage of operators in our classes. Python is largely objects and methods call on objects and this even goes on even when its hidden by some convenient syntax.
>>> var1 = 'Hello'
>>> var2 = ' World!'
>>> var1 + var2
'Hello World!'
>>>
>>> var1.__add__(var2)
'Hello World!'
>>> num1 = 45
>>> num2 = 60
>>> num1.__add__(num2)
105
>>> var3 = ['a', 'b']
>>> var4 = ['hello', ' John']
>>> var3.__add__(var4)
['a', 'b', 'hello', ' John']
So if we have to add magic method __add__ to our own classes, could we do that too. Let’s try to do that.
We have a class called Sumlist which has a contructor __init__ which takes list as an argument called my_list.
class SumList(object):
def __init__(self, my_list):
self.mylist = my_list
def __add__(self, other):
new_list = [ x + y for x, y in zip(self.mylist, other.mylist)]
return SumList(new_list)
def __repr__(self):
return str(self.mylist)
aa = SumList([3,6, 9, 12, 15])
bb = SumList([100, 200, 300, 400, 500])
cc = aa + bb # aa.__add__(bb)
print(cc) # should gives us a list ([103, 206, 309, 412, 515])
Output
[103, 206, 309, 412, 515]
But there are many methods which are internally managed by others magic methods. Below are some of them,
'abc' in var # var.__contains__('abc')
var == 'abc' # var.__eq__('abc')
var[1] # var.__getitem__(1)
var[1:3] # var.__getslice__(1, 3)
len(var) # var.__len__()
print(var) # var.__repr__()
Inheriting From built-in types
Classes can also inherit from built-in types this means inherits from any built-in and take advantage of all the functionality found there.
In below example we are inheriting from dictionary but then we are implementing one of its method __setitem__. This (setitem) is invoked when we set key and value in the dictionary. As this is a magic method, this will be called implicitly.
class MyDict(dict):
def __setitem__(self, key, val):
print('setting a key and value!')
dict.__setitem__(self, key, val)
dd = MyDict()
dd['a'] = 10
dd['b'] = 20
for key in dd.keys():
print('{0} = {1}'.format(key, dd[key]))
Output
setting a key and value!
setting a key and value!
a = 10
b = 20
Let’s extend our previous example, below we have called two magic methods called __getitem__ and __setitem__ better invoked when we deal with list index.
# Mylist inherits from 'list' object but indexes from 1 instead for 0!
class Mylist(list): # inherits from list
def __getitem__(self, index):
if index == 0:
raise IndexError
if index > 0:
index = index - 1
return list.__getitem__(self, index) # this method is called when
# we access a value with subscript like x[1]
def __setitem__(self, index, value):
if index == 0:
raise IndexError
if index > 0:
index = index - 1
list.__setitem__(self, index, value)
x = Mylist(['a', 'b', 'c']) # __init__() inherited from builtin list
print(x) # __repr__() inherited from builtin list
x.append('HELLO'); # append() inherited from builtin list
print(x[1]) # 'a' (Mylist.__getitem__ cutomizes list superclass
# method. index is 1, but reflects 0!
print (x[4]) # 'HELLO' (index is 4 but reflects 3!
Output
['a', 'b', 'c']
a
HELLO
In above example, we set a three item list in Mylist and implicitly __init__ method is called and when we print the element x, we get the three item list ([‘a’,’b’,’c’]). Then we append another element to this list. Later we ask for index 1 and index 4. But if you see the output, we are getting element from the (index-1) what we have asked for. As we know list indexing start from 0 but here the indexing start from 1 (that’s why we are getting the first item of the list).
Naming Conventions
In this we will look into names we’ll used for variables especially private variables and conventions used by Python programmers worldwide. Although variables are designated as private but there is not privacy in Python and this by design. Like any other well documented languages, Python has naming and style conventions that it promote although it doesn’t enforce them. There is a style guide written by “Guido van Rossum” the originator of Python, that describe the best practices and use of name and is called PEP8. Here is the link for this, https://www.python.org/dev/peps/pep-0008/
PEP stands for Python enhancement proposal and is a series of documentation that distributed among the Python community to discuss proposed changes. For example it is recommended all,
- Module names − all_lower_case
- Class names and exception names − CamelCase
- Global and local names − all_lower_case
- Functions and method names − all_lower_case
- Constants − ALL_UPPER_CASE
These are just the recommendation, you can vary if you like. But as most of the developers follows these recommendation so might me your code is less readable.
Why conform to convention?
We can follow the PEP recommendation we it allows us to get,
- More familiar to the vast majority of developers
- Clearer to most readers of your code.
- Will match style of other contributers who work on same code base.
- Mark of a professional software developers
- Everyone will accept you.
