Gensim-LDA 토픽 모델 사용
이 장에서는 LDA (Latent Dirichlet Allocation) 주제 모델을 사용하는 방법을 이해합니다.
LDA 모델에서 주제보기
위에서 만든 LDA 모델 (lda_model)을 사용하여 문서의 주제를 볼 수 있습니다. 다음 스크립트의 도움으로 할 수 있습니다-
pprint(lda_model.print_topics())
doc_lda = lda_model[corpus]
산출
[
(0,
'0.036*"go" + 0.027*"get" + 0.021*"time" + 0.017*"back" + 0.015*"good" + '
'0.014*"much" + 0.014*"be" + 0.013*"car" + 0.013*"well" + 0.013*"year"'),
(1,
'0.078*"screen" + 0.067*"video" + 0.052*"character" + 0.046*"normal" + '
'0.045*"mouse" + 0.034*"manager" + 0.034*"disease" + 0.031*"processor" + '
'0.028*"excuse" + 0.028*"choice"'),
(2,
'0.776*"ax" + 0.079*"_" + 0.011*"boy" + 0.008*"ticket" + 0.006*"red" + '
'0.004*"conservative" + 0.004*"cult" + 0.004*"amazing" + 0.003*"runner" + '
'0.003*"roughly"'),
(3,
'0.086*"season" + 0.078*"fan" + 0.072*"reality" + 0.065*"trade" + '
'0.045*"concept" + 0.040*"pen" + 0.028*"blow" + 0.025*"improve" + '
'0.025*"cap" + 0.021*"penguin"'),
(4,
'0.027*"group" + 0.023*"issue" + 0.016*"case" + 0.016*"cause" + '
'0.014*"state" + 0.012*"whole" + 0.012*"support" + 0.011*"government" + '
'0.010*"year" + 0.010*"rate"'),
(5,
'0.133*"evidence" + 0.047*"believe" + 0.044*"religion" + 0.042*"belief" + '
'0.041*"sense" + 0.041*"discussion" + 0.034*"atheist" + 0.030*"conclusion" +
'
'0.029*"explain" + 0.029*"claim"'),
(6,
'0.083*"space" + 0.059*"science" + 0.031*"launch" + 0.030*"earth" + '
'0.026*"route" + 0.024*"orbit" + 0.024*"scientific" + 0.021*"mission" + '
'0.018*"plane" + 0.017*"satellite"'),
(7,
'0.065*"file" + 0.064*"program" + 0.048*"card" + 0.041*"window" + '
'0.038*"driver" + 0.037*"software" + 0.034*"run" + 0.029*"machine" + '
'0.029*"entry" + 0.028*"version"'),
(8,
'0.078*"publish" + 0.059*"mount" + 0.050*"turkish" + 0.043*"armenian" + '
'0.027*"western" + 0.026*"russian" + 0.025*"locate" + 0.024*"proceed" + '
'0.024*"electrical" + 0.022*"terrorism"'),
(9,
'0.023*"people" + 0.023*"child" + 0.021*"kill" + 0.020*"man" + 0.019*"death" '
'+ 0.015*"die" + 0.015*"live" + 0.014*"attack" + 0.013*"age" + '
'0.011*"church"'),
(10,
'0.092*"cpu" + 0.085*"black" + 0.071*"controller" + 0.039*"white" + '
'0.028*"water" + 0.027*"cold" + 0.025*"solid" + 0.024*"cool" + 0.024*"heat" '
'+ 0.023*"nuclear"'),
(11,
'0.071*"monitor" + 0.044*"box" + 0.042*"option" + 0.041*"generate" + '
'0.038*"vote" + 0.032*"battery" + 0.029*"wave" + 0.026*"tradition" + '
'0.026*"fairly" + 0.025*"task"'),
