Gensim - เอกสารและแบบจำลอง LDA
บทนี้จะกล่าวถึงเอกสารและแบบจำลอง LDA ใน Gensim
การค้นหาจำนวนหัวข้อที่เหมาะสมที่สุดสำหรับ LDA
เราสามารถค้นหาจำนวนหัวข้อที่เหมาะสมที่สุดสำหรับ LDA ได้โดยการสร้างโมเดล LDA จำนวนมากพร้อมค่าต่างๆของหัวข้อ ในบรรดา LDA เหล่านั้นเราสามารถเลือกหนึ่งที่มีค่าการเชื่อมโยงกันสูงสุด
ฟังก์ชั่นต่อไปนี้ชื่อ coherence_values_computation()จะฝึก LDA หลายรุ่น นอกจากนี้ยังจะให้แบบจำลองและคะแนนการเชื่อมโยงที่สอดคล้องกัน -
def coherence_values_computation(dictionary, corpus, texts, limit, start=2, step=3):
coherence_values = []
model_list = []
for num_topics in range(start, limit, step):
model = gensim.models.wrappers.LdaMallet(
mallet_path, corpus=corpus, num_topics=num_topics, id2word=id2word
)
model_list.append(model)
coherencemodel = CoherenceModel(
model=model, texts=texts, dictionary=dictionary, coherence='c_v'
)
coherence_values.append(coherencemodel.get_coherence())
return model_list, coherence_values
ตอนนี้ด้วยความช่วยเหลือของโค้ดต่อไปนี้เราจะได้รับจำนวนหัวข้อที่เหมาะสมที่สุดซึ่งเราสามารถแสดงด้วยความช่วยเหลือของกราฟได้เช่นกัน -
model_list, coherence_values = coherence_values_computation (
dictionary=id2word, corpus=corpus, texts=data_lemmatized,
start=1, limit=50, step=8
)
limit=50; start=1; step=8;
x = range(start, limit, step)
plt.plot(x, coherence_values)
plt.xlabel("Num Topics")
plt.ylabel("Coherence score")
plt.legend(("coherence_values"), loc='best')
plt.show()
เอาต์พุต
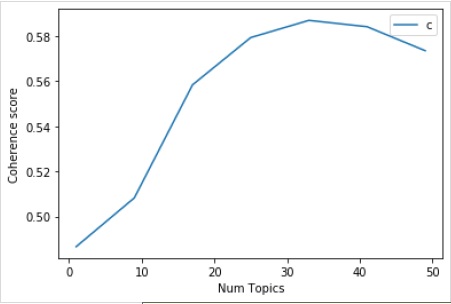
จากนั้นเรายังสามารถพิมพ์ค่าการเชื่อมโยงสำหรับหัวข้อต่างๆได้ดังนี้ -
for m, cv in zip(x, coherence_values):
print("Num Topics =", m, " is having Coherence Value of", round(cv, 4))
เอาต์พุต
Num Topics = 1 is having Coherence Value of 0.4866
Num Topics = 9 is having Coherence Value of 0.5083
Num Topics = 17 is having Coherence Value of 0.5584
Num Topics = 25 is having Coherence Value of 0.5793
Num Topics = 33 is having Coherence Value of 0.587
Num Topics = 41 is having Coherence Value of 0.5842
Num Topics = 49 is having Coherence Value of 0.5735
ตอนนี้เกิดคำถามว่าเราควรเลือกรุ่นไหนดี? แนวทางปฏิบัติที่ดีประการหนึ่งคือการเลือกแบบจำลองซึ่งให้ค่าการเชื่อมโยงกันสูงสุดก่อนที่จะประจบสอพลอ นั่นคือเหตุผลที่เราจะเลือกรุ่นที่มี 25 หัวข้อซึ่งอยู่ที่อันดับ 4 ในรายการด้านบน
optimal_model = model_list[3]
