객체 지향 파이썬-퀵 가이드
프로그래밍 언어는 지속적으로 등장하고 있으며 다른 방법론도 있습니다. 객체 지향 프로그래밍은 지난 몇 년 동안 매우 인기있는 방법론 중 하나입니다.
이 장에서는 파이썬 프로그래밍 언어를 객체 지향 프로그래밍 언어로 만드는 기능에 대해 설명합니다.
언어 프로그래밍 분류 체계
파이썬은 객체 지향 프로그래밍 방법론으로 특성화 될 수 있습니다. 다음 이미지는 다양한 프로그래밍 언어의 특성을 보여줍니다. 파이썬을 객체 지향으로 만드는 기능을 관찰하십시오.
언어 클래스 | 카테고리 | 언어 |
---|---|---|
프로그래밍 패러다임 | 절차 적 | C, C ++, C #, Objective-C, 자바, Go |
스크립팅 | CoffeeScript, JavaScript, Python, Perl, Php, Ruby | |
기능의 | 클로저,에 랄랑, 하스켈, 스칼라 | |
컴파일 클래스 | 공전 | C, C ++, C #, Objective-C, 자바, Go, Haskell, Scala |
동적 | CoffeeScript, JavaScript, Python, Perl, Php, Ruby, Clojure, Erlang | |
유형 클래스 | 강한 | C #, 자바, Go, Python, Ruby, Clojure, Erlang, Haskell, Scala |
약한 | C, C ++, C #, Objective-C, CoffeeScript, JavaScript, Perl, Php | |
메모리 클래스 | 관리 | 기타 |
관리되지 않음 | C, C ++, C #, Objective-C |
객체 지향 프로그래밍이란 무엇입니까?
Object Oriented사물을 향한 것을 의미합니다. 즉, 기능적으로 객체를 모델링하는 것을 의미합니다. 이것은 데이터와 동작을 통해 상호 작용하는 개체의 모음을 설명하여 복잡한 시스템을 모델링하는 데 사용되는 많은 기술 중 하나입니다.
객체 지향 프로그래밍 (OOP) 인 Python은 객체와 클래스를 사용하여 응용 프로그램을 설계하고 구축하는 데 초점을 맞춘 프로그래밍 방법입니다. OOP (객체 지향 프로그래밍)의 주요 기둥은 다음과 같습니다. Inheritance, Polymorphism, Abstraction, 기원 후 Encapsulation.
객체 지향 분석 (OOA)은 문제, 시스템 또는 작업을 검사하고 객체와 이들 간의 상호 작용을 식별하는 프로세스입니다.
객체 지향 프로그래밍을 선택하는 이유는 무엇입니까?
Python은 객체 지향 접근 방식으로 설계되었습니다. OOP는 다음과 같은 이점을 제공합니다.
명확한 프로그램 구조를 제공하여 실제 문제와 솔루션을 쉽게 매핑 할 수 있습니다.
기존 코드를 쉽게 유지 관리하고 수정할 수 있습니다.
각 개체가 독립적으로 존재하고 기존 개체를 방해하지 않고 새로운 기능을 쉽게 추가 할 수 있으므로 프로그램 모듈성이 향상됩니다.
제공된 구성 요소를 프로그래머가 쉽게 조정하고 수정할 수있는 코드 라이브러리를위한 좋은 프레임 워크를 제공합니다.
코드 재사용 성 부여
절차 적 프로그래밍과 객체 지향 프로그래밍
절차 기반 프로그래밍은 다음 개념을 기반으로하는 구조적 프로그래밍에서 파생됩니다. functions/procedure/routines. 절차 지향 프로그래밍에서 데이터에 쉽게 액세스하고 데이터를 변경할 수 있습니다. 반면에 OOP (Object Oriented Programming)는 문제를 다음과 같은 여러 단위로 분해 할 수 있습니다.objects그런 다음 이러한 개체를 중심으로 데이터와 함수를 구축합니다. 절차 나 기능보다 데이터에 더 중점을 둡니다. 또한 OOP에서 데이터는 숨겨져 있으며 외부 프로 시저에서 액세스 할 수 없습니다.
다음 이미지의 표는 POP 및 OOP 접근 방식의 주요 차이점을 보여줍니다.
절차 지향 프로그래밍 (POP) 대. 객체 지향 프로그래밍 (OOP).
절차 지향 프로그래밍 | 객체 지향 프로그래밍 | |
---|---|---|
기반 | Pop에서는 데이터와 기능에 중점을 둡니다. | Oops는 실제 시나리오를 기반으로하며 전체 프로그램은 개체라는 작은 부분으로 나뉩니다. |
재사용 성 | 제한된 코드 재사용 | 코드 재사용 |
접근하다 | 하향식 접근 | 개체 중심 디자인 |
액세스 지정자 | 아님 | 공개, 비공개 및 보호 |
데이터 이동 | 데이터는 기능간에 자유롭게 이동할 수 있습니다. | Oops에서 데이터는 멤버 함수를 통해 이동하고 서로 통신 할 수 있습니다. |
데이터 접근 | 팝에서 대부분의 기능은 공유를 위해 전역 데이터를 사용하여 기능에서 시스템의 기능으로 자유롭게 액세스 할 수 있습니다. | Oops에서 데이터는 메소드간에 자유롭게 이동할 수 없으며 공개 또는 비공개로 유지되어 데이터 액세스를 제어 할 수 있습니다. |
데이터 숨기기 | 팝에서는 데이터를 숨기는 특정 방법으로 보안 수준이 약간 떨어집니다. | 데이터 숨김을 제공하므로 훨씬 더 안전합니다. |
과부하 | 불가능 | 함수 및 연산자 오버로딩 |
예제-언어 | C, VB, 포트란, 파스칼 | C ++, Python, 자바, C # |
추출 | 프로 시저 수준에서 추상화 사용 | 클래스 및 객체 수준에서 추상화 사용 |
객체 지향 프로그래밍의 원리
객체 지향 프로그래밍 (OOP)은 다음과 같은 개념을 기반으로합니다. objects 행동보다는 data논리보다는. 프로그래밍 언어가 객체 지향이 되려면 클래스 및 객체 작업은 물론 상속, 추상화, 캡슐화 및 다형성과 같은 기본적인 객체 지향 원칙 및 개념의 구현 및 사용을 가능하게하는 메커니즘이 있어야합니다.

객체 지향 프로그래밍의 각 기둥을 간략하게 이해하겠습니다.
캡슐화
이 속성은 불필요한 세부 정보를 숨기고 프로그램 구조를보다 쉽게 관리 할 수 있도록합니다. 각 개체의 구현 및 상태는 잘 정의 된 경계 뒤에 숨겨져 있으며 작업을위한 깔끔하고 간단한 인터페이스를 제공합니다. 이를 수행하는 한 가지 방법은 데이터를 비공개로 만드는 것입니다.
계승
일반화라고도하는 상속을 통해 클래스와 객체 간의 계층 적 관계를 파악할 수 있습니다. 예를 들어, '과일'은 '주황색'의 일반화입니다. 상속은 코드 재사용 관점에서 매우 유용합니다.
추출
이 속성을 사용하면 세부 정보를 숨기고 개념 또는 개체의 필수 기능 만 노출 할 수 있습니다. 예를 들어 스쿠터를 운전하는 사람은 경적을 눌렀을 때 소리가 나는 것을 알고 있지만 경적을 눌렀을 때 실제로 소리가 어떻게 생성되는지 알지 못합니다.
다형성
다형성은 다양한 형태를 의미합니다. 즉, 사물이나 행동이 다른 형태 나 방식으로 존재합니다. 다형성의 한 가지 좋은 예는 클래스의 생성자 오버로딩입니다.
객체 지향 파이썬
Python 프로그래밍의 핵심은 object 과 OOP그러나 코드를 클래스로 구성하여 OOP를 사용하도록 제한 할 필요는 없습니다. OOP는 Python의 전체 디자인 철학에 추가되고 프로그래밍에 대한 깨끗하고 실용적인 방법을 장려합니다. OOP를 사용하면 더 크고 복잡한 프로그램을 작성할 수도 있습니다.
모듈 대 클래스 및 개체
모듈은 "사전"과 같습니다.
모듈에서 작업 할 때 다음 사항에 유의하십시오.
Python 모듈은 재사용 가능한 코드를 캡슐화하는 패키지입니다.
모듈은 __init__.py 그것에 파일.
모듈에는 함수와 클래스가 포함됩니다.
모듈은 import 예어.
사전은 key-value쌍. 즉, 키가있는 사전이있는 경우EmployeID 검색하고 싶다면 다음 코드 줄을 사용해야합니다.
employee = {“EmployeID”: “Employee Unique Identity!”}
print (employee [‘EmployeID])
다음과 같은 과정으로 모듈을 작업해야합니다.
모듈은 일부 함수 또는 변수가 포함 된 Python 파일입니다.
필요한 파일을 가져옵니다.
이제 '.'를 사용하여 해당 모듈의 함수 또는 변수에 액세스 할 수 있습니다. (dot) 운영자.
이름이 지정된 모듈을 고려하십시오. employee.py 그 안에 함수와 함께 employee. 함수의 코드는 다음과 같습니다.
# this goes in employee.py
def EmployeID():
print (“Employee Unique Identity!”)
이제 모듈을 가져온 다음 함수에 액세스하십시오. EmployeID −
import employee
employee. EmployeID()
이름이 지정된 변수를 삽입 할 수 있습니다. Age, 그림과 같이-
def EmployeID():
print (“Employee Unique Identity!”)
# just a variable
Age = “Employee age is **”
이제 다음과 같은 방법으로 해당 변수에 액세스하십시오.
import employee
employee.EmployeID()
print(employee.Age)
이제 이것을 사전과 비교해 봅시다.
Employee[‘EmployeID’] # get EmployeID from employee
Employee.employeID() # get employeID from the module
Employee.Age # get access to variable
Python에는 일반적인 패턴이 있습니다.
받아 key = value 스타일 컨테이너
열쇠 이름으로 뭔가 꺼내세요
모듈을 사전과 비교할 때 다음을 제외하고는 둘 다 비슷합니다.
의 경우 dictionary, 키는 문자열이고 구문은 [key]입니다.
의 경우 module, 키는 식별자이고 구문은 .key입니다.
클래스는 모듈과 같습니다.
Module은 Python 코드를 저장할 수있는 특수 사전이므로 '.' 운영자. 클래스는 함수와 데이터의 그룹화를 가져 와서 '.'연산자로 액세스 할 수 있도록 컨테이너 내부에 배치하는 방법입니다.
직원 모듈과 유사한 클래스를 만들어야하는 경우 다음 코드를 사용하여 수행 할 수 있습니다.
class employee(object):
def __init__(self):
self. Age = “Employee Age is ##”
def EmployeID(self):
print (“This is just employee unique identity”)
Note− 클래스는 그대로 재사용 할 수 있고 많은 간섭없이 모듈보다 선호됩니다. 모듈을 사용하는 동안 전체 프로그램에는 하나만 있습니다.
개체는 미니 가져 오기와 같습니다.
수업은 mini-module 클래스와 비슷한 방식으로 가져올 수 있습니다. instantiate. 클래스를 인스턴스화하면object.
다음과 같이 함수와 같은 클래스를 호출하는 것과 유사하게 객체를 인스턴스화 할 수 있습니다.
this_obj = employee() # Instantiatethis_obj.EmployeID() # get EmployeId from the class
print(this_obj.Age) # get variable Age
다음 세 가지 방법 중 하나로이 작업을 수행 할 수 있습니다.
# dictionary style
Employee[‘EmployeID’]
# module style
Employee.EmployeID()
Print(employee.Age)
# Class style
this_obj = employee()
this_obj.employeID()
Print(this_obj.Age)
이 장에서는 로컬 컴퓨터에서 Python 환경을 설정하는 방법에 대해 자세히 설명합니다.
전제 조건 및 툴킷
Python에 대해 자세히 알아보기 전에 다음 전제 조건이 충족되었는지 확인하는 것이 좋습니다.
최신 버전의 Python이 컴퓨터에 설치되어 있습니다.
IDE 또는 텍스트 편집기가 설치됨
Python으로 작성하고 디버깅하는 데 기본적인 지식이 있습니다. 즉, Python에서 다음을 수행 할 수 있습니다.
Python 프로그램을 작성하고 실행할 수 있습니다.
프로그램을 디버그하고 오류를 진단합니다.
기본 데이터 유형으로 작업합니다.
쓰다 for 루프, while 루프 및 if 명세서
암호 functions
프로그래밍 언어 경험이없는 경우 Python에서 초보자 자습서를 많이 찾을 수 있습니다.
https://www.tutorialpoints.com/Python 설치
다음 단계는 로컬 컴퓨터에 Python을 설치하는 방법을 자세히 보여줍니다.
Step 1 − 공식 Python 웹 사이트로 이동 https://www.python.org/, 클릭 Downloads 메뉴에서 최신 또는 안정적인 버전을 선택하십시오.

Step 2− 다운로드중인 Python 설치 프로그램 exe 파일을 저장하고 다운로드 한 후 엽니 다. 클릭Run 그리고 선택 Next 기본적으로 옵션을 선택하고 설치를 완료하십시오.

Step 3− 설치가 완료되면 아래 이미지와 같이 Python 메뉴가 표시됩니다. IDLE (Python GUI)를 선택하여 프로그램을 시작합니다.

그러면 Python 셸이 시작됩니다. 간단한 명령을 입력하여 설치를 확인하십시오.