(12,
'0.048*"send" + 0.045*"mail" + 0.036*"list" + 0.033*"include" + '
'0.032*"price" + 0.031*"address" + 0.027*"email" + 0.026*"receive" + '
'0.024*"book" + 0.024*"sell"'),
(13,
'0.515*"drive" + 0.052*"laboratory" + 0.042*"blind" + 0.020*"investment" + '
'0.011*"creature" + 0.010*"loop" + 0.005*"dialog" + 0.000*"slave" + '
'0.000*"jumper" + 0.000*"sector"'),
(14,
'0.153*"patient" + 0.066*"treatment" + 0.062*"printer" + 0.059*"doctor" + '
'0.036*"medical" + 0.031*"energy" + 0.029*"study" + 0.029*"probe" + '
'0.024*"mph" + 0.020*"physician"'),
(15,
'0.068*"law" + 0.055*"gun" + 0.039*"government" + 0.036*"right" + '
'0.029*"state" + 0.026*"drug" + 0.022*"crime" + 0.019*"person" + '
'0.019*"citizen" + 0.019*"weapon"'),
(16,
'0.107*"team" + 0.102*"game" + 0.078*"play" + 0.055*"win" + 0.052*"player" + '
'0.051*"year" + 0.030*"score" + 0.025*"goal" + 0.023*"wing" + 0.023*"run"'),
(17,
'0.031*"say" + 0.026*"think" + 0.022*"people" + 0.020*"make" + 0.017*"see" + '
'0.016*"know" + 0.013*"come" + 0.013*"even" + 0.013*"thing" + 0.013*"give"'),
(18,
'0.039*"system" + 0.034*"use" + 0.023*"key" + 0.016*"bit" + 0.016*"also" + '
'0.015*"information" + 0.014*"source" + 0.013*"chip" + 0.013*"available" + '
'0.010*"provide"'),
(19,
'0.085*"line" + 0.073*"write" + 0.053*"article" + 0.046*"organization" + '
'0.034*"host" + 0.023*"be" + 0.023*"know" + 0.017*"thank" + 0.016*"want" + '
'0.014*"help"')
]
컴퓨팅 모델 난이도
위에서 만든 LDA 모델 (lda_model)은 모델의 난이도, 즉 모델이 얼마나 좋은지를 계산하는 데 사용할 수 있습니다. 점수가 낮을수록 모델이 더 좋아집니다. 다음 스크립트의 도움으로 할 수 있습니다-
print('\nPerplexity: ', lda_model.log_perplexity(corpus))
산출
Perplexity: -12.338664984332151
일관성 점수 계산
LDA 모델 (lda_model)위에서 생성 한 것은 모델의 일관성 점수, 즉 주제에있는 단어의 쌍별 단어 유사성 점수의 평균 / 중앙값을 계산하는 데 사용할 수 있습니다. 다음 스크립트의 도움으로 할 수 있습니다-
coherence_model_lda = CoherenceModel(
model=lda_model, texts=data_lemmatized, dictionary=id2word, coherence='c_v'
)
coherence_lda = coherence_model_lda.get_coherence()
print('\nCoherence Score: ', coherence_lda)
산출
Coherence Score: 0.510264381411751
주제-키워드 시각화
LDA 모델 (lda_model)위에서 만든 내용을 사용하여 생성 된 주제 및 관련 키워드를 검토 할 수 있습니다. 다음을 사용하여 시각화 할 수 있습니다.pyLDAvis다음과 같이 패키지-
pyLDAvis.enable_notebook()
vis = pyLDAvis.gensim.prepare(lda_model, corpus, id2word)
vis
산출
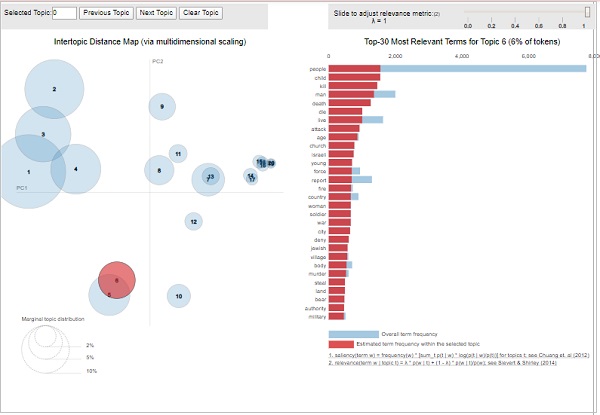
위의 출력에서 왼쪽의 거품은 주제를 나타내고 거품이 클수록 해당 주제가 더 널리 퍼집니다. 주제 모델에 차트 전체에 겹치지 않는 큰 거품이 흩어져 있으면 주제 모델이 좋습니다.