model_topics = optimal_model.show_topics(formatted=False)
pprint(optimal_model.print_topics(num_words=10))
[
(0,
'0.018*"power" + 0.011*"high" + 0.010*"ground" + 0.009*"current" + '
'0.008*"low" + 0.008*"wire" + 0.007*"water" + 0.007*"work" + 0.007*"design" '
'+ 0.007*"light"'),
(1,
'0.036*"game" + 0.029*"team" + 0.029*"year" + 0.028*"play" + 0.020*"player" '
'+ 0.019*"win" + 0.018*"good" + 0.013*"season" + 0.012*"run" + 0.011*"hit"'),
(2,
'0.020*"image" + 0.019*"information" + 0.017*"include" + 0.017*"mail" + '
'0.016*"send" + 0.015*"list" + 0.013*"post" + 0.012*"address" + '
'0.012*"internet" + 0.012*"system"'),
(3,
'0.986*"ax" + 0.002*"_" + 0.001*"tm" + 0.000*"part" + 0.000*"biz" + '
'0.000*"mb" + 0.000*"mbs" + 0.000*"pne" + 0.000*"end" + 0.000*"di"'),
(4,
'0.020*"make" + 0.014*"work" + 0.013*"money" + 0.013*"year" + 0.012*"people" '
'+ 0.011*"job" + 0.010*"group" + 0.009*"government" + 0.008*"support" + '
'0.008*"question"'),
(5,
'0.011*"study" + 0.011*"drug" + 0.009*"science" + 0.008*"food" + '
'0.008*"problem" + 0.008*"result" + 0.008*"effect" + 0.007*"doctor" + '
'0.007*"research" + 0.007*"patient"'),
(6,
'0.024*"gun" + 0.024*"law" + 0.019*"state" + 0.015*"case" + 0.013*"people" + '
'0.010*"crime" + 0.010*"weapon" + 0.010*"person" + 0.008*"firearm" + '
'0.008*"police"'),
(7,
'0.012*"word" + 0.011*"question" + 0.011*"exist" + 0.011*"true" + '
'0.010*"religion" + 0.010*"claim" + 0.008*"argument" + 0.008*"truth" + '
'0.008*"life" + 0.008*"faith"'),
(8,
'0.077*"time" + 0.029*"day" + 0.029*"call" + 0.025*"back" + 0.021*"work" + '
'0.019*"long" + 0.015*"end" + 0.015*"give" + 0.014*"year" + 0.014*"week"'),
(9,
'0.048*"thing" + 0.041*"make" + 0.038*"good" + 0.037*"people" + '
'0.028*"write" + 0.019*"bad" + 0.019*"point" + 0.018*"read" + 0.018*"post" + '
'0.016*"idea"'),
(10,
'0.022*"book" + 0.020*"_" + 0.013*"man" + 0.012*"people" + 0.011*"write" + '
'0.011*"find" + 0.010*"history" + 0.010*"armenian" + 0.009*"turkish" + '
'0.009*"number"'),
(11,
'0.064*"line" + 0.030*"buy" + 0.028*"organization" + 0.025*"price" + '
'0.025*"sell" + 0.023*"good" + 0.021*"host" + 0.018*"sale" + 0.017*"mail" + '
'0.016*"cost"'),
(12,
'0.041*"car" + 0.015*"bike" + 0.011*"ride" + 0.010*"engine" + 0.009*"drive" '
'+ 0.008*"side" + 0.008*"article" + 0.007*"turn" + 0.007*"front" + '
