Seaborn-커널 밀도 추정
커널 밀도 추정 (KDE)은 연속 랜덤 변수의 확률 밀도 함수를 추정하는 방법입니다. 비모수 분석에 사용됩니다.
설정 hist False로 플래그 지정 distplot 커널 밀도 추정 플롯을 생성합니다.
예
import pandas as pd
import seaborn as sb
from matplotlib import pyplot as plt
df = sb.load_dataset('iris')
sb.distplot(df['petal_length'],hist=False)
plt.show()
산출
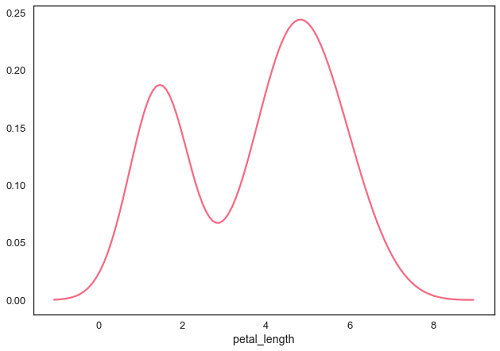
피팅 모수 분포
distplot() 데이터 세트의 매개 변수 분포를 시각화하는 데 사용됩니다.
예
import pandas as pd
import seaborn as sb
from matplotlib import pyplot as plt
df = sb.load_dataset('iris')
sb.distplot(df['petal_length'])
plt.show()
산출

이변 량 분포 플로팅
이변 량 분포는 두 변수 간의 관계를 결정하는 데 사용됩니다. 이것은 주로 두 변수 간의 관계와 한 변수가 다른 변수에 대해 어떻게 작동 하는지를 다룹니다.
seaborn에서 이변 량 분포를 분석하는 가장 좋은 방법은 jointplot() 함수.
Jointplot은 두 변수 간의 이변 량 관계와 개별 축에있는 각 변수의 일 변량 분포를 투영하는 다중 패널 그림을 생성합니다.
산포도
산점도는 각 관측 값이 x 및 y 축을 통해 2 차원 플롯으로 표현되는 분포를 시각화하는 가장 편리한 방법입니다.
예
import pandas as pd
import seaborn as sb
from matplotlib import pyplot as plt
df = sb.load_dataset('iris')
sb.jointplot(x = 'petal_length',y = 'petal_width',data = df)
plt.show()
산출

위 그림은 petal_length 과 petal_widthIris 데이터에서. 플롯의 추세에 따르면 연구중인 변수간에 양의 상관 관계가 있습니다.
Hexbin 플롯
Hexagonal Binning은 데이터 밀도가 희박 할 때, 즉 데이터가 매우 흩어져 산점도를 통해 분석하기 어려운 경우 이변 량 데이터 분석에 사용됩니다.
'kind'및 값 'hex'라는 추가 매개 변수는 hexbin 플롯을 플로팅합니다.
예
import pandas as pd
import seaborn as sb
from matplotlib import pyplot as plt
df = sb.load_dataset('iris')
sb.jointplot(x = 'petal_length',y = 'petal_width',data = df,kind = 'hex')
plt.show()

커널 밀도 추정
커널 밀도 추정은 변수의 분포를 추정하는 비모수 적 방법입니다. seaborn에서는 다음을 사용하여 kde를 플로팅 할 수 있습니다.jointplot().
커널 플롯을 플로팅하려면 매개 변수 종류에 'kde'값을 전달합니다.
예
import pandas as pd
import seaborn as sb
from matplotlib import pyplot as plt
df = sb.load_dataset('iris')
sb.jointplot(x = 'petal_length',y = 'petal_width',data = df,kind = 'hex')
plt.show()
산출