Variable Naming − ‘Public’ and ‘Private’
In Python, when we are dealing with modules and classes, we designate some variables or attribute as private. In Python, there is no existence of “Private” instance variable which cannot be accessed except inside an object. Private simply means they are simply not intended to be used by the users of the code instead they are intended to be used internally. In general, a convention is being followed by most Python developers i.e. a name prefixed with an underscore for example. _attrval (example below) should be treated as a non-public part of the API or any Python code, whether it is a function, a method or a data member. Below is the naming convention we follow,
Public attributes or variables (intended to be used by the importer of this module or user of this class) −regular_lower_case
Private attributes or variables (internal use by the module or class) −_single_leading_underscore
Private attributes that shouldn’t be subclassed −__double_leading_underscore
Magic attributes −__double_underscores__(use them, don’t create them)
class GetSet(object):
instance_count = 0 # public
__mangled_name = 'no privacy!' # special variable
def __init__(self, value):
self._attrval = value # _attrval is for internal use only
GetSet.instance_count += 1
@property
def var(self):
print('Getting the "var" attribute')
return self._attrval
@var.setter
def var(self, value):
print('setting the "var" attribute')
self._attrval = value
@var.deleter
def var(self):
print('deleting the "var" attribute')
self._attrval = None
cc = GetSet(5)
cc.var = 10 # public name
print(cc._attrval)
print(cc._GetSet__mangled_name)
Output
setting the "var" attribute
10
no privacy!
Object Oriented Python - Files and Strings
Strings
Strings are the most popular data types used in every programming language. Why? Because we, understand text better than numbers, so in writing and talking we use text and words, similarly in programming too we use strings. In string we parse text, analyse text semantics, and do data mining – and all this data is human consumed text.The string in Python is immutable.
String Manipulation
In Python, string can be marked in multiple ways, using single quote ( ‘ ), double quote( “ ) or even triple quote ( ‘’’ ) in case of multiline strings.
>>> # String Examples
>>> a = "hello"
>>> b = ''' A Multi line string,
Simple!'''
>>> e = ('Multiple' 'strings' 'togethers')
String manipulation is very useful and very widely used in every language. Often, programmers are required to break down strings and examine them closely.
Strings can be iterated over (character by character), sliced, or concatenated. The syntax is the same as for lists.
The str class has numerous methods on it to make manipulating strings easier. The dir and help commands provides guidance in the Python interpreter how to use them.
Below are some of the commonly used string methods we use.
Sr.No.
Method & Description
1
isalpha()
Checks if all characters are Alphabets
2
isdigit()
Checks Digit Characters
3
isdecimal()
Checks decimal Characters
4
isnumeric()
checks Numeric Characters
5
find()
Returns the Highest Index of substrings
6
istitle()
Checks for Titlecased strings
7
join()
Returns a concatenated string
8
lower()
returns lower cased string
9
upper()
returns upper cased string
10
partion()
Returns a tuple
11
bytearray()
Returns array of given byte size
12
enumerate()
Returns an enumerate object
13
isprintable()
Checks printable character
Let’s try to run couple of string methods,
>>> str1 = 'Hello World!'
>>> str1.startswith('h')
False
>>> str1.startswith('H')
True
>>> str1.endswith('d')
False
>>> str1.endswith('d!')
True
>>> str1.find('o')
4
>>> #Above returns the index of the first occurence of the character/substring.
>>> str1.find('lo')
3
>>> str1.upper()
'HELLO WORLD!'
>>> str1.lower()
'hello world!'
>>> str1.index('b')
Traceback (most recent call last):
File "<pyshell#19>", line 1, in <module>
str1.index('b')
ValueError: substring not found
>>> s = ('hello How Are You')
>>> s.split(' ')
['hello', 'How', 'Are', 'You']
>>> s1 = s.split(' ')
>>> '*'.join(s1)
'hello*How*Are*You'
>>> s.partition(' ')
('hello', ' ', 'How Are You')
>>>
String Formatting
In Python 3.x formatting of strings has changed, now it more logical and is more flexible. Formatting can be done using the format() method or the % sign(old style) in format string.
The string can contain literal text or replacement fields delimited by braces {} and each replacement field may contains either the numeric index of a positional argument or the name of a keyword argument.
syntax
str.format(*args, **kwargs)
Basic Formatting
>>> '{} {}'.format('Example', 'One')
'Example One'
>>> '{} {}'.format('pie', '3.1415926')
'pie 3.1415926'
Below example allows re-arrange the order of display without changing the arguments.
>>> '{1} {0}'.format('pie', '3.1415926')
'3.1415926 pie'
Padding and aligning strings
A value can be padded to a specific length.