'0.007*"speed"'),
(13,
'0.018*"people" + 0.011*"attack" + 0.011*"state" + 0.011*"israeli" + '
'0.010*"war" + 0.010*"country" + 0.010*"government" + 0.009*"live" + '
'0.009*"give" + 0.009*"land"'),
(14,
'0.037*"file" + 0.026*"line" + 0.021*"read" + 0.019*"follow" + '
'0.018*"number" + 0.015*"program" + 0.014*"write" + 0.012*"entry" + '
'0.012*"give" + 0.011*"check"'),
(15,
'0.196*"write" + 0.172*"line" + 0.165*"article" + 0.117*"organization" + '
'0.086*"host" + 0.030*"reply" + 0.010*"university" + 0.008*"hear" + '
'0.007*"post" + 0.007*"news"'),
(16,
'0.021*"people" + 0.014*"happen" + 0.014*"child" + 0.012*"kill" + '
'0.011*"start" + 0.011*"live" + 0.010*"fire" + 0.010*"leave" + 0.009*"hear" '
'+ 0.009*"home"'),
(17,
'0.038*"key" + 0.018*"system" + 0.015*"space" + 0.015*"technology" + '
'0.014*"encryption" + 0.010*"chip" + 0.010*"bit" + 0.009*"launch" + '
'0.009*"public" + 0.009*"government"'),
(18,
'0.035*"drive" + 0.031*"system" + 0.027*"problem" + 0.027*"card" + '
'0.020*"driver" + 0.017*"bit" + 0.017*"work" + 0.016*"disk" + '
'0.014*"monitor" + 0.014*"machine"'),
(19,
'0.031*"window" + 0.020*"run" + 0.018*"color" + 0.018*"program" + '
'0.017*"application" + 0.016*"display" + 0.015*"set" + 0.015*"version" + '
'0.012*"screen" + 0.012*"problem"')
]
การค้นหาหัวข้อที่โดดเด่นในประโยค
การค้นหาหัวข้อที่โดดเด่นในประโยคเป็นหนึ่งในการประยุกต์ใช้การสร้างแบบจำลองหัวข้อที่เป็นประโยชน์มากที่สุด เป็นตัวกำหนดว่าเอกสารนั้นเกี่ยวกับหัวข้อใด ที่นี่เราจะพบหมายเลขหัวข้อที่มีเปอร์เซ็นต์การสนับสนุนสูงสุดในเอกสารนั้น ๆ ในการรวบรวมข้อมูลในตารางเราจะสร้างฟังก์ชันชื่อdominant_topics() -
def dominant_topics(ldamodel=lda_model, corpus=corpus, texts=data):
sent_topics_df = pd.DataFrame()
ต่อไปเราจะได้รับหัวข้อหลักในทุกเอกสาร -
for i, row in enumerate(ldamodel[corpus]):
row = sorted(row, key=lambda x: (x[1]), reverse=True)
ต่อไปเราจะได้รับหัวข้อ Dominant, Perc Contribution และ Keywords สำหรับทุกเอกสาร -
for j, (topic_num, prop_topic) in enumerate(row):
if j == 0: # => dominant topic
wp = ldamodel.show_topic(topic_num)
topic_keywords = ", ".join([word for word, prop in wp])
sent_topics_df = sent_topics_df.append(
pd.Series([int(topic_num), round(prop_topic,4), topic_keywords]), ignore_index=True
)
else:
break
sent_topics_df.columns = ['Dominant_Topic', 'Perc_Contribution', 'Topic_Keywords']