IDE 선택
통합 개발 환경은 소프트웨어 개발을위한 텍스트 편집기입니다. 프로그래밍 흐름을 제어하고 Python에서 작업 할 때 프로젝트를 함께 그룹화하려면 IDE를 설치해야합니다. 다음은 온라인에서 사용할 수있는 몇 가지 IDE입니다. 편의에 따라 하나를 선택할 수 있습니다.
- Pycharm IDE
- 코모도 IDE
- Eric Python IDE
Note − Eclipse IDE는 대부분 Java에서 사용되지만 Python 플러그인이 있습니다.
파이 참

크로스 플랫폼 IDE 인 Pycharm은 현재 사용 가능한 가장 인기있는 IDE 중 하나입니다. 코드 완성, 프로젝트 및 코드 탐색, 통합 단위 테스트, 버전 제어 통합, 디버깅 등을 통해 코딩 지원 및 분석을 제공합니다.
다운로드 링크
https://www.jetbrains.com/pycharm/download/#section=windowsLanguages Supported − Python, HTML, CSS, JavaScript, Coffee Script, TypeScript, Cython, AngularJS, Node.js, 템플릿 언어.
스크린 샷

왜 선택해야합니까?
PyCharm은 사용자에게 다음과 같은 기능과 이점을 제공합니다.
- Windows, Linux 및 Mac OS와 호환되는 크로스 플랫폼 IDE
- Django IDE, CSS 및 JavaScript 지원 포함
- 수천 개의 플러그인, 통합 터미널 및 버전 제어 포함
- Git, SVN 및 Mercurial과 통합
- Python을위한 지능형 편집 도구 제공
- Virtualenv, Docker 및 Vagrant와의 손쉬운 통합
- 간단한 탐색 및 검색 기능
- 코드 분석 및 리팩토링
- 구성 가능한 주입
- 수많은 Python 라이브러리 지원
- 템플릿 및 JavaScript 디버거 포함
- Python / Django 디버거 포함
- Google App Engine, 추가 프레임 워크 및 라이브러리와 함께 작동합니다.
- 사용자 정의 가능한 UI, VIM 에뮬레이션 사용 가능
코모도 IDE

100 개 이상의 언어를 지원하고 기본적으로 Python, PHP 및 Ruby와 같은 동적 언어를 지원하는 다중 언어 IDE입니다. 모든 기능을 갖춘 21 일 무료 평가판으로 사용할 수있는 상용 IDE입니다. ActiveState는 Komodo IDE의 개발을 관리하는 소프트웨어 회사입니다. 또한 간단한 프로그래밍 작업을 위해 Komodo Edit로 알려진 잘린 Komodo 버전을 제공합니다.
이 IDE에는 가장 기본적인 수준부터 고급 수준까지 모든 종류의 기능이 포함되어 있습니다. 학생이나 프리랜서라면 실제 가격의 거의 절반을 살 수 있습니다. 그러나 공인 기관 및 대학의 교사와 교수에게는 완전히 무료입니다.
모든 언어 및 프레임 워크에 대한 지원을 포함하여 웹 및 모바일 개발에 필요한 모든 기능을 갖추고 있습니다.
다운로드 링크
Komodo Edit (무료 버전) 및 Komodo IDE (유료 버전)의 다운로드 링크는 다음과 같습니다.
Komodo Edit (free)
https://www.activestate.com/komodo-editKomodo IDE (paid)
https://www.activestate.com/komodo-ide/downloads/ide스크린 샷

왜 선택해야합니까?
- Perl, PHP, Python, Ruby 등을 지원하는 강력한 IDE.
- 교차 플랫폼 IDE.
통합 디버거 지원, 자동 완성, DOM (Document Object Model) 뷰어, 코드 브라우저, 대화 형 셸, 중단 점 구성, 코드 프로파일 링, 통합 단위 테스트와 같은 기본 기능이 포함됩니다. 요컨대 생산성 향상 기능이 많은 전문 IDE입니다.
Eric Python IDE

Python 및 Ruby 용 오픈 소스 IDE입니다. Eric은 Python으로 작성된 완전한 기능의 편집기이자 IDE입니다. 매우 유연한 Scintilla 편집기 컨트롤을 통합하는 크로스 플랫폼 Qt GUI 툴킷을 기반으로합니다. IDE는 구성이 매우 가능하며 무엇을 사용할지 선택하지 않을지 선택할 수 있습니다. 아래 링크에서 Eric IDE를 다운로드 할 수 있습니다.
https://eric-ide.python-projects.org/eric-download.html선택해야하는 이유
- 큰 들여 쓰기, 오류 강조 표시.
- 코드 지원
- 코드 완성
- PyLint로 코드 정리
- 빠른 탐색
- 통합 Python 디버거.
스크린 샷

텍스트 편집기 선택
항상 IDE가 필요한 것은 아닙니다. Python 또는 Arduino로 코딩하는 방법을 배우거나 일부 작업을 자동화하는 데 도움이되는 셸 스크립트의 빠른 스크립트 작업과 같은 작업의 경우 간단하고 가벼운 코드 중심 텍스트 편집기가 수행합니다. 또한 많은 텍스트 편집기는 IDE와 유사한 구문 강조 및 프로그램 내 스크립트 실행과 같은 기능을 제공합니다. 일부 텍스트 편집기가 여기에 제공됩니다.
- Atom
- 숭고한 텍스트
- Notepad++
Atom 텍스트 편집기

Atom은 GitHub 팀이 만든 해킹 가능한 텍스트 편집기입니다. 무료 오픈 소스 텍스트 및 코드 편집기로, 모든 코드를 읽고 자신의 용도로 수정하고 개선에 기여할 수 있습니다. Node.js 및 임베디드 Git Control로 작성된 플러그인을 지원하는 macOS, Linux 및 Microsoft Windows와 호환되는 크로스 플랫폼 텍스트 편집기입니다.
다운로드 링크
https://atom.io/스크린 샷
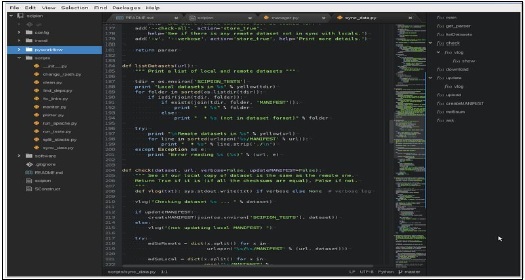
지원되는 언어
C / C ++, C #, CSS, CoffeeScript, HTML, JavaScript, Java, JSON, Julia, Objective-C, PHP, Perl, Python, Ruby on Rails, Ruby, Shell 스크립트, Scala, SQL, XML, YAML 등.
숭고한 텍스트 편집기

Sublime text는 독점 소프트웨어이며 구매하기 전에 테스트 할 수있는 무료 평가판을 제공합니다. stackoverflow.com 에 따르면 네 번째로 인기있는 개발 환경입니다.
그것이 제공하는 장점 중 일부는 놀라운 속도, 사용 용이성 및 커뮤니티 지원입니다. 또한 많은 프로그래밍 언어와 마크 업 언어를 지원하며, 일반적으로 커뮤니티에서 구축하고 무료 소프트웨어 라이선스에 따라 유지 관리하는 플러그인을 사용하여 사용자가 기능을 추가 할 수 있습니다.
스크린 샷

지원되는 언어
- Python, Ruby, JavaScript 등
왜 선택해야합니까?
키 바인딩, 메뉴, 스 니펫, 매크로, 완성 등을 사용자 지정합니다.
자동 완성 기능
- 스 니펫, 필드 마커 및 자리 표시자를 사용하여 숭고한 텍스트 스 니펫으로 텍스트 및 코드를 빠르게 삽입
빨리 열립니다
Mac, Linux 및 Windows에 대한 교차 플랫폼 지원.
가고 싶은 곳으로 커서를 이동
여러 줄, 단어 및 열 선택
메모장 ++

Python을 포함하여 Assembly에서 XML까지 여러 언어를 지원하는 무료 소스 코드 편집기 및 메모장 대체품입니다. MS Windows 환경에서 실행되는 경우 GPL 라이센스가 사용됩니다. 구문 강조 표시 외에도 Notepad ++에는 코더에게 특히 유용한 몇 가지 기능이 있습니다.
스크린 샷