>>> #Padding Character, can be space or special character
>>> '{:12}'.format('PYTHON')
'PYTHON '
>>> '{:>12}'.format('PYTHON')
' PYTHON'
>>> '{:<{}s}'.format('PYTHON',12)
'PYTHON '
>>> '{:*<12}'.format('PYTHON')
'PYTHON******'
>>> '{:*^12}'.format('PYTHON')
'***PYTHON***'
>>> '{:.15}'.format('PYTHON OBJECT ORIENTED PROGRAMMING')
'PYTHON OBJECT O'
>>> #Above, truncated 15 characters from the left side of a specified string
>>> '{:.{}}'.format('PYTHON OBJECT ORIENTED',15)
'PYTHON OBJECT O'
>>> #Named Placeholders
>>> data = {'Name':'Raghu', 'Place':'Bangalore'}
>>> '{Name} {Place}'.format(**data)
'Raghu Bangalore'
>>> #Datetime
>>> from datetime import datetime
>>> '{:%Y/%m/%d.%H:%M}'.format(datetime(2018,3,26,9,57))
'2018/03/26.09:57'
Strings are Unicode
Strings as collections of immutable Unicode characters. Unicode strings provide an opportunity to create software or programs that works everywhere because the Unicode strings can represent any possible character not just the ASCII characters.
Many IO operations only know how to deal with bytes, even if the bytes object refers to textual data. It is therefore very important to know how to interchange between bytes and Unicode.
Converting text to bytes
Converting a strings to byte object is termed as encoding. There are numerous forms of encoding, most common ones are: PNG; JPEG, MP3, WAV, ASCII, UTF-8 etc. Also this(encoding) is a format to represent audio, images, text, etc. in bytes.
This conversion is possible through encode(). It take encoding technique as argument. By default, we use ‘UTF-8’ technique.
>>> # Python Code to demonstrate string encoding
>>>
>>> # Initialising a String
>>> x = 'TutorialsPoint'
>>>
>>> #Initialising a byte object
>>> y = b'TutorialsPoint'
>>>
>>> # Using encode() to encode the String >>> # encoded version of x is stored in z using ASCII mapping
>>> z = x.encode('ASCII')
>>>
>>> # Check if x is converted to bytes or not
>>>
>>> if(z==y):
print('Encoding Successful!')
else:
print('Encoding Unsuccessful!')
Encoding Successful!
Converting bytes to text
Converting bytes to text is called the decoding. This is implemented through decode(). We can convert a byte string to a character string if we know which encoding is used to encode it.
So Encoding and decoding are inverse processes.
>>>
>>> # Python code to demonstrate Byte Decoding
>>>
>>> #Initialise a String
>>> x = 'TutorialsPoint'
>>>
>>> #Initialising a byte object
>>> y = b'TutorialsPoint'
>>>
>>> #using decode() to decode the Byte object
>>> # decoded version of y is stored in z using ASCII mapping
>>> z = y.decode('ASCII')
>>> #Check if y is converted to String or not
>>> if (z == x):
print('Decoding Successful!')
else:
print('Decoding Unsuccessful!') Decoding Successful!
>>>
File I/O
Operating systems represents files as a sequence of bytes, not text.
A file is a named location on disk to store related information. It is used to permanently store data in your disk.
In Python, a file operation takes place in the following order.
- Open a file
- Read or write onto a file (operation).Open a file
- Close the file.
Python wraps the incoming (or outgoing) stream of bytes with appropriate decode (or encode) calls so we can deal directly with str objects.
Opening a file
Python has a built-in function open() to open a file. This will generate a file object, also called a handle as it is used to read or modify the file accordingly.
>>> f = open(r'c:\users\rajesh\Desktop\index.webm','rb')
>>> f
<_io.BufferedReader name='c:\\users\\rajesh\\Desktop\\index.webm'>
>>> f.mode
'rb'
>>> f.name
'c:\\users\\rajesh\\Desktop\\index.webm'
For reading text from a file, we only need to pass the filename into the function. The file will be opened for reading, and the bytes will be converted to text using the platform default encoding.
Exception and Exception Classes
In general, an exception is any unusual condition. Exception usually indicates errors but sometimes they intentionally puts in the program, in cases like terminating a procedure early or recovering from a resource shortage. There are number of built-in exceptions, which indicate conditions like reading past the end of a file, or dividing by zero. We can define our own exceptions called custom exception.
Exception handling enables you handle errors gracefully and do something meaningful about it. Exception handling has two components: “throwing” and ‘catching’.
Identifying Exception (Errors)
Every error occurs in Python result an exception which will an error condition identified by its error type.
>>> #Exception
>>> 1/0
Traceback (most recent call last):
File "<pyshell#2>", line 1, in <module>
1/0
ZeroDivisionError: division by zero
>>>
>>> var = 20
>>> print(ver)
Traceback (most recent call last):
File "<pyshell#5>", line 1, in <module>
print(ver)
NameError: name 'ver' is not defined
>>> #Above as we have misspelled a variable name so we get an NameError.