ด้วยความช่วยเหลือของรหัสต่อไปนี้เราจะเพิ่มข้อความต้นฉบับที่ส่วนท้ายของผลลัพธ์ -
contents = pd.Series(texts)
sent_topics_df = pd.concat([sent_topics_df, contents], axis=1)
return(sent_topics_df)
df_topic_sents_keywords = dominant_topics(
ldamodel=optimal_model, corpus=corpus, texts=data
)
ตอนนี้ทำการจัดรูปแบบของหัวข้อในประโยคดังนี้ -
df_dominant_topic = df_topic_sents_keywords.reset_index()
df_dominant_topic.columns = [
'Document_No', 'Dominant_Topic', 'Topic_Perc_Contrib', 'Keywords', 'Text'
]
สุดท้ายเราสามารถแสดงหัวข้อที่โดดเด่นได้ดังนี้ -
df_dominant_topic.head(15)
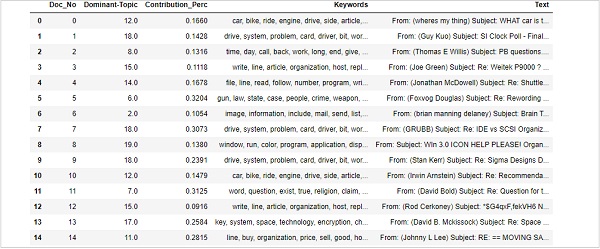
การค้นหาเอกสารที่เป็นตัวแทนส่วนใหญ่
เพื่อทำความเข้าใจเพิ่มเติมเกี่ยวกับหัวข้อนี้เรายังสามารถค้นหาเอกสารได้หัวข้อหนึ่ง ๆ มีส่วนทำให้เกิดมากที่สุด เราสามารถสรุปหัวข้อนั้นได้โดยการอ่านเอกสารนั้น ๆ
sent_topics_sorteddf_mallet = pd.DataFrame()
sent_topics_outdf_grpd = df_topic_sents_keywords.groupby('Dominant_Topic')
for i, grp in sent_topics_outdf_grpd:
sent_topics_sorteddf_mallet = pd.concat([sent_topics_sorteddf_mallet,
grp.sort_values(['Perc_Contribution'], ascending=[0]).head(1)], axis=0)
sent_topics_sorteddf_mallet.reset_index(drop=True, inplace=True)
sent_topics_sorteddf_mallet.columns = [
'Topic_Number', "Contribution_Perc", "Keywords", "Text"
]
sent_topics_sorteddf_mallet.head()
เอาต์พุต
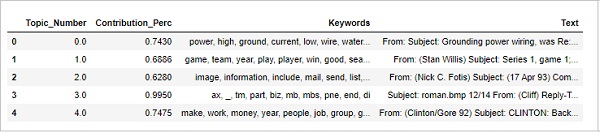
ปริมาณและการกระจายหัวข้อ
บางครั้งเรายังต้องการตัดสินว่ามีการพูดถึงหัวข้อในเอกสารอย่างกว้างขวางเพียงใด สำหรับสิ่งนี้เราจำเป็นต้องเข้าใจปริมาณและการกระจายของหัวข้อในเอกสาร
ขั้นแรกให้คำนวณจำนวนเอกสารสำหรับทุกหัวข้อดังนี้ -
topic_counts = df_topic_sents_keywords['Dominant_Topic'].value_counts()
จากนั้นคำนวณเปอร์เซ็นต์ของเอกสารสำหรับทุกหัวข้อดังนี้ -;
topic_contribution = round(topic_counts/topic_counts.sum(), 4)
ตอนนี้ค้นหาหัวข้อ Number และ Keywords ดังนี้ -
topic_num_keywords = df_topic_sents_keywords[['Dominant_Topic', 'Topic_Keywords']]
ตอนนี้เชื่อมต่อคอลัมน์ที่ชาญฉลาดดังนี้ -
df_dominant_topics = pd.concat(
[topic_num_keywords, topic_counts, topic_contribution], axis=1
)
ต่อไปเราจะเปลี่ยนชื่อคอลัมน์ดังนี้ -
df_dominant_topics.columns = [
'Dominant-Topic', 'Topic-Keywords', 'Num_Documents', 'Perc_Documents'
]
df_dominant_topics
เอาต์พุต
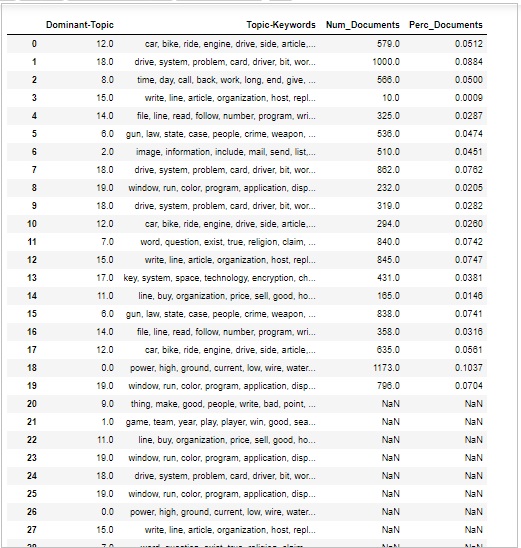