주요 특징들
- 구문 강조 및 구문 접기
- PCRE (Perl 호환 정규식) 검색 / 바꾸기
- 완전히 사용자 정의 가능한 GUI
- SAuto 완성
- 탭 편집
- Multi-View
- 다국어 환경
- 다른 인수로 실행 가능
지원되는 언어
- Python, C, C ++, C #, Java 등과 같은 거의 모든 언어 (60 개 이상의 언어)
Python 데이터 구조는 구문 관점에서 매우 직관적이며 다양한 작업을 제공합니다. 데이터에 포함 된 내용, 수정해야하는 경우 또는 고정 데이터인지 여부 및 시작 / 종료 / 무작위 등에서 필요한 액세스 유형에 따라 Python 데이터 구조를 선택해야합니다.
기울기
List는 Python에서 가장 다양한 유형의 데이터 구조를 나타냅니다. 목록은 대괄호 사이에 쉼표로 구분 된 값 (항목 또는 요소)을 보유하는 컨테이너입니다. 목록은 여러 관련 값으로 작업 할 때 유용합니다. 목록은 데이터를 함께 보관하므로 한 번에 여러 값에 대해 동일한 메서드와 작업을 수행 할 수 있습니다. 목록 인덱스는 0부터 시작하고 문자열과 달리 목록은 변경 가능합니다.
데이터 구조-목록
>>>
>>> # Any Empty List
>>> empty_list = []
>>>
>>> # A list of String
>>> str_list = ['Life', 'Is', 'Beautiful']
>>> # A list of Integers
>>> int_list = [1, 4, 5, 9, 18]
>>>
>>> #Mixed items list
>>> mixed_list = ['This', 9, 'is', 18, 45.9, 'a', 54, 'mixed', 99, 'list']
>>> # To print the list
>>>
>>> print(empty_list)
[]
>>> print(str_list)
['Life', 'Is', 'Beautiful']
>>> print(type(str_list))
<class 'list'>
>>> print(int_list)
[1, 4, 5, 9, 18]
>>> print(mixed_list)
['This', 9, 'is', 18, 45.9, 'a', 54, 'mixed', 99, 'list']
Python 목록의 항목에 액세스
목록의 각 항목에는 번호가 할당됩니다. 즉 해당 번호의 인덱스 또는 위치입니다. 인덱싱은 항상 0에서 시작하고 두 번째 인덱스는 1입니다. 목록의 항목에 액세스하려면 대괄호 안에 이러한 색인 번호를 사용할 수 있습니다. 예를 들어 다음 코드를 관찰하십시오-
>>> mixed_list = ['This', 9, 'is', 18, 45.9, 'a', 54, 'mixed', 99, 'list']
>>>
>>> # To access the First Item of the list
>>> mixed_list[0]
'This'
>>> # To access the 4th item
>>> mixed_list[3]
18
>>> # To access the last item of the list
>>> mixed_list[-1]
'list'
빈 개체
빈 객체는 가장 단순하고 가장 기본적인 Python 내장 유형입니다. 우리는 눈치 채지 않고 여러 번 사용했으며 우리가 만든 모든 클래스로 확장했습니다. 빈 클래스를 작성하는 주된 목적은 당분간 무언가를 차단하고 나중에 확장하고 동작을 추가하는 것입니다.
클래스에 비헤이비어를 추가한다는 것은 데이터 구조를 객체로 교체하고 이에 대한 모든 참조를 변경하는 것을 의미합니다. 따라서 데이터를 생성하기 전에 데이터가 변장 된 개체인지 여부를 확인하는 것이 중요합니다. 더 나은 이해를 위해 다음 코드를 관찰하십시오.
>>> #Empty objects
>>>
>>> obj = object()
>>> obj.x = 9
Traceback (most recent call last):
File "<pyshell#3>", line 1, in <module>
obj.x = 9
AttributeError: 'object' object has no attribute 'x'
따라서 위에서 직접 인스턴스화 된 객체에 속성을 설정할 수 없음을 알 수 있습니다. 파이썬이 객체가 임의의 속성을 가질 수 있도록 허용 할 때, 속성 이름과 그 값을 모두 저장하기 위해 각 객체가 가진 속성을 추적하기 위해 일정량의 시스템 메모리가 필요합니다. 속성이 저장되지 않은 경우에도 잠재적 인 새 속성에 대해 일정량의 메모리가 할당됩니다.
따라서 Python은 기본적으로 객체 및 기타 여러 내장 기능의 임의 속성을 비활성화합니다.
>>> # Empty Objects
>>>
>>> class EmpObject:
pass
>>> obj = EmpObject()
>>> obj.x = 'Hello, World!'
>>> obj.x
'Hello, World!'
따라서 속성을 함께 그룹화하려면 위 코드와 같이 빈 개체에 저장할 수 있습니다. 그러나이 방법이 항상 제안되는 것은 아닙니다. 클래스와 객체는 데이터와 동작을 모두 지정하려는 경우에만 사용해야합니다.
튜플
튜플은 목록과 유사하며 요소를 저장할 수 있습니다. 그러나 변경이 불가능하므로 객체를 추가, 제거 또는 교체 할 수 없습니다. 불변성으로 인해 튜플이 제공하는 주요 이점은 사전이나 객체에 해시 값이 필요한 다른 위치에서 키로 사용할 수 있다는 것입니다.
튜플은 동작이 아니라 데이터를 저장하는 데 사용됩니다. 튜플을 조작하기 위해 동작이 필요한 경우 해당 동작을 수행하는 함수 (또는 다른 객체의 메서드)에 튜플을 전달해야합니다.
튜플은 사전 키 역할을 할 수 있으므로 저장된 값은 서로 다릅니다. 쉼표로 값을 구분하여 튜플을 만들 수 있습니다. 튜플은 괄호로 묶여 있지만 필수는 아닙니다. 다음 코드는 두 개의 동일한 할당을 보여줍니다.
>>> stock1 = 'MSFT', 95.00, 97.45, 92.45
>>> stock2 = ('MSFT', 95.00, 97.45, 92.45)
>>> type (stock1)
<class 'tuple'>
>>> type(stock2)
<class 'tuple'>
>>> stock1 == stock2
True
>>>
튜플 정의
튜플은 전체 요소 집합이 대괄호 대신 괄호로 묶여 있다는 점을 제외하면 목록과 매우 유사합니다.
목록을 분할 할 때와 마찬가지로 새 목록을 얻고 튜플을 분할하면 새 튜플을 얻습니다.
>>> tupl = ('Tuple','is', 'an','IMMUTABLE', 'list')
>>> tupl
('Tuple', 'is', 'an', 'IMMUTABLE', 'list')
>>> tupl[0]
'Tuple'
>>> tupl[-1]
'list'
>>> tupl[1:3]
('is', 'an')
Python 튜플 메서드
다음 코드는 Python 튜플의 메서드를 보여줍니다.
>>> tupl
('Tuple', 'is', 'an', 'IMMUTABLE', 'list')
>>> tupl.append('new')
Traceback (most recent call last):
File "<pyshell#148>", line 1, in <module>
tupl.append('new')
AttributeError: 'tuple' object has no attribute 'append'
>>> tupl.remove('is')
Traceback (most recent call last):
File "<pyshell#149>", line 1, in <module>
tupl.remove('is')
AttributeError: 'tuple' object has no attribute 'remove'
>>> tupl.index('list')
4
>>> tupl.index('new')
Traceback (most recent call last):
File "<pyshell#151>", line 1, in <module>
tupl.index('new')
ValueError: tuple.index(x): x not in tuple
>>> "is" in tupl
True
>>> tupl.count('is')
1
위에 표시된 코드에서 우리는 튜플이 불변이라는 것을 이해할 수 있습니다.
당신 cannot 튜플에 요소를 추가합니다.
당신 cannot 메서드를 추가하거나 확장합니다.
당신 cannot 튜플에서 요소를 제거합니다.
튜플은 no 제거 또는 팝 방법.
Count와 index는 튜플에서 사용할 수있는 메서드입니다.
사전
사전은 Python의 내장 데이터 유형 중 하나이며 키와 값 사이의 일대일 관계를 정의합니다.
사전 정의
사전 정의에 대해 이해하려면 다음 코드를 관찰하십시오.
>>> # empty dictionary
>>> my_dict = {}
>>>
>>> # dictionary with integer keys
>>> my_dict = { 1:'msft', 2: 'IT'}
>>>
>>> # dictionary with mixed keys
>>> my_dict = {'name': 'Aarav', 1: [ 2, 4, 10]}
>>>
>>> # using built-in function dict()
>>> my_dict = dict({1:'msft', 2:'IT'})
>>>
>>> # From sequence having each item as a pair
>>> my_dict = dict([(1,'msft'), (2,'IT')])
>>>
>>> # Accessing elements of a dictionary
>>> my_dict[1]
'msft'
>>> my_dict[2]
'IT'
>>> my_dict['IT']
Traceback (most recent call last):
File "<pyshell#177>", line 1, in <module>
my_dict['IT']
KeyError: 'IT'
>>>
위의 코드에서 우리는 다음을 관찰 할 수 있습니다.
먼저 두 개의 요소가있는 사전을 만들고 변수에 할당합니다. my_dict. 각 요소는 키-값 쌍이며 전체 요소 집합은 중괄호로 묶여 있습니다.
수 1 열쇠이고 msft그 가치입니다. 비슷하게,2 열쇠이고 IT 그 가치입니다.
키로 값을 얻을 수 있지만 그 반대는 불가능합니다. 따라서 우리가 시도 할 때my_dict[‘IT’] , 예외가 발생합니다. IT 열쇠가 아닙니다.
사전 수정
사전 수정에 대해 이해하려면 다음 코드를 관찰하십시오.
>>> # Modifying a Dictionary
>>>
>>> my_dict
{1: 'msft', 2: 'IT'}
>>> my_dict[2] = 'Software'
>>> my_dict
{1: 'msft', 2: 'Software'}
>>>
>>> my_dict[3] = 'Microsoft Technologies'
>>> my_dict
{1: 'msft', 2: 'Software', 3: 'Microsoft Technologies'}
위의 코드에서 우리는-
사전에 중복 키를 가질 수 없습니다. 기존 키의 값을 변경하면 이전 값이 삭제됩니다.
언제든지 새 키-값 쌍을 추가 할 수 있습니다.
사전에는 요소 간의 순서 개념이 없습니다. 순서가 지정되지 않은 단순한 컬렉션입니다.
사전에 데이터 유형 혼합
사전에서 혼합 데이터 유형에 대해 이해하려면 다음 코드를 관찰하십시오.
>>> # Mixing Data Types in a Dictionary
>>>
>>> my_dict
{1: 'msft', 2: 'Software', 3: 'Microsoft Technologies'}
>>> my_dict[4] = 'Operating System'
>>> my_dict
{1: 'msft', 2: 'Software', 3: 'Microsoft Technologies', 4: 'Operating System'}
>>> my_dict['Bill Gates'] = 'Owner'
>>> my_dict
{1: 'msft', 2: 'Software', 3: 'Microsoft Technologies', 4: 'Operating System',
'Bill Gates': 'Owner'}
위의 코드에서 우리는-
문자열뿐만 아니라 사전 값은 사전 자체를 포함하여 문자열, 정수를 포함한 모든 데이터 유형이 될 수 있습니다.
사전 값과 달리 사전 키는 더 제한적이지만 문자열, 정수 또는 기타 모든 유형이 될 수 있습니다.
사전에서 항목 삭제
사전에서 항목을 삭제하는 방법을 이해하려면 다음 코드를 참조하십시오.
>>> # Deleting Items from a Dictionary
>>>
>>> my_dict
{1: 'msft', 2: 'Software', 3: 'Microsoft Technologies', 4: 'Operating System',
'Bill Gates': 'Owner'}
>>>
>>> del my_dict['Bill Gates']
>>> my_dict
{1: 'msft', 2: 'Software', 3: 'Microsoft Technologies', 4: 'Operating System'}
>>>
>>> my_dict.clear()
>>> my_dict
{}
위의 코드에서 우리는-
del − 키로 사전에서 개별 항목을 삭제할 수 있습니다.
clear − 사전에서 모든 항목을 삭제합니다.
세트
Set ()는 중복 요소가없는 순서가 지정되지 않은 컬렉션입니다. 개별 항목은 변경 불가능하지만 세트 자체는 변경 가능합니다. 즉, 세트에서 요소 / 항목을 추가하거나 제거 할 수 있습니다. 집합을 사용하여 합집합, 교차 등의 수학적 연산을 수행 할 수 있습니다.
일반적으로 집합은 트리를 사용하여 구현할 수 있지만 Python으로 설정된 집합은 해시 테이블을 사용하여 구현할 수 있습니다. 이를 통해 특정 요소가 세트에 포함되어 있는지 확인하는 고도로 최적화 된 방법을 사용할 수 있습니다.
세트 만들기
모든 항목 (요소)을 중괄호 안에 배치하여 집합을 만듭니다. {}, 쉼표로 구분하거나 내장 함수를 사용하여 구분 set(). 다음 코드 줄을 관찰하십시오-
>>> #set of integers
>>> my_set = {1,2,4,8}
>>> print(my_set)
{8, 1, 2, 4}
>>>
>>> #set of mixed datatypes
>>> my_set = {1.0, "Hello World!", (2, 4, 6)}
>>> print(my_set)
{1.0, (2, 4, 6), 'Hello World!'}
>>>
세트 방법
집합에 대한 방법을 이해하려면 다음 코드를 관찰하십시오.
>>> >>> #METHODS FOR SETS
>>>
>>> #add(x) Method
>>> topics = {'Python', 'Java', 'C#'}
>>> topics.add('C++')
>>> topics
{'C#', 'C++', 'Java', 'Python'}
>>>
>>> #union(s) Method, returns a union of two set.
>>> topics
{'C#', 'C++', 'Java', 'Python'}
>>> team = {'Developer', 'Content Writer', 'Editor','Tester'}
>>> group = topics.union(team)
>>> group
{'Tester', 'C#', 'Python', 'Editor', 'Developer', 'C++', 'Java', 'Content
Writer'}
>>> # intersets(s) method, returns an intersection of two sets
>>> inters = topics.intersection(team)
>>> inters
set()
>>>
>>> # difference(s) Method, returns a set containing all the elements of
invoking set but not of the second set.
>>>
>>> safe = topics.difference(team)
>>> safe
{'Python', 'C++', 'Java', 'C#'}
>>>
>>> diff = topics.difference(group)
>>> diff
set()
>>> #clear() Method, Empties the whole set.
>>> group.clear()
>>> group
set()
>>>
집합 연산자
집합 연산자에 대해 이해하려면 다음 코드를 관찰하십시오.
>>> # PYTHON SET OPERATIONS
>>>
>>> #Creating two sets
>>> set1 = set()
>>> set2 = set()
>>>
>>> # Adding elements to set
>>> for i in range(1,5):
set1.add(i)
>>> for j in range(4,9):
set2.add(j)
>>> set1
{1, 2, 3, 4}
>>> set2
{4, 5, 6, 7, 8}
>>>
>>> #Union of set1 and set2
>>> set3 = set1 | set2 # same as set1.union(set2)
>>> print('Union of set1 & set2: set3 = ', set3)
Union of set1 & set2: set3 = {1, 2, 3, 4, 5, 6, 7, 8}
>>>
>>> #Intersection of set1 & set2
>>> set4 = set1 & set2 # same as set1.intersection(set2)
>>> print('Intersection of set1 and set2: set4 = ', set4)
Intersection of set1 and set2: set4 = {4}
>>>
>>> # Checking relation between set3 and set4
>>> if set3 > set4: # set3.issuperset(set4)
print('Set3 is superset of set4')
elif set3 < set4: #set3.issubset(set4)
print('Set3 is subset of set4')
else: #set3 == set4
print('Set 3 is same as set4')
Set3 is superset of set4
>>>
>>> # Difference between set3 and set4
>>> set5 = set3 - set4
>>> print('Elements in set3 and not in set4: set5 = ', set5)
Elements in set3 and not in set4: set5 = {1, 2, 3, 5, 6, 7, 8}
>>>
>>> # Check if set4 and set5 are disjoint sets
>>> if set4.isdisjoint(set5):
print('Set4 and set5 have nothing in common\n')
Set4 and set5 have nothing in common
>>> # Removing all the values of set5
>>> set5.clear()
>>> set5 set()
이 장에서는 객체 지향 용어와 프로그래밍 개념에 대해 자세히 설명합니다. 클래스는 인스턴스에 대한 단순한 팩토리입니다. 이 팩토리에는 인스턴스를 만드는 방법을 설명하는 청사진이 포함되어 있습니다. 인스턴스 또는 개체는 클래스에서 생성됩니다. 대부분의 경우 클래스의 인스턴스를 두 개 이상 가질 수 있습니다. 모든 인스턴스에는 속성 집합이 있으며 이러한 속성은 클래스에 정의되어 있으므로 특정 클래스의 모든 인스턴스는 동일한 속성을 가질 것으로 예상됩니다.
클래스 번들 : 동작 및 상태
클래스를 사용하면 개체의 동작과 상태를 함께 묶을 수 있습니다. 더 나은 이해를 위해 다음 다이어그램을 참조하십시오.
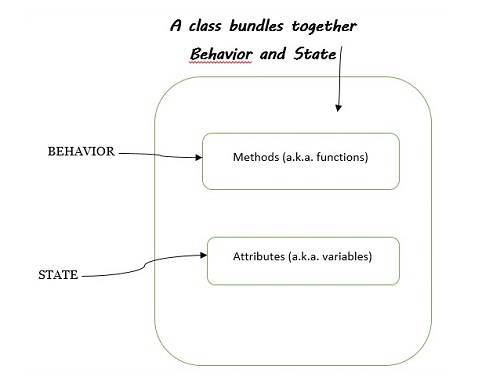
클래스 번들을 논의 할 때 다음 사항이 주목할 가치가 있습니다.
단어 behavior ~와 동일하다 function – 무언가를 수행하는 (또는 동작을 구현하는) 코드 조각입니다.
단어 state ~와 동일하다 variables – 클래스 내에서 값을 저장하는 장소입니다.
클래스 동작과 상태를 함께 주장 할 때, 이는 클래스가 함수와 변수를 패키지화한다는 것을 의미합니다.
클래스에는 메서드와 속성이 있습니다.
Python에서 메서드 생성은 클래스 동작을 정의합니다. method라는 단어는 클래스 내에 정의 된 함수에 부여 된 OOP 이름입니다. 요약하면-
Class functions −는 methods
Class variables −는 name attributes.
Class − 정확한 동작을 가진 인스턴스에 대한 청사진.
Object − 클래스의 인스턴스 중 하나는 클래스에 정의 된 기능을 수행합니다.
Type − 인스턴스가 속한 클래스를 나타냅니다.
Attribute − 모든 객체 값 : object.attribute
Method − 클래스에 정의 된 "호출 가능한 속성"
예를 들어 다음 코드를 관찰하십시오-
var = “Hello, John”
print( type (var)) # < type ‘str’> or <class 'str'>
print(var.upper()) # upper() method is called, HELLO, JOHN
생성 및 인스턴스화
다음 코드는 첫 번째 클래스와 인스턴스를 생성하는 방법을 보여줍니다.
class MyClass(object):
pass
# Create first instance of MyClass
this_obj = MyClass()
print(this_obj)
# Another instance of MyClass
that_obj = MyClass()
print (that_obj)
여기에서 우리는 MyClass어떤 작업도 수행하지 않습니다. 논쟁 거리object 에 MyClass class는 클래스 상속을 포함하며 이후 장에서 설명합니다. pass 위 코드에서이 블록이 비어 있음, 즉 빈 클래스 정의임을 나타냅니다.
인스턴스를 생성하겠습니다. this_obj 의 MyClass() 클래스와 함께 인쇄하십시오-
<__main__.MyClass object at 0x03B08E10>
<__main__.MyClass object at 0x0369D390>
여기에서 우리는 MyClass.16 진 코드는 객체가 저장되는 주소를 나타냅니다. 다른 인스턴스가 다른 주소를 가리키고 있습니다.
이제 클래스 내부에 하나의 변수를 정의하겠습니다. MyClass() 다음 코드와 같이 해당 클래스의 인스턴스에서 변수를 가져옵니다.
class MyClass(object):
var = 9
# Create first instance of MyClass
this_obj = MyClass()
print(this_obj.var)
# Another instance of MyClass
that_obj = MyClass()
print (that_obj.var)
산출
위에 주어진 코드를 실행하면 다음 출력을 관찰 할 수 있습니다.
9
9
인스턴스는 인스턴스화되는 클래스를 알고 있으므로 인스턴스에서 속성을 요청하면 인스턴스가 속성과 클래스를 찾습니다. 이것은attribute lookup.
인스턴스 방법
클래스에 정의 된 함수를 method.인스턴스 메서드를 호출하려면 인스턴스가 필요하며 데코레이터가 필요하지 않습니다. 인스턴스 메소드를 생성 할 때 첫 번째 매개 변수는 항상self. 다른 이름으로 (self)라고 부를 수는 있지만 명명 규칙이므로 self를 사용하는 것이 좋습니다.
class MyClass(object):
var = 9
def firstM(self):
print("hello, World")
obj = MyClass()
print(obj.var)
obj.firstM()
산출
위에 주어진 코드를 실행하면 다음 출력을 관찰 할 수 있습니다.
9
hello, World
위의 프로그램에서 우리는 self를 인자로하는 메소드를 정의했습니다. 그러나 인수를 선언하지 않았으므로 메서드를 호출 할 수 없습니다.
class MyClass(object):
def firstM(self):
print("hello, World")
print(self)
obj = MyClass()
obj.firstM()
print(obj)
산출
위에 주어진 코드를 실행하면 다음 출력을 관찰 할 수 있습니다.
hello, World
<__main__.MyClass object at 0x036A8E10>
<__main__.MyClass object at 0x036A8E10>
캡슐화
캡슐화는 OOP의 기본 요소 중 하나입니다. OOP를 사용하면 다음과 같은 방식으로 개발자에게 유리한 객체의 내부 작업의 복잡성을 숨길 수 있습니다.
내부를 몰라도 객체 사용을 단순화하고 이해하기 쉽게 만듭니다.
모든 변경 사항을 쉽게 관리 할 수 있습니다.
객체 지향 프로그래밍은 캡슐화에 크게 의존합니다. 캡슐화 및 추상화 (데이터 숨김이라고도 함)라는 용어는 종종 동의어로 사용됩니다. 캡슐화를 통해 추상화가 이루어 지므로 거의 동의어입니다.
캡슐화는 객체의 일부 구성 요소에 대한 액세스를 제한하는 메커니즘을 제공합니다. 이는 객체 정의 외부에서 객체의 내부 표현을 볼 수 없음을 의미합니다. 이 데이터에 대한 액세스는 일반적으로 특별한 방법을 통해 이루어집니다.Getters 과 Setters.
이 데이터는 인스턴스 속성에 저장되며 클래스 외부에서 조작 할 수 있습니다. 이를 보호하려면 해당 데이터는 인스턴스 메서드를 사용하여 액세스해야합니다. 직접 액세스는 허용되지 않아야합니다.
class MyClass(object):
def setAge(self, num):
self.age = num
def getAge(self):
return self.age
zack = MyClass()
zack.setAge(45)
print(zack.getAge())
zack.setAge("Fourty Five")
print(zack.getAge())
산출
위에 주어진 코드를 실행하면 다음 출력을 관찰 할 수 있습니다.
45
Fourty Five
데이터는 정확하고 유효한 경우에만 예외 처리 구문을 사용하여 저장해야합니다. 위에서 볼 수 있듯이 setAge () 메서드에 대한 사용자 입력에는 제한이 없습니다. 문자열, 숫자 또는 목록 일 수 있습니다. 따라서 우리는 저장의 정확성을 확인하기 위해 위의 코드를 확인해야합니다.
class MyClass(object):
def setAge(self, num):
self.age = num
def getAge(self):
return self.age
zack = MyClass()
zack.setAge(45)
print(zack.getAge())
zack.setAge("Fourty Five")
print(zack.getAge())
초기화 생성자
__init__ 메소드는 클래스의 객체가 인스턴스화 되 자마자 암시 적으로 호출되어 객체를 초기화합니다.
x = MyClass()
위에 표시된 코드 줄은 새 인스턴스를 만들고이 개체를 지역 변수 x에 할당합니다.
인스턴스화 작업, 즉 calling a class object, 빈 개체를 만듭니다. 많은 클래스는 특정 초기 상태에 맞게 사용자 정의 된 인스턴스로 객체를 생성하는 것을 좋아합니다. 따라서 클래스는 다음과 같이 '__init __ ()'라는 특수 메서드를 정의 할 수 있습니다.