>>>
>>> print('hello)
SyntaxError: EOL while scanning string literal
>>> #Above we have not closed the quote in a string, so we get SyntaxError.
>>>
>>> #Below we are asking for a key, that doen't exists.
>>> mydict = {}
>>> mydict['x']
Traceback (most recent call last):
File "<pyshell#15>", line 1, in <module>
mydict['x']
KeyError: 'x'
>>> #Above keyError
>>>
>>> #Below asking for a index that didn't exist in a list.
>>> mylist = [1,2,3,4]
>>> mylist[5]
Traceback (most recent call last):
File "<pyshell#20>", line 1, in <module>
mylist[5]
IndexError: list index out of range
>>> #Above, index out of range, raised IndexError.
Catching/Trapping Exception
When something unusual occurs in your program and you wish to handle it using the exception mechanism, you ‘throw an exception’. The keywords try and except are used to catch exceptions. Whenever an error occurs within a try block, Python looks for a matching except block to handle it. If there is one, execution jumps there.
syntax
try:
#write some code
#that might throw some exception
except <ExceptionType>:
# Exception handler, alert the user
The code within the try clause will be executed statement by statement.
If an exception occurs, the rest of the try block will be skipped and the except clause will be executed.
try:
some statement here
except:
exception handling
Let’s write some code to see what happens when you not use any error handling mechanism in your program.
number = int(input('Please enter the number between 1 & 10: '))
print('You have entered number',number)
Above programme will work correctly as long as the user enters a number, but what happens if the users try to puts some other data type(like a string or a list).
Please enter the number between 1 > 10: 'Hi'
Traceback (most recent call last):
File "C:/Python/Python361/exception2.py", line 1, in <module>
number = int(input('Please enter the number between 1 & 10: '))
ValueError: invalid literal for int() with base 10: "'Hi'"
Now ValueError is an exception type. Let’s try to rewrite the above code with exception handling.
import sys
print('Previous code with exception handling')
try:
number = int(input('Enter number between 1 > 10: '))
except(ValueError):
print('Error..numbers only')
sys.exit()
print('You have entered number: ',number)
If we run the program, and enter a string (instead of a number), we can see that we get a different result.
Previous code with exception handling
Enter number between 1 > 10: 'Hi'
Error..numbers only
Raising Exceptions
To raise your exceptions from your own methods you need to use raise keyword like this
raise ExceptionClass(‘Some Text Here’)
Let’s take an example
def enterAge(age):
if age<0:
raise ValueError('Only positive integers are allowed')
if age % 2 ==0:
print('Entered Age is even')
else:
print('Entered Age is odd')
try:
num = int(input('Enter your age: '))
enterAge(num)
except ValueError:
print('Only positive integers are allowed')
Run the program and enter positive integer.
Expected Output
Enter your age: 12
Entered Age is even
But when we try to enter a negative number we get,
Expected Output
Enter your age: -2
Only positive integers are allowed
Creating Custom exception class
You can create a custom exception class by Extending BaseException class or subclass of BaseException.
From above diagram we can see most of the exception classes in Python extends from the BaseException class. You can derive your own exception class from BaseException class or from its subclass.
Create a new file called NegativeNumberException.py and write the following code.
class NegativeNumberException(RuntimeError):
def __init__(self, age):
super().__init__()
self.age = age
Above code creates a new exception class named NegativeNumberException, which consists of only constructor which call parent class constructor using super()__init__() and sets the age.
Now to create your own custom exception class, will write some code and import the new exception class.
from NegativeNumberException import NegativeNumberException
def enterage(age):
if age < 0:
raise NegativeNumberException('Only positive integers are allowed')
if age % 2 == 0:
print('Age is Even')
else:
print('Age is Odd')
try:
num = int(input('Enter your age: '))
enterage(num)
except NegativeNumberException:
print('Only positive integers are allowed')
except:
print('Something is wrong')
Output
Enter your age: -2
Only positive integers are allowed
Another way to create a custom Exception class.
class customException(Exception):
def __init__(self, value):
self.parameter = value
def __str__(self):
return repr(self.parameter)
try:
raise customException('My Useful Error Message!')
except customException as instance:
print('Caught: ' + instance.parameter)
Output
Caught: My Useful Error Message!
Exception hierarchy
The class hierarchy for built-in exceptions is −
+-- SystemExit
+-- KeyboardInterrupt
+-- GeneratorExit
+-- Exception
+-- StopIteration
+-- StopAsyncIteration
+-- ArithmeticError
| +-- FloatingPointError
| +-- OverflowError
| +-- ZeroDivisionError
+-- AssertionError
+-- AttributeError
+-- BufferError
+-- EOFError
+-- ImportError
+-- LookupError
| +-- IndexError
| +-- KeyError
+-- MemoryError
+-- NameError
| +-- UnboundLocalError
+-- OSError
| +-- BlockingIOError
| +-- ChildProcessError
| +-- ConnectionError
| | +-- BrokenPipeError
| | +-- ConnectionAbortedError
| | +-- ConnectionRefusedError
| | +-- ConnectionResetError
| +-- FileExistsError
| +-- FileNotFoundError
| +-- InterruptedError
| +-- IsADirectoryError
| +-- NotADirectoryError
| +-- PermissionError
| +-- ProcessLookupError
| +-- TimeoutError
+-- ReferenceError
+-- RuntimeError
| +-- NotImplementedError
| +-- RecursionError
+-- SyntaxError
| +-- IndentationError
| +-- TabError
+-- SystemError
+-- TypeError
+-- ValueError
| +-- UnicodeError
| +-- UnicodeDecodeError
| +-- UnicodeEncodeError
| +-- UnicodeTranslateError
+-- Warning
+-- DeprecationWarning
+-- PendingDeprecationWarning
+-- RuntimeWarning
+-- SyntaxWarning
+-- UserWarning
+-- FutureWarning
+-- ImportWarning
+-- UnicodeWarning
+-- BytesWarning
+-- ResourceWarning
Object Oriented Python - Object Serialization
In the context of data storage, serialization is the process of translating data structures or object state into a format that can be stored (for example, in a file or memory buffer) or transmitted and reconstructed later.
In serialization, an object is transformed into a format that can be stored, so as to be able to deserialize it later and recreate the original object from the serialized format.
Pickle
Pickling is the process whereby a Python object hierarchy is converted into a byte stream (usually not human readable) to be written to a file, this is also known as Serialization. Unpickling is the reverse operation, whereby a byte stream is converted back into a working Python object hierarchy.
Pickle is operationally simplest way to store the object. The Python Pickle module is an object-oriented way to store objects directly in a special storage format.
What can it do?
- Pickle can store and reproduce dictionaries and lists very easily.
- Stores object attributes and restores them back to the same State.
What pickle can’t do?
- It does not save an objects code. Only it’s attributes values.
- It cannot store file handles or connection sockets.
In short we can say, pickling is a way to store and retrieve data variables into and out from files where variables can be lists, classes, etc.
To Pickle something you must −
- import pickle
- Write a variable to file, something like
pickle.dump(mystring, outfile, protocol),
where 3rd argument protocol is optional To unpickling something you must −
Import pickle
Write a variable to a file, something like
myString = pickle.load(inputfile)
Methods
The pickle interface provides four different methods.
dump() − The dump() method serializes to an open file (file-like object).
dumps() − Serializes to a string
load() − Deserializes from an open-like object.
loads() − Deserializes from a string.
Based on above procedure, below is an example of “pickling”.
Output
My Cat pussy is White and has 4 legs
Would you like to see her pickled? Here she is!
b'\x80\x03c__main__\nCat\nq\x00)\x81q\x01}q\x02(X\x0e\x00\x00\x00number_of_legsq\x03K\x04X\x05\x00\x00\x00colorq\x04X\x05\x00\x00\x00Whiteq\x05ub.'
So, in the example above, we have created an instance of a Cat class and then we’ve pickled it, transforming our “Cat” instance into a simple array of bytes.
This way we can easily store the bytes array on a binary file or in a database field and restore it back to its original form from our storage support in a later time.
Also if you want to create a file with a pickled object, you can use the dump() method ( instead of the dumps*()* one) passing also an opened binary file and the pickling result will be stored in the file automatically.
[….]
binary_file = open(my_pickled_Pussy.bin', mode='wb')
my_pickled_Pussy = pickle.dump(Pussy, binary_file)
binary_file.close()
Unpickling
The process that takes a binary array and converts it to an object hierarchy is called unpickling.
The unpickling process is done by using the load() function of the pickle module and returns a complete object hierarchy from a simple bytes array.
Let’s use the load function in our previous example.
Output
MeOw is black
Pussy is white
JSON
JSON(JavaScript Object Notation) has been part of the Python standard library is a lightweight data-interchange format. It is easy for humans to read and write. It is easy to parse and generate.
Because of its simplicity, JSON is a way by which we store and exchange data, which is accomplished through its JSON syntax, and is used in many web applications. As it is in human readable format, and this may be one of the reasons for using it in data transmission, in addition to its effectiveness when working with APIs.
An example of JSON-formatted data is as follow −
{"EmployID": 40203, "Name": "Zack", "Age":54, "isEmployed": True}
Python makes it simple to work with Json files. The module sused for this purpose is the JSON module. This module should be included (built-in) within your Python installation.
So let’s see how can we convert Python dictionary to JSON and write it to a text file.