def __init__(self):
self.data = []
Python은 인스턴스화 중에 __init__를 호출하여 클래스가 인스턴스화 될 때 발생해야하는 추가 속성을 정의합니다.이 속성은 해당 객체에 대한 일부 시작 값을 설정하거나 인스턴스화에 필요한 루틴을 실행할 수 있습니다. 따라서이 예제에서 초기화 된 새 인스턴스는 다음과 같이 얻을 수 있습니다.
x = MyClass()
__init __ () 메서드는 유연성을 높이기 위해 단일 또는 다중 인수를 가질 수 있습니다. init는 인스턴스의 속성을 초기화하므로 초기화를 의미합니다. 클래스의 생성자라고합니다.
class myclass(object):
def __init__(self,aaa, bbb):
self.a = aaa
self.b = bbb
x = myclass(4.5, 3)
print(x.a, x.b)
산출
4.5 3
클래스 속성
클래스에 정의 된 속성을 '클래스 속성'이라고하고 함수에 정의 된 속성을 '인스턴스 속성'이라고합니다. 정의하는 동안 이러한 속성은 특정 인스턴스가 아닌 클래스의 속성이기 때문에 self가 접두사로 붙지 않습니다.
클래스 속성은 클래스 자체 (className.attributeName) 및 클래스 인스턴스 (inst.attributeName)에서 액세스 할 수 있습니다. 따라서 인스턴스는 인스턴스 속성과 클래스 속성 모두에 액세스 할 수 있습니다.
>>> class myclass():
age = 21
>>> myclass.age
21
>>> x = myclass()
>>> x.age
21
>>>
캡슐화를 해제하는 좋은 방법이 아니지만 클래스 속성은 인스턴스에서 재정의 될 수 있습니다.
Python에는 속성에 대한 조회 경로가 있습니다. 첫 번째는 클래스 내에 정의 된 메서드이고 그 위에있는 클래스입니다.
>>> class myclass(object):
classy = 'class value'
>>> dd = myclass()
>>> print (dd.classy) # This should return the string 'class value'
class value
>>>
>>> dd.classy = "Instance Value"
>>> print(dd.classy) # Return the string "Instance Value"
Instance Value
>>>
>>> # This will delete the value set for 'dd.classy' in the instance.
>>> del dd.classy
>>> >>> # Since the overriding attribute was deleted, this will print 'class
value'.
>>> print(dd.classy)
class value
>>>
인스턴스 dd에서 'classy'클래스 속성을 재정의합니다. 재정의되면 Python 인터프리터는 재정의 된 값을 읽습니다. 그러나 새 값이 'del'로 삭제되면 재정의 된 값이 더 이상 인스턴스에 존재하지 않으므로 조회가 한 수준 위로 올라가 클래스에서 가져옵니다.
클래스 및 인스턴스 데이터 작업
이 섹션에서는 클래스 데이터가 인스턴스 데이터와 어떻게 관련되는지 이해하겠습니다. 우리는 클래스 나 인스턴스에 데이터를 저장할 수 있습니다. 클래스를 디자인 할 때 인스턴스에 속하는 데이터와 전체 클래스에 저장해야하는 데이터를 결정합니다.
인스턴스는 클래스 데이터에 액세스 할 수 있습니다. 여러 인스턴스를 생성하면 이러한 인스턴스는 개별 속성 값과 전체 클래스 데이터에 액세스 할 수 있습니다.
따라서 클래스 데이터는 모든 인스턴스간에 공유되는 데이터입니다. 더 나은 언더 샌딩을 위해 아래 주어진 코드를 관찰하십시오-
class InstanceCounter(object):
count = 0 # class attribute, will be accessible to all instances
def __init__(self, val):
self.val = val
InstanceCounter.count +=1 # Increment the value of class attribute, accessible through class name
# In above line, class ('InstanceCounter') act as an object
def set_val(self, newval):
self.val = newval
def get_val(self):
return self.val
def get_count(self):
return InstanceCounter.count
a = InstanceCounter(9)
b = InstanceCounter(18)
c = InstanceCounter(27)
for obj in (a, b, c):
print ('val of obj: %s' %(obj.get_val())) # Initialized value ( 9, 18, 27)
print ('count: %s' %(obj.get_count())) # always 3
산출
val of obj: 9
count: 3
val of obj: 18
count: 3
val of obj: 27
count: 3
간단히 말해, 클래스 속성은 클래스의 모든 인스턴스에 대해 동일하지만 인스턴스 속성은 각 인스턴스에 대해 특별합니다. 두 개의 다른 인스턴스에 대해 두 개의 다른 인스턴스 속성이 있습니다.
class myClass:
class_attribute = 99
def class_method(self):
self.instance_attribute = 'I am instance attribute'
print (myClass.__dict__)
산출
위에 주어진 코드를 실행하면 다음 출력을 관찰 할 수 있습니다.
{'__module__': '__main__', 'class_attribute': 99, 'class_method': <function myClass.class_method at 0x04128D68>, '__dict__': <attribute '__dict__' of 'myClass' objects>, '__weakref__': <attribute '__weakref__' of 'myClass' objects>, '__doc__': None}
인스턴스 속성 myClass.__dict__ 그림과 같이-
>>> a = myClass()
>>> a.class_method()
>>> print(a.__dict__)
{'instance_attribute': 'I am instance attribute'}
이 장에서는 Python의 다양한 내장 함수, 파일 I / O 작업 및 오버로딩 개념에 대해 자세히 설명합니다.
Python 내장 함수
Python 인터프리터에는 쉽게 사용할 수있는 내장 함수라고하는 여러 함수가 있습니다. 최신 버전에서 Python은 아래 표에 나열된 68 개의 내장 함수를 포함합니다.
내장 기능 | ||||
---|---|---|---|---|
abs () | dict () | 도움() | min () | setattr () |
모두() | dir () | 마녀() | 다음() | 일부분() |
어떤() | divmod () | 신분증() | 목적() | 정렬 됨 () |
ascii () | 세다() | 입력() | oct () | staticmethod () |
큰 상자() | eval () | int () | 열다() | str () |
부울 () | exec () | isinstance () | ord () | 합집합() |
bytearray () | 필터() | issubclass () | 펑 () | 감독자() |
바이트 () | 흙손() | iter () | 인쇄() | 튜플 () |
callable () | 체재() | len () | 특성() | 유형() |
chr () | frozenset () | 명부() | 범위() | vars () |
classmethod () | getattr () | 지역 주민 () | repr () | 지퍼() |
엮다() | 전역 () | 지도() | 반전 () | __수입__() |
복잡한() | hasattr () | max () | 일주() | |
delattr () | 해시시() | memoryview () | 세트() |
이 섹션에서는 몇 가지 중요한 기능에 대해 간략하게 설명합니다.
len () 함수
len () 함수는 문자열, 목록 또는 컬렉션의 길이를 가져옵니다. 개체의 항목 수 또는 길이를 반환하며 개체는 문자열, 목록 또는 컬렉션 일 수 있습니다.
>>> len(['hello', 9 , 45.0, 24])
4
len () 함수는 내부적으로 다음과 같이 작동합니다. list.__len__() 또는 tuple.__len__(). 따라서 len ()은 __가있는 객체에서만 작동합니다.len__() 방법.
>>> set1
{1, 2, 3, 4}
>>> set1.__len__()
4
그러나 실제로 우리는 len() 대신 __len__() 다음과 같은 이유로 인해 기능-
더 효율적입니다. 그리고 __len__과 같은 특수 메서드에 대한 액세스를 거부하기 위해 특정 메서드를 작성할 필요는 없습니다.
유지 관리가 쉽습니다.
이전 버전과의 호환성을 지원합니다.
반전 (seq)
역방향 반복자를 반환합니다. seq는 __reversed __ () 메서드가 있거나 시퀀스 프로토콜 (__len __ () 메서드 및 __getitem __ () 메서드)을 지원하는 객체 여야합니다. 일반적으로 사용됩니다for 항목을 뒤에서 앞으로 반복하고 싶을 때 반복합니다.
>>> normal_list = [2, 4, 5, 7, 9]
>>>
>>> class CustomSequence():
def __len__(self):
return 5
def __getitem__(self,index):
return "x{0}".format(index)
>>> class funkyback():
def __reversed__(self):
return 'backwards!'
>>> for seq in normal_list, CustomSequence(), funkyback():
print('\n{}: '.format(seq.__class__.__name__), end="")
for item in reversed(seq):
print(item, end=", ")
끝에있는 for 루프는 일반 목록의 반전 된 목록과 두 사용자 지정 시퀀스의 인스턴스를 인쇄합니다. 출력은reversed() 세 가지 모두에서 작동하지만 정의 할 때 결과는 매우 다릅니다. __reversed__.
산출
위에 주어진 코드를 실행하면 다음 출력을 관찰 할 수 있습니다.
list: 9, 7, 5, 4, 2,
CustomSequence: x4, x3, x2, x1, x0,
funkyback: b, a, c, k, w, a, r, d, s, !,
세다
그만큼 enumerate () 메서드는 iterable에 카운터를 추가하고 열거 개체를 반환합니다.
enumerate () 구문은 다음과 같습니다.
enumerate(iterable, start = 0)
여기 두 번째 주장 start 선택 사항이며 기본적으로 인덱스는 0으로 시작합니다.
>>> # Enumerate
>>> names = ['Rajesh', 'Rahul', 'Aarav', 'Sahil', 'Trevor']
>>> enumerate(names)
<enumerate object at 0x031D9F80>
>>> list(enumerate(names))
[(0, 'Rajesh'), (1, 'Rahul'), (2, 'Aarav'), (3, 'Sahil'), (4, 'Trevor')]
>>>
그래서 enumerate()전달 된 시퀀스의 요소 수를 유지하는 튜플을 생성하는 반복기를 반환합니다. 반환 값은 반복기이므로 직접 액세스하는 것은별로 유용하지 않습니다. enumerate ()에 대한 더 나은 접근 방식은 for 루프 내에서 카운트를 유지하는 것입니다.
>>> for i, n in enumerate(names):
print('Names number: ' + str(i))
print(n)
Names number: 0
Rajesh
Names number: 1
Rahul
Names number: 2
Aarav
Names number: 3
Sahil
Names number: 4
Trevor
표준 라이브러리에는 다른 많은 함수가 있으며 여기에 더 널리 사용되는 함수 목록이 있습니다.
hasattr, getattr, setattr 과 delattr, 객체의 속성을 문자열 이름으로 조작 할 수 있습니다.
all 과 any, 반복 가능한 객체를 받아들이고 True 항목의 전부 또는 일부가 참으로 평가되는 경우.
nzip, 두 개 이상의 시퀀스를 취하고 새로운 튜플 시퀀스를 반환합니다. 여기서 각 튜플은 각 시퀀스의 단일 값을 포함합니다.
파일 I / O
파일의 개념은 객체 지향 프로그래밍이라는 용어와 관련이 있습니다. 파이썬은 운영 체제가 파일 객체로 작업 할 수 있도록 추상화 된 인터페이스를 제공했습니다.
그만큼 open()내장 함수는 파일을 열고 파일 객체를 반환하는 데 사용됩니다. 두 개의 인수가있는 가장 일반적으로 사용되는 함수입니다.
open(filename, mode)
open () 함수는 두 개의 인수를 호출합니다. 첫 번째는 파일 이름이고 두 번째는 모드입니다. 여기서 모드는 읽기 전용 모드 인 경우 'r', 쓰기 전용 인 경우 'w'(동일한 이름의 기존 파일이 지워짐)가 될 수 있으며, 'a'는 추가를 위해 파일을 열고 파일에 기록 된 모든 데이터가 자동으로 추가됩니다. 끝까지. 'r +'는 읽기와 쓰기를 위해 파일을 엽니 다. 기본 모드는 읽기 전용입니다.
Windows에서 모드에 'b'가 추가되면 바이너리 모드로 파일이 열리므로 'rb', 'wb'및 'r + b'와 같은 모드도 있습니다.
>>> text = 'This is the first line'
>>> file = open('datawork','w')
>>> file.write(text)
22
>>> file.close()
어떤 경우에는 덮어 쓰는 대신 기존 파일에 추가하기 만하면됩니다. 기존 파일을 완전히 덮어 쓰는 대신 모드 인수로 'a'값을 제공하여 파일 끝에 추가 할 수 있기 때문입니다. 내용.
>>> f = open('datawork','a')
>>> text1 = ' This is second line'
>>> f.write(text1)
20
>>> f.close()
읽기 위해 파일이 열리면 read, readline 또는 readlines 메소드를 호출하여 파일 내용을 가져올 수 있습니다. read 메서드는 두 번째 인수가 'b'인지 여부에 따라 파일의 전체 내용을 str 또는 bytes 객체로 반환합니다.
가독성을 위해 그리고 한 번에 큰 파일을 읽지 않으려면 파일 객체에서 직접 for 루프를 사용하는 것이 좋습니다. 텍스트 파일의 경우 각 줄을 한 번에 하나씩 읽고 루프 본문 내에서 처리 할 수 있습니다. 그러나 바이너리 파일의 경우 read () 메서드를 사용하여 고정 크기의 데이터 청크를 읽고 읽을 최대 바이트 수에 대한 매개 변수를 전달하는 것이 좋습니다.
>>> f = open('fileone','r+')
>>> f.readline()
'This is the first line. \n'
>>> f.readline()
'This is the second line. \n'
파일 개체에 대한 쓰기 메서드를 통해 파일에 쓰면 문자열 (이진 데이터의 경우 바이트) 개체가 파일에 씁니다. writelines 메서드는 문자열 시퀀스를 받아들이고 각 반복 값을 파일에 씁니다. writelines 메서드는 시퀀스의 각 항목 뒤에 새 줄을 추가하지 않습니다.
마지막으로 파일 읽기 또는 쓰기를 마쳤을 때 close () 메서드를 호출하여 버퍼링 된 쓰기가 디스크에 기록되었는지, 파일이 제대로 정리되었는지, 파일과 연결된 모든 리소스가 다시 해제되었는지 확인해야합니다. 운영 체제. close () 메서드를 호출하는 것이 더 나은 방법이지만 기술적으로는 스크립트가 존재할 때 자동으로 발생합니다.
메서드 오버로딩의 대안
메서드 오버로딩은 서로 다른 인수 집합을 허용하는 동일한 이름의 여러 메서드를 갖는 것을 말합니다.
단일 메소드 또는 함수가 주어지면 매개 변수의 수를 직접 지정할 수 있습니다. 함수 정의에 따라 0, 1, 2 개 이상의 매개 변수를 사용하여 호출 할 수 있습니다.
class Human:
def sayHello(self, name = None):
if name is not None:
print('Hello ' + name)
else:
print('Hello ')
#Create Instance
obj = Human()
#Call the method, else part will be executed
obj.sayHello()
#Call the method with a parameter, if part will be executed
obj.sayHello('Rahul')
산출
Hello
Hello Rahul
기본 인수
함수는 객체이기도하다
호출 가능한 객체는 객체가 일부 인수를받을 수 있으며 가능하면 객체를 반환합니다. 함수는 Python에서 가장 간단한 호출 가능한 객체이지만 클래스 또는 특정 클래스 인스턴스와 같은 다른 객체도 있습니다.
Python의 모든 함수는 객체입니다. 객체는 메소드 나 함수를 포함 할 수 있지만 객체는 함수가 필요하지 않습니다.
def my_func():
print('My function was called')
my_func.description = 'A silly function'
def second_func():
print('Second function was called')
second_func.description = 'One more sillier function'
def another_func(func):
print("The description:", end=" ")
print(func.description)
print('The name: ', end=' ')
print(func.__name__)
print('The class:', end=' ')
print(func.__class__)
print("Now I'll call the function passed in")
func()
another_func(my_func)
another_func(second_func)
위의 코드에서 우리는 세 번째 함수에 인수로 두 개의 다른 함수를 전달하고 각각에 대해 다른 출력을 얻을 수 있습니다.
The description: A silly function
The name: my_func
The class:
Now I'll call the function passed in My function was called The description: One more sillier function The name: second_func The class:
Now I'll call the function passed in Second function was called
callable objects
Just as functions are objects that can have attributes set on them, it is possible to create an object that can be called as though it were a function.
In Python any object with a __call__() method can be called using function-call syntax.
Inheritance and Polymorphism
Inheritance and polymorphism – this is a very important concept in Python. You must understand it better if you want to learn.
Inheritance
One of the major advantages of Object Oriented Programming is re-use. Inheritance is one of the mechanisms to achieve the same. Inheritance allows programmer to create a general or a base class first and then later extend it to more specialized class. It allows programmer to write better code.
Using inheritance you can use or inherit all the data fields and methods available in your base class. Later you can add you own methods and data fields, thus inheritance provides a way to organize code, rather than rewriting it from scratch.
In object-oriented terminology when class X extend class Y, then Y is called super/parent/base class and X is called subclass/child/derived class. One point to note here is that only data fields and method which are not private are accessible by child classes. Private data fields and methods are accessible only inside the class.
syntax to create a derived class is −
class BaseClass:
Body of base class
class DerivedClass(BaseClass):
Body of derived class
Inheriting Attributes
Now look at the below example −
Output
We first created a class called Date and pass the object as an argument, here-object is built-in class provided by Python. Later we created another class called time and called the Date class as an argument. Through this call we get access to all the data and attributes of Date class into the Time class. Because of that when we try to get the get_date method from the Time class object tm we created earlier possible.
Object.Attribute Lookup Hierarchy
- The instance
- The class
- Any class from which this class inherits
Inheritance Examples
Let’s take a closure look into the inheritance example −
Let’s create couple of classes to participate in examples −
- Animal − Class simulate an animal
- Cat − Subclass of Animal
- Dog − Subclass of Animal
In Python, constructor of class used to create an object (instance), and assign the value for the attributes.
Constructor of subclasses always called to a constructor of parent class to initialize value for the attributes in the parent class, then it start assign value for its attributes.
Output
In the above example, we see the command attributes or methods we put in the parent class so that all subclasses or child classes will inherits that property from the parent class.
If a subclass try to inherits methods or data from another subclass then it will through an error as we see when Dog class try to call swatstring() methods from that cat class, it throws an error(like AttributeError in our case).
Polymorphism (“MANY SHAPES”)
Polymorphism is an important feature of class definition in Python that is utilized when you have commonly named methods across classes or subclasses. This permits functions to use entities of different types at different times. So, it provides flexibility and loose coupling so that code can be extended and easily maintained over time.
This allows functions to use objects of any of these polymorphic classes without needing to be aware of distinctions across the classes.
Polymorphism can be carried out through inheritance, with subclasses making use of base class methods or overriding them.
Let understand the concept of polymorphism with our previous inheritance example and add one common method called show_affection in both subclasses −
From the example we can see, it refers to a design in which object of dissimilar type can be treated in the same manner or more specifically two or more classes with method of the same name or common interface because same method(show_affection in below example) is called with either type of objects.