JSON to Python
Reading JSON means converting JSON into a Python value (object). The json library parses JSON into a dictionary or list in Python. In order to do that, we use the loads() function (load from a string), as follow −
Output
Below is one sample json file,
data1.json
{"menu": {
"id": "file",
"value": "File",
"popup": {
"menuitem": [
{"value": "New", "onclick": "CreateNewDoc()"},
{"value": "Open", "onclick": "OpenDoc()"},
{"value": "Close", "onclick": "CloseDoc()"}
]
}
}}
Above content (Data1.json) looks like a conventional dictionary. We can use pickle to store this file but the output of it is not human readable form.
JSON(Java Script Object Notification) is a very simple format and that’s one of the reason for its popularity. Now let’s look into json output through below program.
Output
Above we open the json file (data1.json) for reading, obtain the file handler and pass on to json.load and getting back the object. When we try to print the output of the object, its same as the json file. Although the type of the object is dictionary, it comes out as a Python object. Writing to the json is simple as we saw this pickle. Above we load the json file, add another key value pair and writing it back to the same json file. Now if we see out data1.json, it looks different .i.e. not in the same format as we see previously.
To make our Output looks same (human readable format), add the couple of arguments into our last line of the program,
json.dump(conf, fh, indent = 4, separators = (‘,’, ‘: ‘))
Similarly like pickle, we can print the string with dumps and load with loads. Below is an example of that,
YAML
YAML may be the most human friendly data serialization standard for all programming languages.
Python yaml module is called pyaml
YAML is an alternative to JSON −
Human readable code − YAML is the most human readable format so much so that even its front-page content is displayed in YAML to make this point.
Compact code − In YAML we use whitespace indentation to denote structure not brackets.
Syntax for relational data − For internal references we use anchors (&) and aliases (*).
One of the area where it is used widely is for viewing/editing of data structures − for example configuration files, dumping during debugging and document headers.
Installing YAML
As yaml is not a built-in module, we need to install it manually. Best way to install yaml on windows machine is through pip. Run below command on your windows terminal to install yaml,
pip install pyaml (Windows machine)
sudo pip install pyaml (*nix and Mac)
On running above command, screen will display something like below based on what’s the current latest version.
Collecting pyaml
Using cached pyaml-17.12.1-py2.py3-none-any.whl
Collecting PyYAML (from pyaml)
Using cached PyYAML-3.12.tar.gz
Installing collected packages: PyYAML, pyaml
Running setup.py install for PyYAML ... done
Successfully installed PyYAML-3.12 pyaml-17.12.1
To test it, go to the Python shell and import the yaml module, import yaml, if no error is found, then we can say installation is successful.
After installing pyaml, let’s look at below code,
script_yaml1.py
Above we created three different data structure, dictionary, list and tuple. On each of the structure, we do yaml.dump. Important point is how the output is displayed on the screen.
Output
Dictionary output looks clean .ie. key: value.
White space to separate different objects.
List is notated with dash (-)
Tuple is indicated first with !!Python/tuple and then in the same format as lists.
Loading a yaml file
So let’s say I have one yaml file, which contains,
---
# An employee record
name: Raagvendra Joshi
job: Developer
skill: Oracle
employed: True
foods:
- Apple
- Orange
- Strawberry
- Mango
languages:
Oracle: Elite
power_builder: Elite
Full Stack Developer: Lame
education:
4 GCSEs
3 A-Levels
MCA in something called com
Now let’s write a code to load this yaml file through yaml.load function. Below is code for the same.
As the output doesn’t looks that much readable, I prettify it by using json in the end. Compare the output we got and the actual yaml file we have.
Output
One of the most important aspect of software development is debugging. In this section we’ll see different ways of Python debugging either with built-in debugger or third party debuggers.
PDB – The Python Debugger
The module PDB supports setting breakpoints. A breakpoint is an intentional pause of the program, where you can get more information about the programs state.
To set a breakpoint, insert the line
pdb.set_trace()
Example
pdb_example1.py
import pdb
x = 9
y = 7
pdb.set_trace()
total = x + y
pdb.set_trace()
We have inserted a few breakpoints in this program. The program will pause at each breakpoint (pdb.set_trace()). To view a variables contents simply type the variable name.
c:\Python\Python361>Python pdb_example1.py
> c:\Python\Python361\pdb_example1.py(8)<module>()
-> total = x + y
(Pdb) x
9
(Pdb) y
7
(Pdb) total
*** NameError: name 'total' is not defined
(Pdb)
Press c or continue to go on with the programs execution until the next breakpoint.
(Pdb) c
--Return--
> c:\Python\Python361\pdb_example1.py(8)<module>()->None
-> total = x + y
(Pdb) total
16
Eventually, you will need to debug much bigger programs – programs that use subroutines. And sometimes, the problem that you’re trying to find will lie inside a subroutine. Consider the following program.
import pdb
def squar(x, y):
out_squared = x^2 + y^2
return out_squared
if __name__ == "__main__":
#pdb.set_trace()
print (squar(4, 5))
Now on running the above program,
c:\Python\Python361>Python pdb_example2.py
> c:\Python\Python361\pdb_example2.py(10)<module>()
-> print (squar(4, 5))
(Pdb)
We can use ? to get help, but the arrow indicates the line that’s about to be executed. At this point it’s helpful to hit s to s to step into that line.