Output
So, all animals show affections (show_affection), but they do differently. The “show_affection” behaviors is thus polymorphic in the sense that it acted differently depending on the animal. So, the abstract “animal” concept does not actually “show_affection”, but specific animals(like dogs and cats) have a concrete implementation of the action “show_affection”.
Python itself have classes that are polymorphic. Example, the len() function can be used with multiple objects and all return the correct output based on the input parameter.
Overriding
In Python, when a subclass contains a method that overrides a method of the superclass, you can also call the superclass method by calling
Super(Subclass, self).method instead of self.method.
Example
class Thought(object):
def __init__(self):
pass
def message(self):
print("Thought, always come and go")
class Advice(Thought):
def __init__(self):
super(Advice, self).__init__()
def message(self):
print('Warning: Risk is always involved when you are dealing with market!')
Inheriting the Constructor
If we see from our previous inheritance example, __init__ was located in the parent class in the up ‘cause the child class dog or cat didn’t‘ve __init__ method in it. Python used the inheritance attribute lookup to find __init__ in animal class. When we created the child class, first it will look the __init__ method in the dog class, then it didn’t find it then looked into parent class Animal and found there and called that there. So as our class design became complex we may wish to initialize a instance firstly processing it through parent class constructor and then through child class constructor.
Output
In above example- all animals have a name and all dogs a particular breed. We called parent class constructor with super. So dog has its own __init__ but the first thing that happen is we call super. Super is built in function and it is designed to relate a class to its super class or its parent class.
In this case we saying that get the super class of dog and pass the dog instance to whatever method we say here the constructor __init__. So in another words we are calling parent class Animal __init__ with the dog object. You may ask why we won’t just say Animal __init__ with the dog instance, we could do this but if the name of animal class were to change, sometime in the future. What if we wanna rearrange the class hierarchy,so the dog inherited from another class. Using super in this case allows us to keep things modular and easy to change and maintain.
So in this example we are able to combine general __init__ functionality with more specific functionality. This gives us opportunity to separate common functionality from the specific functionality which can eliminate code duplication and relate class to one another in a way that reflects the system overall design.
Conclusion
__init__ is like any other method; it can be inherited
If a class does not have a __init__ constructor, Python will check its parent class to see if it can find one.
As soon as it finds one, Python calls it and stops looking
We can use the super () function to call methods in the parent class.
We may want to initialize in the parent as well as our own class.
Multiple Inheritance and the Lookup Tree
As its name indicates, multiple inheritance is Python is when a class inherits from multiple classes.
For example, a child inherits personality traits from both parents (Mother and Father).
Python Multiple Inheritance Syntax
To make a class inherits from multiple parents classes, we write the the names of these classes inside the parentheses to the derived class while defining it. We separate these names with comma.
Below is an example of that −
>>> class Mother:
pass
>>> class Father:
pass
>>> class Child(Mother, Father):
pass
>>> issubclass(Child, Mother) and issubclass(Child, Father)
True
Multiple inheritance refers to the ability of inheriting from two or more than two class. The complexity arises as child inherits from parent and parents inherits from the grandparent class. Python climbs an inheriting tree looking for attributes that is being requested to be read from an object. It will check the in the instance, within class then parent class and lastly from the grandparent class. Now the question arises in what order the classes will be searched - breath-first or depth-first. By default, Python goes with the depth-first.
That’s is why in the below diagram the Python searches the dothis() method first in class A. So the method resolution order in the below example will be
Mro- D→B→A→C
Look at the below multiple inheritance diagram −
Let’s go through an example to understand the “mro” feature of an Python.
Output
Example 3
Let’s take another example of “diamond shape” multiple inheritance.
Above diagram will be considered ambiguous. From our previous example understanding “method resolution order” .i.e. mro will be D→B→A→C→A but it’s not. On getting the second A from the C, Python will ignore the previous A. so the mro will be in this case will be D→B→C→A.
Let’s create an example based on above diagram −
Output
Simple rule to understand the above output is- if the same class appear in the method resolution order, the earlier appearances of this class will be remove from the method resolution order.
In conclusion −
Any class can inherit from multiple classes
Python normally uses a “depth-first” order when searching inheriting classes.
But when two classes inherit from the same class, Python eliminates the first appearances of that class from the mro.
Decorators, Static and Class Methods
Functions(or methods) are created by def statement.
Though methods works in exactly the same way as a function except one point where method first argument is instance object.
We can classify methods based on how they behave, like
Simple method − defined outside of a class. This function can access class attributes by feeding instance argument:
def outside_func(():
Instance method −
def func(self,)
Class method − if we need to use class attributes
@classmethod
def cfunc(cls,)
Static method − do not have any info about the class
@staticmethod
def sfoo()
Till now we have seen the instance method, now is the time to get some insight into the other two methods,
Class Method
The @classmethod decorator, is a builtin function decorator that gets passed the class it was called on or the class of the instance it was called on as first argument. The result of that evaluation shadows your function definition.
syntax
class C(object):
@classmethod
def fun(cls, arg1, arg2, ...):
....
fun: function that needs to be converted into a class method
returns: a class method for function
They have the access to this cls argument, it can’t modify object instance state. That would require access to self.
It is bound to the class and not the object of the class.
Class methods can still modify class state that applies across all instances of the class.
Static Method
A static method takes neither a self nor a cls(class) parameter but it’s free to accept an arbitrary number of other parameters.
syntax
class C(object):
@staticmethod
def fun(arg1, arg2, ...):
...
returns: a static method for function funself.
- A static method can neither modify object state nor class state.
- They are restricted in what data they can access.
When to use what
We generally use class method to create factory methods. Factory methods return class object (similar to a constructor) for different use cases.
We generally use static methods to create utility functions.
Python Design Pattern
Overview
Modern software development needs to address complex business requirements. It also needs to take into account factors such as future extensibility and maintainability. A good design of a software system is vital to accomplish these goals. Design patterns play an important role in such systems.
To understand design pattern, let’s consider below example −
Every car’s design follows a basic design pattern, four wheels, steering wheel, the core drive system like accelerator-break-clutch, etc.
So, all things repeatedly built/ produced, shall inevitably follow a pattern in its design.. it cars, bicycle, pizza, atm machines, whatever…even your sofa bed.
Designs that have almost become standard way of coding some logic/mechanism/technique in software, hence come to be known as or studied as, Software Design Patterns.
Why is Design Pattern Important?
Benefits of using Design Patterns are −
Helps you to solve common design problems through a proven approach.
No ambiguity in the understanding as they are well documented.
Reduce the overall development time.
Helps you deal with future extensions and modifications with more ease than otherwise.
May reduce errors in the system since they are proven solutions to common problems.
Classification of Design Patterns
The GoF (Gang of Four) design patterns are classified into three categories namely creational, structural and behavioral.
Creational Patterns
Creational design patterns separate the object creation logic from the rest of the system. Instead of you creating objects, creational patterns creates them for you. The creational patterns include Abstract Factory, Builder, Factory Method, Prototype and Singleton.
Creational Patterns are not commonly used in Python because of the dynamic nature of the language. Also language itself provide us with all the flexibility we need to create in a sufficient elegant fashion, we rarely need to implement anything on top, like singleton or Factory.
Also these patterns provide a way to create objects while hiding the creation logic, rather than instantiating objects directly using a new operator.
Structural Patterns
Sometimes instead of starting from scratch, you need to build larger structures by using an existing set of classes. That’s where structural class patterns use inheritance to build a new structure. Structural object patterns use composition/ aggregation to obtain a new functionality. Adapter, Bridge, Composite, Decorator, Façade, Flyweight and Proxy are Structural Patterns. They offers best ways to organize class hierarchy.
Behavioral Patterns
Behavioral patterns offers best ways of handling communication between objects. Patterns comes under this categories are: Visitor, Chain of responsibility, Command, Interpreter, Iterator, Mediator, Memento, Observer, State, Strategy and Template method are Behavioral Patterns.
Because they represent the behavior of a system, they are used generally to describe the functionality of software systems.
Commonly used Design Patterns
Singleton
It is one of the most controversial and famous of all design patterns. It is used in overly object-oriented languages, and is a vital part of traditional object-oriented programming.
The Singleton pattern is used for,
When logging needs to be implemented. The logger instance is shared by all the components of the system.
The configuration files is using this because cache of information needs to be maintained and shared by all the various components in the system.
Managing a connection to a database.
Here is the UML diagram,
class Logger(object):
def __new__(cls, *args, **kwargs):
if not hasattr(cls, '_logger'):
cls._logger = super(Logger, cls).__new__(cls, *args, **kwargs)
return cls._logger
In this example, Logger is a Singleton.
When __new__ is called, it normally constructs a new instance of that class. When we override it, we first check if our singleton instance has been created or not. If not, we create it using a super call. Thus, whenever we call the constructor on Logger, we always get the exact same instance.
>>>
>>> obj1 = Logger()
>>> obj2 = Logger()
>>> obj1 == obj2
True
>>>
>>> obj1
<__main__.Logger object at 0x03224090>
>>> obj2
<__main__.Logger object at 0x03224090>
Object Oriented Python - Advanced Features
In this we will look into some of the advanced features which Python provide
Core Syntax in our Class design
In this we will look onto, how Python allows us to take advantage of operators in our classes. Python is largely objects and methods call on objects and this even goes on even when its hidden by some convenient syntax.
>>> var1 = 'Hello'
>>> var2 = ' World!'
>>> var1 + var2
'Hello World!'
>>>
>>> var1.__add__(var2)
'Hello World!'
>>> num1 = 45
>>> num2 = 60
>>> num1.__add__(num2)
105
>>> var3 = ['a', 'b']
>>> var4 = ['hello', ' John']
>>> var3.__add__(var4)
['a', 'b', 'hello', ' John']
So if we have to add magic method __add__ to our own classes, could we do that too. Let’s try to do that.
We have a class called Sumlist which has a contructor __init__ which takes list as an argument called my_list.
class SumList(object):
def __init__(self, my_list):
self.mylist = my_list
def __add__(self, other):
new_list = [ x + y for x, y in zip(self.mylist, other.mylist)]
return SumList(new_list)
def __repr__(self):
return str(self.mylist)
aa = SumList([3,6, 9, 12, 15])
bb = SumList([100, 200, 300, 400, 500])
cc = aa + bb # aa.__add__(bb)
print(cc) # should gives us a list ([103, 206, 309, 412, 515])
Output
[103, 206, 309, 412, 515]
But there are many methods which are internally managed by others magic methods. Below are some of them,
'abc' in var # var.__contains__('abc')
var == 'abc' # var.__eq__('abc')
var[1] # var.__getitem__(1)
var[1:3] # var.__getslice__(1, 3)