(Pdb) s
--Call--
>c:\Python\Python361\pdb_example2.py(3)squar()
-> def squar(x, y):
This is a call to a function. If you want an overview of where you are in your code, try l −
(Pdb) l
1 import pdb
2
3 def squar(x, y):
4 -> out_squared = x^2 + y^2
5
6 return out_squared
7
8 if __name__ == "__main__":
9 pdb.set_trace()
10 print (squar(4, 5))
[EOF]
(Pdb)
You can hit n to advance to the next line. At this point you are inside the out_squared method and you have access to the variable declared inside the function .i.e. x and y.
(Pdb) x
4
(Pdb) y
5
(Pdb) x^2
6
(Pdb) y^2
7
(Pdb) x**2
16
(Pdb) y**2
25
(Pdb)
So we can see the ^ operator is not what we wanted instead we need to use ** operator to do squares.
This way we can debug our program inside the functions/methods.
Logging
The logging module has been a part of Python’s Standard Library since Python version 2.3. As it’s a built-in module all Python module can participate in logging, so that our application log can include your own message integrated with messages from third party module. It provides a lot of flexibility and functionality.
Benefits of Logging
Diagnostic logging − It records events related to the application’s operation.
Audit logging − It records events for business analysis.
Messages are written and logged at levels of “severity” &minu
DEBUG (debug()) − diagnostic messages for development.
INFO (info()) − standard “progress” messages.
WARNING (warning()) − detected a non-serious issue.
ERROR (error()) − encountered an error, possibly serious.
CRITICAL (critical()) − usually a fatal error (program stops).
Let’s looks into below simple program,
import logging
logging.basicConfig(level=logging.INFO)
logging.debug('this message will be ignored') # This will not print
logging.info('This should be logged') # it'll print
logging.warning('And this, too') # It'll print
Above we are logging messages on severity level. First we import the module, call basicConfig and set the logging level. Level we set above is INFO. Then we have three different statement: debug statement, info statement and a warning statement.
Output of logging1.py
INFO:root:This should be logged
WARNING:root:And this, too
As the info statement is below debug statement, we are not able to see the debug message. To get the debug statement too in the Output terminal, all we need to change is the basicConfig level.
logging.basicConfig(level = logging.DEBUG)
And in the Output we can see,
DEBUG:root:this message will be ignored
INFO:root:This should be logged
WARNING:root:And this, too
Also the default behavior means if we don’t set any logging level is warning. Just comment out the second line from the above program and run the code.
#logging.basicConfig(level = logging.DEBUG)
Output
WARNING:root:And this, too
Python built in logging level are actually integers.
>>> import logging
>>>
>>> logging.DEBUG
10
>>> logging.CRITICAL
50
>>> logging.WARNING
30
>>> logging.INFO
20
>>> logging.ERROR
40
>>>
We can also save the log messages into the file.
logging.basicConfig(level = logging.DEBUG, filename = 'logging.log')
Now all log messages will go the file (logging.log) in your current working directory instead of the screen. This is a much better approach as it lets us to do post analysis of the messages we got.
We can also set the date stamp with our log message.
logging.basicConfig(level=logging.DEBUG, format = '%(asctime)s %(levelname)s:%(message)s')
Output will get something like,
2018-03-08 19:30:00,066 DEBUG:this message will be ignored
2018-03-08 19:30:00,176 INFO:This should be logged
2018-03-08 19:30:00,201 WARNING:And this, too
Benchmarking
Benchmarking or profiling is basically to test how fast is your code executes and where the bottlenecks are? The main reason to do this is for optimization.
timeit
Python comes with a in-built module called timeit. You can use it to time small code snippets. The timeit module uses platform-specific time functions so that you will get the most accurate timings possible.
So, it allows us to compare two shipment of code taken by each and then optimize the scripts to given better performance.
The timeit module has a command line interface, but it can also be imported.
There are two ways to call a script. Let’s use the script first, for that run the below code and see the Output.
import timeit
print ( 'by index: ', timeit.timeit(stmt = "mydict['c']", setup = "mydict = {'a':5, 'b':10, 'c':15}", number = 1000000))
print ( 'by get: ', timeit.timeit(stmt = 'mydict.get("c")', setup = 'mydict = {"a":5, "b":10, "c":15}', number = 1000000))
Output
by index: 0.1809192126703489
by get: 0.6088525265034692
Above we use two different method .i.e. by subscript and get to access the dictionary key value. We execute statement 1 million times as it executes too fast for a very small data. Now we can see the index access much faster as compared to the get. We can run the code multiply times and there will be slight variation in the time execution to get the better understanding.