len(var) # var.__len__()
print(var) # var.__repr__()
Inheriting From built-in types
Classes can also inherit from built-in types this means inherits from any built-in and take advantage of all the functionality found there.
In below example we are inheriting from dictionary but then we are implementing one of its method __setitem__. This (setitem) is invoked when we set key and value in the dictionary. As this is a magic method, this will be called implicitly.
class MyDict(dict):
def __setitem__(self, key, val):
print('setting a key and value!')
dict.__setitem__(self, key, val)
dd = MyDict()
dd['a'] = 10
dd['b'] = 20
for key in dd.keys():
print('{0} = {1}'.format(key, dd[key]))
Output
setting a key and value!
setting a key and value!
a = 10
b = 20
Let’s extend our previous example, below we have called two magic methods called __getitem__ and __setitem__ better invoked when we deal with list index.
# Mylist inherits from 'list' object but indexes from 1 instead for 0!
class Mylist(list): # inherits from list
def __getitem__(self, index):
if index == 0:
raise IndexError
if index > 0:
index = index - 1
return list.__getitem__(self, index) # this method is called when
# we access a value with subscript like x[1]
def __setitem__(self, index, value):
if index == 0:
raise IndexError
if index > 0:
index = index - 1
list.__setitem__(self, index, value)
x = Mylist(['a', 'b', 'c']) # __init__() inherited from builtin list
print(x) # __repr__() inherited from builtin list
x.append('HELLO'); # append() inherited from builtin list
print(x[1]) # 'a' (Mylist.__getitem__ cutomizes list superclass
# method. index is 1, but reflects 0!
print (x[4]) # 'HELLO' (index is 4 but reflects 3!
Output
['a', 'b', 'c']
a
HELLO
In above example, we set a three item list in Mylist and implicitly __init__ method is called and when we print the element x, we get the three item list ([‘a’,’b’,’c’]). Then we append another element to this list. Later we ask for index 1 and index 4. But if you see the output, we are getting element from the (index-1) what we have asked for. As we know list indexing start from 0 but here the indexing start from 1 (that’s why we are getting the first item of the list).
Naming Conventions
In this we will look into names we’ll used for variables especially private variables and conventions used by Python programmers worldwide. Although variables are designated as private but there is not privacy in Python and this by design. Like any other well documented languages, Python has naming and style conventions that it promote although it doesn’t enforce them. There is a style guide written by “Guido van Rossum” the originator of Python, that describe the best practices and use of name and is called PEP8. Here is the link for this, https://www.python.org/dev/peps/pep-0008/
PEP stands for Python enhancement proposal and is a series of documentation that distributed among the Python community to discuss proposed changes. For example it is recommended all,
- Module names − all_lower_case
- Class names and exception names − CamelCase
- Global and local names − all_lower_case
- Functions and method names − all_lower_case
- Constants − ALL_UPPER_CASE
These are just the recommendation, you can vary if you like. But as most of the developers follows these recommendation so might me your code is less readable.
Why conform to convention?
We can follow the PEP recommendation we it allows us to get,
- More familiar to the vast majority of developers
- Clearer to most readers of your code.
- Will match style of other contributers who work on same code base.
- Mark of a professional software developers
- Everyone will accept you.
Variable Naming − ‘Public’ and ‘Private’
In Python, when we are dealing with modules and classes, we designate some variables or attribute as private. In Python, there is no existence of “Private” instance variable which cannot be accessed except inside an object. Private simply means they are simply not intended to be used by the users of the code instead they are intended to be used internally. In general, a convention is being followed by most Python developers i.e. a name prefixed with an underscore for example. _attrval (example below) should be treated as a non-public part of the API or any Python code, whether it is a function, a method or a data member. Below is the naming convention we follow,
Public attributes or variables (intended to be used by the importer of this module or user of this class) −regular_lower_case
Private attributes or variables (internal use by the module or class) −_single_leading_underscore
Private attributes that shouldn’t be subclassed −__double_leading_underscore
Magic attributes −__double_underscores__(use them, don’t create them)
class GetSet(object):
instance_count = 0 # public
__mangled_name = 'no privacy!' # special variable
def __init__(self, value):
self._attrval = value # _attrval is for internal use only
GetSet.instance_count += 1
@property
def var(self):
print('Getting the "var" attribute')
return self._attrval
@var.setter
def var(self, value):
print('setting the "var" attribute')
self._attrval = value
@var.deleter
def var(self):
print('deleting the "var" attribute')
self._attrval = None
cc = GetSet(5)
cc.var = 10 # public name
print(cc._attrval)
print(cc._GetSet__mangled_name)
Output
setting the "var" attribute
10
no privacy!
Object Oriented Python - Files and Strings
Strings
Strings are the most popular data types used in every programming language. Why? Because we, understand text better than numbers, so in writing and talking we use text and words, similarly in programming too we use strings. In string we parse text, analyse text semantics, and do data mining – and all this data is human consumed text.The string in Python is immutable.
String Manipulation
In Python, string can be marked in multiple ways, using single quote ( ‘ ), double quote( “ ) or even triple quote ( ‘’’ ) in case of multiline strings.
>>> # String Examples
>>> a = "hello"
>>> b = ''' A Multi line string,
Simple!'''
>>> e = ('Multiple' 'strings' 'togethers')
String manipulation is very useful and very widely used in every language. Often, programmers are required to break down strings and examine them closely.
Strings can be iterated over (character by character), sliced, or concatenated. The syntax is the same as for lists.
The str class has numerous methods on it to make manipulating strings easier. The dir and help commands provides guidance in the Python interpreter how to use them.
Below are some of the commonly used string methods we use.
Sr.No.
Method & Description
1
isalpha()
Checks if all characters are Alphabets
2
isdigit()
Checks Digit Characters
3
isdecimal()
Checks decimal Characters
4
isnumeric()
checks Numeric Characters
5
find()
Returns the Highest Index of substrings
6
istitle()
Checks for Titlecased strings
7
join()
Returns a concatenated string
8
lower()
returns lower cased string
9
upper()
returns upper cased string
10
partion()
Returns a tuple
11
bytearray()
Returns array of given byte size
12
enumerate()
Returns an enumerate object
13
isprintable()
Checks printable character
Let’s try to run couple of string methods,
>>> str1 = 'Hello World!'
>>> str1.startswith('h')
False
>>> str1.startswith('H')
True
>>> str1.endswith('d')
False
>>> str1.endswith('d!')
True
>>> str1.find('o')
4
>>> #Above returns the index of the first occurence of the character/substring.
>>> str1.find('lo')
3
>>> str1.upper()
'HELLO WORLD!'
>>> str1.lower()
'hello world!'
>>> str1.index('b')
Traceback (most recent call last):
File "<pyshell#19>", line 1, in <module>
str1.index('b')
ValueError: substring not found
>>> s = ('hello How Are You')
>>> s.split(' ')
['hello', 'How', 'Are', 'You']
>>> s1 = s.split(' ')
>>> '*'.join(s1)
'hello*How*Are*You'
>>> s.partition(' ')
('hello', ' ', 'How Are You')
>>>
String Formatting
In Python 3.x formatting of strings has changed, now it more logical and is more flexible. Formatting can be done using the format() method or the % sign(old style) in format string.
The string can contain literal text or replacement fields delimited by braces {} and each replacement field may contains either the numeric index of a positional argument or the name of a keyword argument.
syntax
str.format(*args, **kwargs)
Basic Formatting
>>> '{} {}'.format('Example', 'One')
'Example One'
>>> '{} {}'.format('pie', '3.1415926')
'pie 3.1415926'
Below example allows re-arrange the order of display without changing the arguments.
>>> '{1} {0}'.format('pie', '3.1415926')
'3.1415926 pie'
Padding and aligning strings
A value can be padded to a specific length.
>>> #Padding Character, can be space or special character
>>> '{:12}'.format('PYTHON')
'PYTHON '
>>> '{:>12}'.format('PYTHON')
' PYTHON'
>>> '{:<{}s}'.format('PYTHON',12)
'PYTHON '
>>> '{:*<12}'.format('PYTHON')
'PYTHON******'
>>> '{:*^12}'.format('PYTHON')
'***PYTHON***'
>>> '{:.15}'.format('PYTHON OBJECT ORIENTED PROGRAMMING')
'PYTHON OBJECT O'
>>> #Above, truncated 15 characters from the left side of a specified string
>>> '{:.{}}'.format('PYTHON OBJECT ORIENTED',15)
'PYTHON OBJECT O'
>>> #Named Placeholders
>>> data = {'Name':'Raghu', 'Place':'Bangalore'}
>>> '{Name} {Place}'.format(**data)
'Raghu Bangalore'
>>> #Datetime
>>> from datetime import datetime
>>> '{:%Y/%m/%d.%H:%M}'.format(datetime(2018,3,26,9,57))
'2018/03/26.09:57'
Strings are Unicode
Strings as collections of immutable Unicode characters. Unicode strings provide an opportunity to create software or programs that works everywhere because the Unicode strings can represent any possible character not just the ASCII characters.
Many IO operations only know how to deal with bytes, even if the bytes object refers to textual data. It is therefore very important to know how to interchange between bytes and Unicode.
Converting text to bytes
Converting a strings to byte object is termed as encoding. There are numerous forms of encoding, most common ones are: PNG; JPEG, MP3, WAV, ASCII, UTF-8 etc. Also this(encoding) is a format to represent audio, images, text, etc. in bytes.
This conversion is possible through encode(). It take encoding technique as argument. By default, we use ‘UTF-8’ technique.
>>> # Python Code to demonstrate string encoding
>>>
>>> # Initialising a String
>>> x = 'TutorialsPoint'
>>>
>>> #Initialising a byte object
>>> y = b'TutorialsPoint'
>>>
>>> # Using encode() to encode the String >>> # encoded version of x is stored in z using ASCII mapping
>>> z = x.encode('ASCII')
>>>
>>> # Check if x is converted to bytes or not
>>>
>>> if(z==y):
print('Encoding Successful!')
else:
print('Encoding Unsuccessful!')
Encoding Successful!
Converting bytes to text
Converting bytes to text is called the decoding. This is implemented through decode(). We can convert a byte string to a character string if we know which encoding is used to encode it.
So Encoding and decoding are inverse processes.
>>>
>>> # Python code to demonstrate Byte Decoding
>>>
>>> #Initialise a String
>>> x = 'TutorialsPoint'
>>>
>>> #Initialising a byte object
>>> y = b'TutorialsPoint'
>>>
>>> #using decode() to decode the Byte object
>>> # decoded version of y is stored in z using ASCII mapping
>>> z = y.decode('ASCII')
>>> #Check if y is converted to String or not
>>> if (z == x):
print('Decoding Successful!')
else:
print('Decoding Unsuccessful!') Decoding Successful!
>>>
File I/O
Operating systems represents files as a sequence of bytes, not text.
A file is a named location on disk to store related information. It is used to permanently store data in your disk.
In Python, a file operation takes place in the following order.
- Open a file
- Read or write onto a file (operation).Open a file
- Close the file.
Python wraps the incoming (or outgoing) stream of bytes with appropriate decode (or encode) calls so we can deal directly with str objects.
Opening a file
Python has a built-in function open() to open a file. This will generate a file object, also called a handle as it is used to read or modify the file accordingly.
>>> f = open(r'c:\users\rajesh\Desktop\index.webm','rb')
>>> f
<_io.BufferedReader name='c:\\users\\rajesh\\Desktop\\index.webm'>
>>> f.mode
'rb'
>>> f.name
'c:\\users\\rajesh\\Desktop\\index.webm'
For reading text from a file, we only need to pass the filename into the function. The file will be opened for reading, and the bytes will be converted to text using the platform default encoding.
Exception and Exception Classes
In general, an exception is any unusual condition. Exception usually indicates errors but sometimes they intentionally puts in the program, in cases like terminating a procedure early or recovering from a resource shortage. There are number of built-in exceptions, which indicate conditions like reading past the end of a file, or dividing by zero. We can define our own exceptions called custom exception.
Exception handling enables you handle errors gracefully and do something meaningful about it. Exception handling has two components: “throwing” and ‘catching’.
Identifying Exception (Errors)
Every error occurs in Python result an exception which will an error condition identified by its error type.
>>> #Exception
>>> 1/0
Traceback (most recent call last):
File "<pyshell#2>", line 1, in <module>
1/0
ZeroDivisionError: division by zero
>>>
>>> var = 20
>>> print(ver)
Traceback (most recent call last):
File "<pyshell#5>", line 1, in <module>
print(ver)
NameError: name 'ver' is not defined
>>> #Above as we have misspelled a variable name so we get an NameError.
>>>
>>> print('hello)
SyntaxError: EOL while scanning string literal
>>> #Above we have not closed the quote in a string, so we get SyntaxError.
>>>
>>> #Below we are asking for a key, that doen't exists.
>>> mydict = {}
>>> mydict['x']
Traceback (most recent call last):
File "<pyshell#15>", line 1, in <module>
mydict['x']
KeyError: 'x'
>>> #Above keyError
>>>
>>> #Below asking for a index that didn't exist in a list.
>>> mylist = [1,2,3,4]
>>> mylist[5]
Traceback (most recent call last):
File "<pyshell#20>", line 1, in <module>
mylist[5]
IndexError: list index out of range
>>> #Above, index out of range, raised IndexError.
Catching/Trapping Exception
When something unusual occurs in your program and you wish to handle it using the exception mechanism, you ‘throw an exception’. The keywords try and except are used to catch exceptions. Whenever an error occurs within a try block, Python looks for a matching except block to handle it. If there is one, execution jumps there.
syntax
try:
#write some code
#that might throw some exception
except <ExceptionType>:
# Exception handler, alert the user
The code within the try clause will be executed statement by statement.
If an exception occurs, the rest of the try block will be skipped and the except clause will be executed.
try:
some statement here
except:
exception handling
Let’s write some code to see what happens when you not use any error handling mechanism in your program.
number = int(input('Please enter the number between 1 & 10: '))
print('You have entered number',number)
Above programme will work correctly as long as the user enters a number, but what happens if the users try to puts some other data type(like a string or a list).
Please enter the number between 1 > 10: 'Hi'
Traceback (most recent call last):
File "C:/Python/Python361/exception2.py", line 1, in <module>
number = int(input('Please enter the number between 1 & 10: '))
ValueError: invalid literal for int() with base 10: "'Hi'"
Now ValueError is an exception type. Let’s try to rewrite the above code with exception handling.
import sys
print('Previous code with exception handling')
try:
number = int(input('Enter number between 1 > 10: '))
except(ValueError):
print('Error..numbers only')
sys.exit()
print('You have entered number: ',number)
If we run the program, and enter a string (instead of a number), we can see that we get a different result.
Previous code with exception handling
Enter number between 1 > 10: 'Hi'
Error..numbers only
Raising Exceptions
To raise your exceptions from your own methods you need to use raise keyword like this
raise ExceptionClass(‘Some Text Here’)
Let’s take an example
def enterAge(age):
if age<0:
raise ValueError('Only positive integers are allowed')
if age % 2 ==0:
print('Entered Age is even')
else:
print('Entered Age is odd')
try:
num = int(input('Enter your age: '))
enterAge(num)
except ValueError:
print('Only positive integers are allowed')
Run the program and enter positive integer.
Expected Output
Enter your age: 12
Entered Age is even
But when we try to enter a negative number we get,
Expected Output
Enter your age: -2
Only positive integers are allowed
Creating Custom exception class
You can create a custom exception class by Extending BaseException class or subclass of BaseException.
From above diagram we can see most of the exception classes in Python extends from the BaseException class. You can derive your own exception class from BaseException class or from its subclass.
Create a new file called NegativeNumberException.py and write the following code.
class NegativeNumberException(RuntimeError):
def __init__(self, age):
super().__init__()
self.age = age
Above code creates a new exception class named NegativeNumberException, which consists of only constructor which call parent class constructor using super()__init__() and sets the age.
Now to create your own custom exception class, will write some code and import the new exception class.
from NegativeNumberException import NegativeNumberException
def enterage(age):
if age < 0:
raise NegativeNumberException('Only positive integers are allowed')
if age % 2 == 0:
print('Age is Even')
else:
print('Age is Odd')
try:
num = int(input('Enter your age: '))
enterage(num)
except NegativeNumberException:
print('Only positive integers are allowed')
except:
print('Something is wrong')
Output
Enter your age: -2
Only positive integers are allowed
Another way to create a custom Exception class.
class customException(Exception):
def __init__(self, value):
self.parameter = value
def __str__(self):
return repr(self.parameter)
try:
raise customException('My Useful Error Message!')
except customException as instance:
print('Caught: ' + instance.parameter)
Output
Caught: My Useful Error Message!
Exception hierarchy
The class hierarchy for built-in exceptions is −
+-- SystemExit
+-- KeyboardInterrupt
+-- GeneratorExit
+-- Exception
+-- StopIteration
+-- StopAsyncIteration
+-- ArithmeticError
| +-- FloatingPointError
| +-- OverflowError
| +-- ZeroDivisionError
+-- AssertionError
+-- AttributeError
+-- BufferError
+-- EOFError
+-- ImportError
+-- LookupError
| +-- IndexError
| +-- KeyError
+-- MemoryError
+-- NameError
| +-- UnboundLocalError
+-- OSError
| +-- BlockingIOError
| +-- ChildProcessError
| +-- ConnectionError
| | +-- BrokenPipeError
| | +-- ConnectionAbortedError
| | +-- ConnectionRefusedError
| | +-- ConnectionResetError
| +-- FileExistsError
| +-- FileNotFoundError
| +-- InterruptedError
| +-- IsADirectoryError
| +-- NotADirectoryError
| +-- PermissionError
| +-- ProcessLookupError
| +-- TimeoutError
+-- ReferenceError
+-- RuntimeError
| +-- NotImplementedError
| +-- RecursionError
+-- SyntaxError
| +-- IndentationError
| +-- TabError
+-- SystemError
+-- TypeError
+-- ValueError
| +-- UnicodeError
| +-- UnicodeDecodeError
| +-- UnicodeEncodeError
| +-- UnicodeTranslateError
+-- Warning
+-- DeprecationWarning
+-- PendingDeprecationWarning
+-- RuntimeWarning
+-- SyntaxWarning
+-- UserWarning
+-- FutureWarning
+-- ImportWarning
+-- UnicodeWarning
+-- BytesWarning
+-- ResourceWarning
Object Oriented Python - Object Serialization
In the context of data storage, serialization is the process of translating data structures or object state into a format that can be stored (for example, in a file or memory buffer) or transmitted and reconstructed later.
In serialization, an object is transformed into a format that can be stored, so as to be able to deserialize it later and recreate the original object from the serialized format.
Pickle
Pickling is the process whereby a Python object hierarchy is converted into a byte stream (usually not human readable) to be written to a file, this is also known as Serialization. Unpickling is the reverse operation, whereby a byte stream is converted back into a working Python object hierarchy.
Pickle is operationally simplest way to store the object. The Python Pickle module is an object-oriented way to store objects directly in a special storage format.
What can it do?
- Pickle can store and reproduce dictionaries and lists very easily.
- Stores object attributes and restores them back to the same State.
What pickle can’t do?
- It does not save an objects code. Only it’s attributes values.
- It cannot store file handles or connection sockets.
In short we can say, pickling is a way to store and retrieve data variables into and out from files where variables can be lists, classes, etc.
To Pickle something you must −
- import pickle
- Write a variable to file, something like
pickle.dump(mystring, outfile, protocol),
where 3rd argument protocol is optional To unpickling something you must −
Import pickle
Write a variable to a file, something like
myString = pickle.load(inputfile)
Methods
The pickle interface provides four different methods.
dump() − The dump() method serializes to an open file (file-like object).
dumps() − Serializes to a string
load() − Deserializes from an open-like object.
loads() − Deserializes from a string.
Based on above procedure, below is an example of “pickling”.
Output
My Cat pussy is White and has 4 legs
Would you like to see her pickled? Here she is!
b'\x80\x03c__main__\nCat\nq\x00)\x81q\x01}q\x02(X\x0e\x00\x00\x00number_of_legsq\x03K\x04X\x05\x00\x00\x00colorq\x04X\x05\x00\x00\x00Whiteq\x05ub.'
So, in the example above, we have created an instance of a Cat class and then we’ve pickled it, transforming our “Cat” instance into a simple array of bytes.
This way we can easily store the bytes array on a binary file or in a database field and restore it back to its original form from our storage support in a later time.
Also if you want to create a file with a pickled object, you can use the dump() method ( instead of the dumps*()* one) passing also an opened binary file and the pickling result will be stored in the file automatically.
[….]
binary_file = open(my_pickled_Pussy.bin', mode='wb')
my_pickled_Pussy = pickle.dump(Pussy, binary_file)
binary_file.close()
Unpickling
The process that takes a binary array and converts it to an object hierarchy is called unpickling.
The unpickling process is done by using the load() function of the pickle module and returns a complete object hierarchy from a simple bytes array.
Let’s use the load function in our previous example.
Output
MeOw is black
Pussy is white
JSON
JSON(JavaScript Object Notation) has been part of the Python standard library is a lightweight data-interchange format. It is easy for humans to read and write. It is easy to parse and generate.
Because of its simplicity, JSON is a way by which we store and exchange data, which is accomplished through its JSON syntax, and is used in many web applications. As it is in human readable format, and this may be one of the reasons for using it in data transmission, in addition to its effectiveness when working with APIs.
An example of JSON-formatted data is as follow −
{"EmployID": 40203, "Name": "Zack", "Age":54, "isEmployed": True}
Python makes it simple to work with Json files. The module sused for this purpose is the JSON module. This module should be included (built-in) within your Python installation.
So let’s see how can we convert Python dictionary to JSON and write it to a text file.
JSON to Python
Reading JSON means converting JSON into a Python value (object). The json library parses JSON into a dictionary or list in Python. In order to do that, we use the loads() function (load from a string), as follow −
Output
Below is one sample json file,
data1.json
{"menu": {
"id": "file",
"value": "File",
"popup": {
"menuitem": [
{"value": "New", "onclick": "CreateNewDoc()"},
{"value": "Open", "onclick": "OpenDoc()"},
{"value": "Close", "onclick": "CloseDoc()"}
]
}
}}
Above content (Data1.json) looks like a conventional dictionary. We can use pickle to store this file but the output of it is not human readable form.
JSON(Java Script Object Notification) is a very simple format and that’s one of the reason for its popularity. Now let’s look into json output through below program.
Output
Above we open the json file (data1.json) for reading, obtain the file handler and pass on to json.load and getting back the object. When we try to print the output of the object, its same as the json file. Although the type of the object is dictionary, it comes out as a Python object. Writing to the json is simple as we saw this pickle. Above we load the json file, add another key value pair and writing it back to the same json file. Now if we see out data1.json, it looks different .i.e. not in the same format as we see previously.
To make our Output looks same (human readable format), add the couple of arguments into our last line of the program,
json.dump(conf, fh, indent = 4, separators = (‘,’, ‘: ‘))
Similarly like pickle, we can print the string with dumps and load with loads. Below is an example of that,
YAML
YAML may be the most human friendly data serialization standard for all programming languages.
Python yaml module is called pyaml
YAML is an alternative to JSON −
Human readable code − YAML is the most human readable format so much so that even its front-page content is displayed in YAML to make this point.
Compact code − In YAML we use whitespace indentation to denote structure not brackets.
Syntax for relational data − For internal references we use anchors (&) and aliases (*).
One of the area where it is used widely is for viewing/editing of data structures − for example configuration files, dumping during debugging and document headers.
Installing YAML
As yaml is not a built-in module, we need to install it manually. Best way to install yaml on windows machine is through pip. Run below command on your windows terminal to install yaml,
pip install pyaml (Windows machine)
sudo pip install pyaml (*nix and Mac)
On running above command, screen will display something like below based on what’s the current latest version.
Collecting pyaml
Using cached pyaml-17.12.1-py2.py3-none-any.whl
Collecting PyYAML (from pyaml)
Using cached PyYAML-3.12.tar.gz
Installing collected packages: PyYAML, pyaml
Running setup.py install for PyYAML ... done
Successfully installed PyYAML-3.12 pyaml-17.12.1
To test it, go to the Python shell and import the yaml module, import yaml, if no error is found, then we can say installation is successful.