Another way is to run the above test in the command line. Let’s do it,
c:\Python\Python361>Python -m timeit -n 1000000 -s "mydict = {'a': 5, 'b':10, 'c':15}" "mydict['c']"
1000000 loops, best of 3: 0.187 usec per loop
c:\Python\Python361>Python -m timeit -n 1000000 -s "mydict = {'a': 5, 'b':10, 'c':15}" "mydict.get('c')"
1000000 loops, best of 3: 0.659 usec per loop
Above output may vary based on your system hardware and what all applications are running currently in your system.
Below we can use the timeit module, if we want to call to a function. As we can add multiple statement inside the function to test.
import timeit
def testme(this_dict, key):
return this_dict[key]
print (timeit.timeit("testme(mydict, key)", setup = "from __main__ import testme; mydict = {'a':9, 'b':18, 'c':27}; key = 'c'", number = 1000000))
Output
0.7713474590139164
Object Oriented Python - Libraries
Requests − Python Requests Module
Requests is a Python module which is an elegant and simple HTTP library for Python. With this you can send all kinds of HTTP requests. With this library we can add headers, form data, multipart files and parameters and access the response data.
As Requests is not a built-in module, so we need to install it first.
You can install it by running the following command in the terminal −
pip install requests
Once you have installed the module, you can verify if the installation is successful by typing below command in the Python shell.
import requests
If the installation has been successful, you won’t see any error message.
Making a GET Request
As a means of example we’ll be using the “pokeapi”
Output −
Making POST Requests
The requests library methods for all of the HTTP verbs currently in use. If you wanted to make a simple POST request to an API endpoint then you can do that like so −
req = requests.post(‘http://api/user’, data = None, json = None)
This would work in exactly the same fashion as our previous GET request, however it features two additional keyword parameters −
data which can be populated with say a dictionary, a file or bytes that will be passed in the HTTP body of our POST request.
json which can be populated with a json object that will be passed in the body of our HTTP request also.
Pandas: Python Library Pandas
Pandas is an open-source Python Library providing high-performance data manipulation and analysis tool using its powerful data structures. Pandas is one of the most widely used Python libraries in data science. It is mainly used for data munging, and with good reason: Powerful and flexible group of functionality.
Built on Numpy package and the key data structure is called the DataFrame. These dataframes allows us to store and manipulate tabular data in rows of observations and columns of variables.
There are several ways to create a DataFrame. One way is to use a dictionary. For example −
Output
From the output we can see new brics DataFrame, Pandas has assigned a key for each country as the numerical values 0 through 4.
If instead of giving indexing values from 0 to 4, we would like to have different index values, say the two letter country code, you can do that easily as well −
Adding below one lines in the above code, gives
brics.index = ['BR', 'RU', 'IN', 'CH', 'SA']
Output
Indexing DataFrames
Output
Pygame
Pygame is the open source and cross-platform library that is for making multimedia applications including games. It includes computer graphics and sound libraries designed to be used with the Python programming language. You can develop many cool games with Pygame.’
Overview
Pygame is composed of various modules, each dealing with a specific set of tasks. For example, the display module deals with the display window and screen, the draw module provides functions to draw shapes and the key module works with the keyboard. These are just some of the modules of the library.
The home of the Pygame library is at https://www.pygame.org/news
To make a Pygame application, you follow these steps −
Import the Pygame library
import pygame
Initialize the Pygame library
pygame.init()
Create a window.
screen = Pygame.display.set_mode((560,480))
Pygame.display.set_caption(‘First Pygame Game’)
Initialize game objects
In this step we load images, load sounds, do object positioning, set up some state variables, etc.
Start the game loop.
It is just a loop where we continuously handle events, checks for input, move objects, and draw them. Each iteration of the loop is called a frame.
Let’s put all the above logic into one below program,
Pygame_script.py
Output
Beautiful Soup: Web Scraping with Beautiful Soup
The general idea behind web scraping is to get the data that exists on a website, and convert it into some format that is usable for analysis.
It’s a Python library for pulling data out of HTML or XML files. With your favourite parser it provide idiomatic ways of navigating, searching and modifying the parse tree.
As BeautifulSoup is not a built-in library, we need to install it before we try to use it. To install BeautifulSoup, run the below command
$ apt-get install Python-bs4 # For Linux and Python2
$ apt-get install Python3-bs4 # for Linux based system and Python3.
$ easy_install beautifulsoup4 # For windows machine,
Or
$ pip instal beatifulsoup4 # For window machine
Once the installation is done, we are ready to run few examples and explores Beautifulsoup in details,
Output
Below are some simple ways to navigate that data structure −
One common task is extracting all the URLs found within a page’s <a> tags −
Another common task is extracting all the text from a page −