After installing pyaml, let’s look at below code,
script_yaml1.py
Above we created three different data structure, dictionary, list and tuple. On each of the structure, we do yaml.dump. Important point is how the output is displayed on the screen.
Output
Dictionary output looks clean .ie. key: value.
White space to separate different objects.
List is notated with dash (-)
Tuple is indicated first with !!Python/tuple and then in the same format as lists.
Loading a yaml file
So let’s say I have one yaml file, which contains,
---
# An employee record
name: Raagvendra Joshi
job: Developer
skill: Oracle
employed: True
foods:
- Apple
- Orange
- Strawberry
- Mango
languages:
Oracle: Elite
power_builder: Elite
Full Stack Developer: Lame
education:
4 GCSEs
3 A-Levels
MCA in something called com
Now let’s write a code to load this yaml file through yaml.load function. Below is code for the same.
As the output doesn’t looks that much readable, I prettify it by using json in the end. Compare the output we got and the actual yaml file we have.
Output
One of the most important aspect of software development is debugging. In this section we’ll see different ways of Python debugging either with built-in debugger or third party debuggers.
PDB – The Python Debugger
The module PDB supports setting breakpoints. A breakpoint is an intentional pause of the program, where you can get more information about the programs state.
To set a breakpoint, insert the line
pdb.set_trace()
Example
pdb_example1.py
import pdb
x = 9
y = 7
pdb.set_trace()
total = x + y
pdb.set_trace()
We have inserted a few breakpoints in this program. The program will pause at each breakpoint (pdb.set_trace()). To view a variables contents simply type the variable name.
c:\Python\Python361>Python pdb_example1.py
> c:\Python\Python361\pdb_example1.py(8)<module>()
-> total = x + y
(Pdb) x
9
(Pdb) y
7
(Pdb) total
*** NameError: name 'total' is not defined
(Pdb)
Press c or continue to go on with the programs execution until the next breakpoint.
(Pdb) c
--Return--
> c:\Python\Python361\pdb_example1.py(8)<module>()->None
-> total = x + y
(Pdb) total
16
Eventually, you will need to debug much bigger programs – programs that use subroutines. And sometimes, the problem that you’re trying to find will lie inside a subroutine. Consider the following program.
import pdb
def squar(x, y):
out_squared = x^2 + y^2
return out_squared
if __name__ == "__main__":
#pdb.set_trace()
print (squar(4, 5))
Now on running the above program,
c:\Python\Python361>Python pdb_example2.py
> c:\Python\Python361\pdb_example2.py(10)<module>()
-> print (squar(4, 5))
(Pdb)
We can use ? to get help, but the arrow indicates the line that’s about to be executed. At this point it’s helpful to hit s to s to step into that line.
(Pdb) s
--Call--
>c:\Python\Python361\pdb_example2.py(3)squar()
-> def squar(x, y):
This is a call to a function. If you want an overview of where you are in your code, try l −
(Pdb) l
1 import pdb
2
3 def squar(x, y):
4 -> out_squared = x^2 + y^2
5
6 return out_squared
7
8 if __name__ == "__main__":
9 pdb.set_trace()
10 print (squar(4, 5))
[EOF]
(Pdb)
You can hit n to advance to the next line. At this point you are inside the out_squared method and you have access to the variable declared inside the function .i.e. x and y.
(Pdb) x
4
(Pdb) y
5
(Pdb) x^2
6
(Pdb) y^2
7
(Pdb) x**2
16
(Pdb) y**2
25
(Pdb)
So we can see the ^ operator is not what we wanted instead we need to use ** operator to do squares.
This way we can debug our program inside the functions/methods.
Logging
The logging module has been a part of Python’s Standard Library since Python version 2.3. As it’s a built-in module all Python module can participate in logging, so that our application log can include your own message integrated with messages from third party module. It provides a lot of flexibility and functionality.
Benefits of Logging
Diagnostic logging − It records events related to the application’s operation.
Audit logging − It records events for business analysis.
Messages are written and logged at levels of “severity” &minu
DEBUG (debug()) − diagnostic messages for development.
INFO (info()) − standard “progress” messages.
WARNING (warning()) − detected a non-serious issue.
ERROR (error()) − encountered an error, possibly serious.
CRITICAL (critical()) − usually a fatal error (program stops).
Let’s looks into below simple program,
import logging
logging.basicConfig(level=logging.INFO)
logging.debug('this message will be ignored') # This will not print
logging.info('This should be logged') # it'll print
logging.warning('And this, too') # It'll print
Above we are logging messages on severity level. First we import the module, call basicConfig and set the logging level. Level we set above is INFO. Then we have three different statement: debug statement, info statement and a warning statement.
Output of logging1.py
INFO:root:This should be logged
WARNING:root:And this, too
As the info statement is below debug statement, we are not able to see the debug message. To get the debug statement too in the Output terminal, all we need to change is the basicConfig level.
logging.basicConfig(level = logging.DEBUG)
And in the Output we can see,
DEBUG:root:this message will be ignored
INFO:root:This should be logged
WARNING:root:And this, too
Also the default behavior means if we don’t set any logging level is warning. Just comment out the second line from the above program and run the code.
#logging.basicConfig(level = logging.DEBUG)
Output
WARNING:root:And this, too
Python built in logging level are actually integers.
>>> import logging
>>>
>>> logging.DEBUG
10
>>> logging.CRITICAL
50
>>> logging.WARNING
30
>>> logging.INFO
20
>>> logging.ERROR
40
>>>
We can also save the log messages into the file.
logging.basicConfig(level = logging.DEBUG, filename = 'logging.log')
Now all log messages will go the file (logging.log) in your current working directory instead of the screen. This is a much better approach as it lets us to do post analysis of the messages we got.
We can also set the date stamp with our log message.
logging.basicConfig(level=logging.DEBUG, format = '%(asctime)s %(levelname)s:%(message)s')
Output will get something like,
2018-03-08 19:30:00,066 DEBUG:this message will be ignored
2018-03-08 19:30:00,176 INFO:This should be logged
2018-03-08 19:30:00,201 WARNING:And this, too
Benchmarking
Benchmarking or profiling is basically to test how fast is your code executes and where the bottlenecks are? The main reason to do this is for optimization.
timeit
Python comes with a in-built module called timeit. You can use it to time small code snippets. The timeit module uses platform-specific time functions so that you will get the most accurate timings possible.
So, it allows us to compare two shipment of code taken by each and then optimize the scripts to given better performance.
The timeit module has a command line interface, but it can also be imported.
There are two ways to call a script. Let’s use the script first, for that run the below code and see the Output.
import timeit
print ( 'by index: ', timeit.timeit(stmt = "mydict['c']", setup = "mydict = {'a':5, 'b':10, 'c':15}", number = 1000000))
print ( 'by get: ', timeit.timeit(stmt = 'mydict.get("c")', setup = 'mydict = {"a":5, "b":10, "c":15}', number = 1000000))
Output
by index: 0.1809192126703489
by get: 0.6088525265034692
Above we use two different method .i.e. by subscript and get to access the dictionary key value. We execute statement 1 million times as it executes too fast for a very small data. Now we can see the index access much faster as compared to the get. We can run the code multiply times and there will be slight variation in the time execution to get the better understanding.
Another way is to run the above test in the command line. Let’s do it,
c:\Python\Python361>Python -m timeit -n 1000000 -s "mydict = {'a': 5, 'b':10, 'c':15}" "mydict['c']"
1000000 loops, best of 3: 0.187 usec per loop
c:\Python\Python361>Python -m timeit -n 1000000 -s "mydict = {'a': 5, 'b':10, 'c':15}" "mydict.get('c')"
1000000 loops, best of 3: 0.659 usec per loop
Above output may vary based on your system hardware and what all applications are running currently in your system.
Below we can use the timeit module, if we want to call to a function. As we can add multiple statement inside the function to test.
import timeit
def testme(this_dict, key):
return this_dict[key]
print (timeit.timeit("testme(mydict, key)", setup = "from __main__ import testme; mydict = {'a':9, 'b':18, 'c':27}; key = 'c'", number = 1000000))
Output
0.7713474590139164
Object Oriented Python - Libraries
Requests − Python Requests Module
Requests is a Python module which is an elegant and simple HTTP library for Python. With this you can send all kinds of HTTP requests. With this library we can add headers, form data, multipart files and parameters and access the response data.
As Requests is not a built-in module, so we need to install it first.
You can install it by running the following command in the terminal −
pip install requests
Once you have installed the module, you can verify if the installation is successful by typing below command in the Python shell.
import requests
If the installation has been successful, you won’t see any error message.
Making a GET Request
As a means of example we’ll be using the “pokeapi”
Output −
Making POST Requests
The requests library methods for all of the HTTP verbs currently in use. If you wanted to make a simple POST request to an API endpoint then you can do that like so −
req = requests.post(‘http://api/user’, data = None, json = None)
This would work in exactly the same fashion as our previous GET request, however it features two additional keyword parameters −
data which can be populated with say a dictionary, a file or bytes that will be passed in the HTTP body of our POST request.
json which can be populated with a json object that will be passed in the body of our HTTP request also.
Pandas: Python Library Pandas
Pandas is an open-source Python Library providing high-performance data manipulation and analysis tool using its powerful data structures. Pandas is one of the most widely used Python libraries in data science. It is mainly used for data munging, and with good reason: Powerful and flexible group of functionality.
Built on Numpy package and the key data structure is called the DataFrame. These dataframes allows us to store and manipulate tabular data in rows of observations and columns of variables.
There are several ways to create a DataFrame. One way is to use a dictionary. For example −
Output
From the output we can see new brics DataFrame, Pandas has assigned a key for each country as the numerical values 0 through 4.
If instead of giving indexing values from 0 to 4, we would like to have different index values, say the two letter country code, you can do that easily as well −
Adding below one lines in the above code, gives
brics.index = ['BR', 'RU', 'IN', 'CH', 'SA']
Output
Indexing DataFrames
Output
Pygame
Pygame is the open source and cross-platform library that is for making multimedia applications including games. It includes computer graphics and sound libraries designed to be used with the Python programming language. You can develop many cool games with Pygame.’
Overview
Pygame is composed of various modules, each dealing with a specific set of tasks. For example, the display module deals with the display window and screen, the draw module provides functions to draw shapes and the key module works with the keyboard. These are just some of the modules of the library.
The home of the Pygame library is at https://www.pygame.org/news
To make a Pygame application, you follow these steps −
Import the Pygame library
import pygame
Initialize the Pygame library
pygame.init()
Create a window.
screen = Pygame.display.set_mode((560,480))
Pygame.display.set_caption(‘First Pygame Game’)
Initialize game objects
In this step we load images, load sounds, do object positioning, set up some state variables, etc.
Start the game loop.
It is just a loop where we continuously handle events, checks for input, move objects, and draw them. Each iteration of the loop is called a frame.
Let’s put all the above logic into one below program,
Pygame_script.py
Output
Beautiful Soup: Web Scraping with Beautiful Soup
The general idea behind web scraping is to get the data that exists on a website, and convert it into some format that is usable for analysis.
It’s a Python library for pulling data out of HTML or XML files. With your favourite parser it provide idiomatic ways of navigating, searching and modifying the parse tree.
As BeautifulSoup is not a built-in library, we need to install it before we try to use it. To install BeautifulSoup, run the below command
$ apt-get install Python-bs4 # For Linux and Python2
$ apt-get install Python3-bs4 # for Linux based system and Python3.
$ easy_install beautifulsoup4 # For windows machine,
Or
$ pip instal beatifulsoup4 # For window machine
Once the installation is done, we are ready to run few examples and explores Beautifulsoup in details,
Output
Below are some simple ways to navigate that data structure −
One common task is extracting all the URLs found within a page’s <a> tags −
Another common task is extracting all the text from a page −